Artificial intelligence (AI) can significantly improve loan decisioning accuracy, but several factors must be considered to maximize that effectiveness. Luckily, given some care and consideration, Zest AI’s chief legal officer Teddy Flo said they can be easily applied.
Flo’s career has focused on policy, legal and compliance issues. A consumer finance lawyer for much of his career, Flo worked for Freddie Mac once the housing crisis set in in 2008.
Zest AI’s thesis is that the older ways of evaluating credit are becoming even less effective as the economy becomes more complex. Those outdated methods introduce biases that are unacceptable today, given the technology that is available to mitigate those factors and their effect on women and people of color.
The importance of locked AI models
Flo said care must be taken before using AI. Lenders must use a locked AI form that cannot be changed as it absorbs new information. That means no generative or dynamic AI.
Locked AI systems are updated under very controlled conditions. As they are created, they are fed specific data sets, and their predictions are analyzed for fairness.
After a time, or as market conditions change, the models are paused before new data is added. The output is again tested to ensure the model is behaving fairly.
“You’re able to continually update the model to take in new data, but you do it in a controlled way,” Flo explained. “And you test it before you begin using it to decide to make decisions for actual consumers.”
Clear reasons needed when AI rejects loan applications
According to the Equal Opportunity Act, AI models must provide specific reasons for rejecting applications based on an advanced algorithm. On Sept. 19, 2023, the Consumer Financial Protection Bureau (CFPB) issued guidance on specific legal requirements lenders must adhere to when using AI and other complex models.
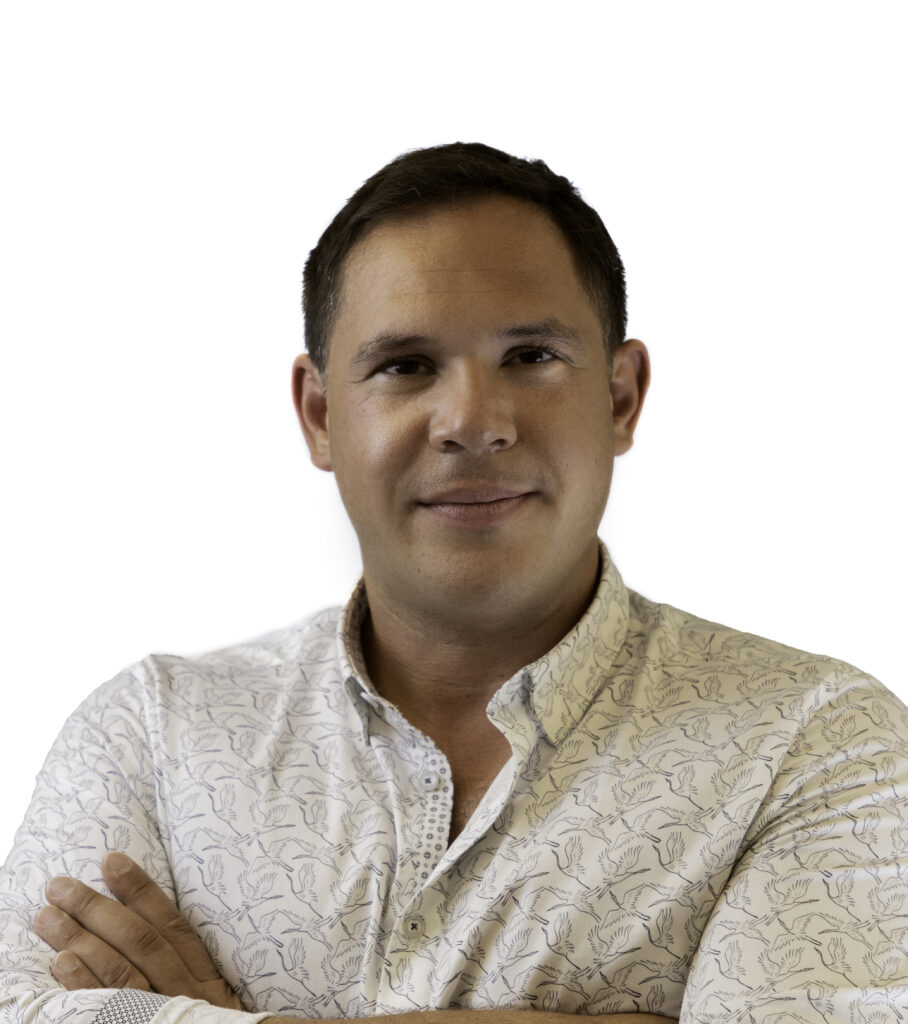
