Google researchers have unveiled TransformerFAM, a novel architecture set to revolutionize long-context processing in large language models (LLMs). By integrating a feedback loop mechanism, TransformerFAM promises to enhance the network’s ability to handle infinitely long sequences. This addresses the limitations posed by quadratic attention complexity.
また読む: PyTorch’s TorchTune: Revolutionizing LLM Fine-Tuning
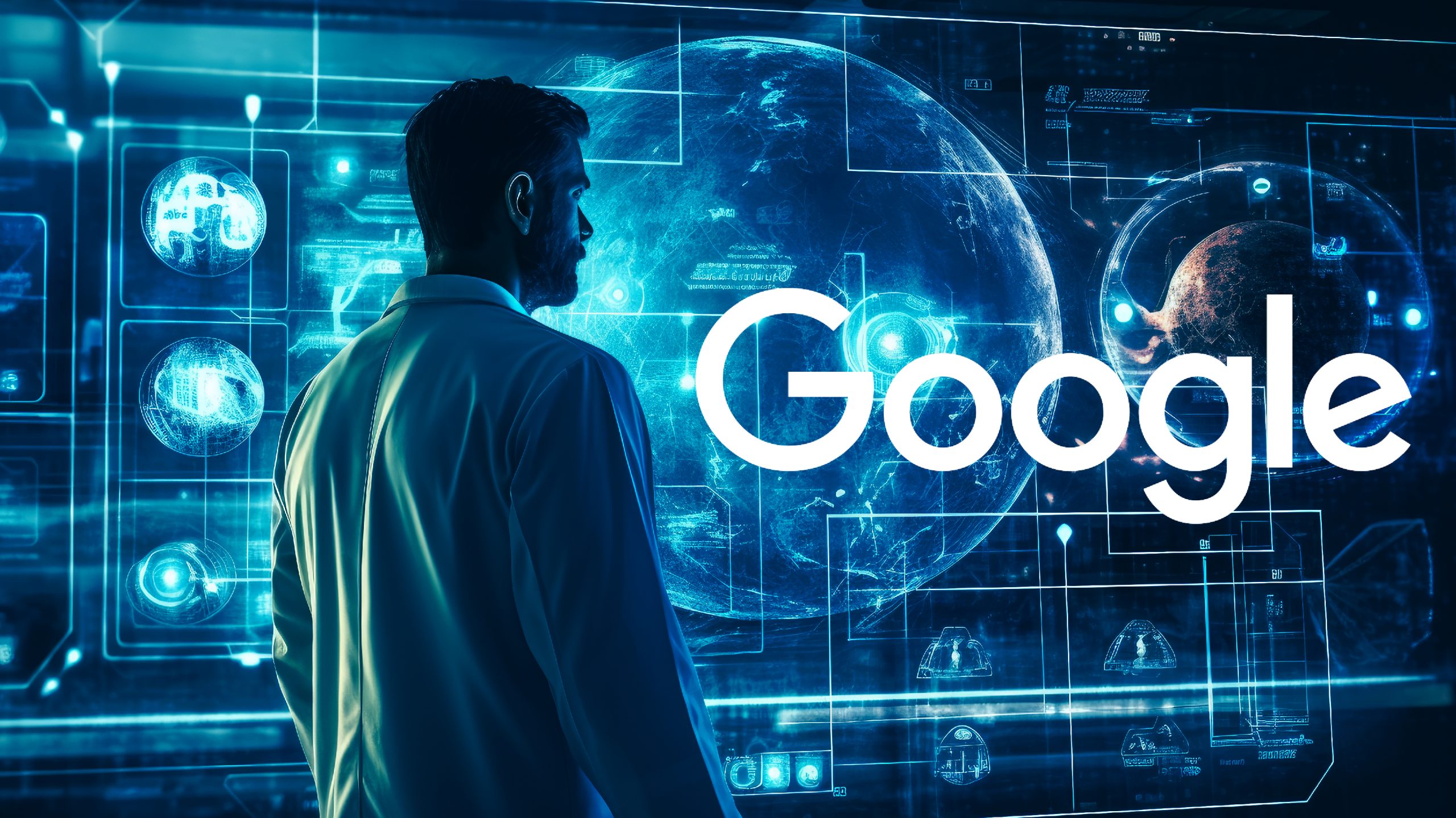
制限を理解する
Traditional attention mechanisms in トランスフォーマー exhibit quadratic complexity concerning context length, constraining their efficacy in processing long sequences. While attempts like sliding window attention and sparse or linear approximations have been made, they often fall short, especially at larger scales.
The Solution: TransformerFAM
In response to these challenges, Google’s TransformerFAM introduces a feedback 注意メカニズム, inspired by the concept of working memory in the human brain. This mechanism allows the model to attend to its own latent representations, fostering the emergence of working memory within the Transformer architecture.
また読む: Microsoft Introduces AllHands: LLM Framework for Large-Scale Feedback Analysis
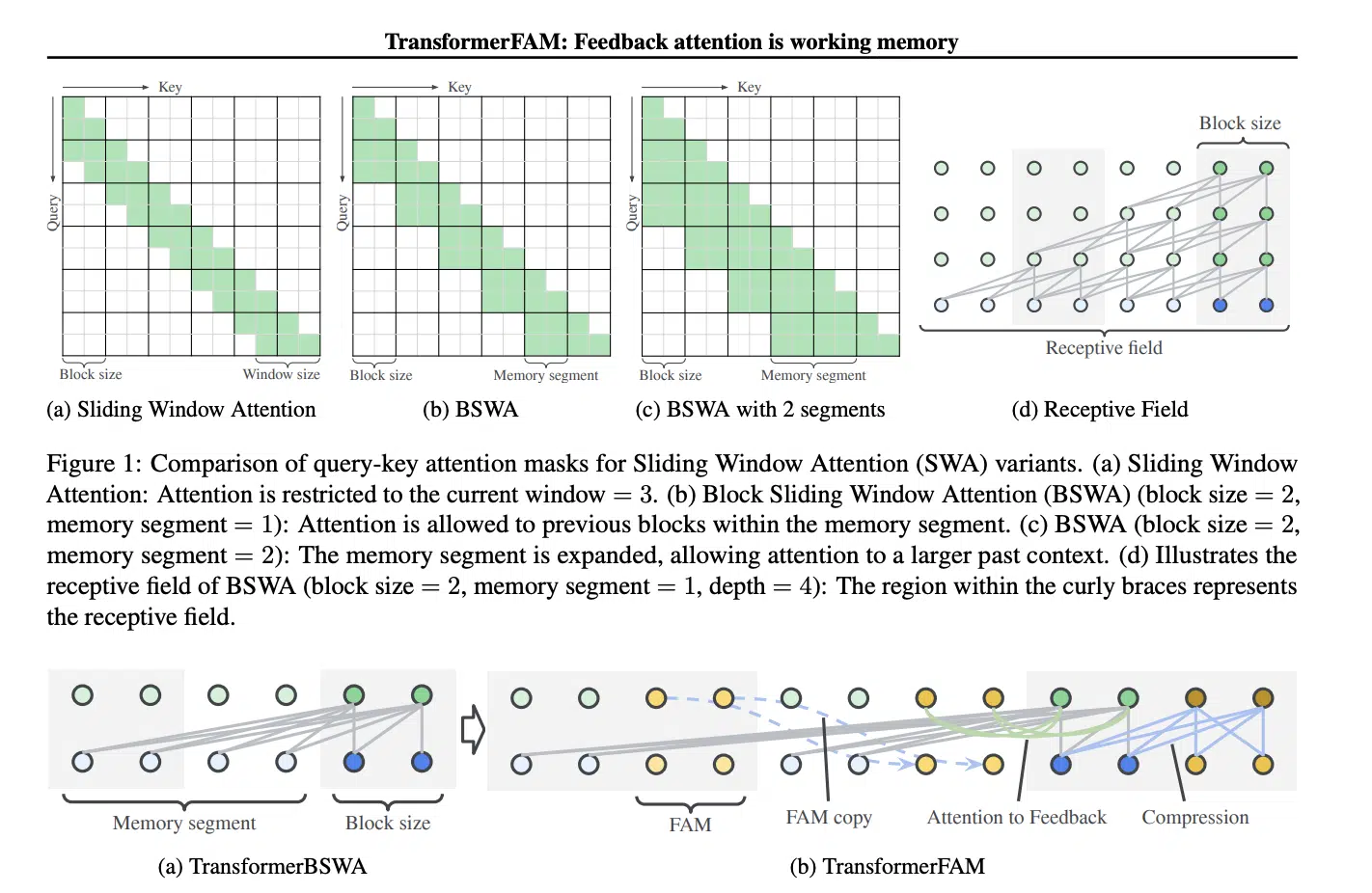
主な機能と革新
TransformerFAM incorporates a Block Sliding Window Attention (BSWA) module, enabling efficient attention to both local and long-range dependencies within input and output sequences. By integrating feedback activations into each block, the architecture facilitates the dynamic propagation of global contextual information across blocks.
Performance and Potential
Experimental results across various model sizes demonstrate significant improvements in long-context tasks, surpassing other configurations. TransformerFAM’s seamless integration with pre-trained models and minimal impact on training efficiency make it a promising solution for empowering LLMs to process sequences of unlimited length.
また読む: Databricks DBRX: 巨人に対抗するオープンソース LLM
私たちの言う
TransformerFAM marks a significant advancement in the field of 深い学習. It offers a promising solution to the long-standing challenge of processing infinitely long sequences. By leveraging feedback attention and Block Sliding Window Attention, Google has paved the way for more efficient and effective long-context processing in LLMs. This has far-reaching implications for natural language understanding and reasoning tasks.
フォローをお願いします グーグルニュース AI、データサイエンス、その他の世界の最新のイノベーションを常に最新の状態に保つため ゲンアイ.
- SEO を活用したコンテンツと PR 配信。 今日増幅されます。
- PlatoData.Network 垂直生成 Ai。 自分自身に力を与えましょう。 こちらからアクセスしてください。
- プラトアイストリーム。 Web3 インテリジェンス。 知識増幅。 こちらからアクセスしてください。
- プラトンESG。 カーボン、 クリーンテック、 エネルギー、 環境、 太陽、 廃棄物管理。 こちらからアクセスしてください。
- プラトンヘルス。 バイオテクノロジーと臨床試験のインテリジェンス。 こちらからアクセスしてください。
- 情報源: https://www.analyticsvidhya.com/blog/2024/04/googles-transformerfam-a-breakthrough-in-long-context-processing/
- :持っている
- a
- 能力
- 越えて
- アクティベーション
- アドレス
- アドバンス
- AI
- ことができます
- および
- 建築
- At
- 試み
- 出席する
- 注意
- き
- ブロック
- ブロック
- 両言語で
- 脳
- 画期的な
- by
- 挑戦する
- 課題
- 複雑さ
- コンセプト
- について
- 構成
- コンテキスト
- 文脈上の
- データ
- データサイエンス
- 実証します
- 依存関係
- ダイナミック
- 各
- 効果的な
- 効能
- 効率
- 効率的な
- 出現
- エンパワーメント
- 有効にする
- 高めます
- 特に
- 展示
- 促進する
- 秋
- 広範囲に及ぶ
- 特徴
- フィードバック
- フィールド
- 助長
- フレームワーク
- グローバル
- でログイン
- Googleの
- ハンドル
- 持ってる
- ハイ
- HTTPS
- 人間
- 影響
- 意義
- 改善
- in
- 組み込む
- 無限に
- 情報
- イノベーション
- インスピレーションある
- 統合
- 統合
- に
- 紹介します
- IT
- ITS
- JPG
- 言語
- 大
- 大規模
- より大きい
- 最新の
- 長さ
- 活用
- ような
- 制限
- 線形
- LLM
- ローカル
- 長い
- 長年の
- 製
- make
- メカニズム
- メカニズム
- メモリ
- 最小限の
- モデル
- モジュール
- 他には?
- もっと効率的
- ナチュラル
- 自然言語
- 自然言語理解
- 小説
- of
- オファー
- 頻繁に
- on
- オープンソース
- or
- その他
- 出力
- 自分の
- 舗装された
- プラトン
- プラトンデータインテリジェンス
- プラトデータ
- 提起
- プロセス
- 処理
- 約束
- 有望
- 伝播
- 二次
- 読む
- 推論
- 表現
- 研究者
- 応答
- 結果
- 革命を起こす
- 革命
- s
- 秤
- 科学
- シームレス
- セッションに
- ショート
- 重要
- サイズ
- スライディング
- 溶液
- まばらな
- 滞在
- 凌駕する
- 取得
- タスク
- 世界
- アプリ環境に合わせて
- ボーマン
- 彼ら
- この
- 〜へ
- トレーニング
- トランス
- 理解する
- 無限の
- 発表
- 更新しました
- us
- さまざまな
- 仕方..
- while
- ウィンドウを使用して入力ファイルを追加します。
- 以内
- ワーキング
- 世界
- ゼファーネット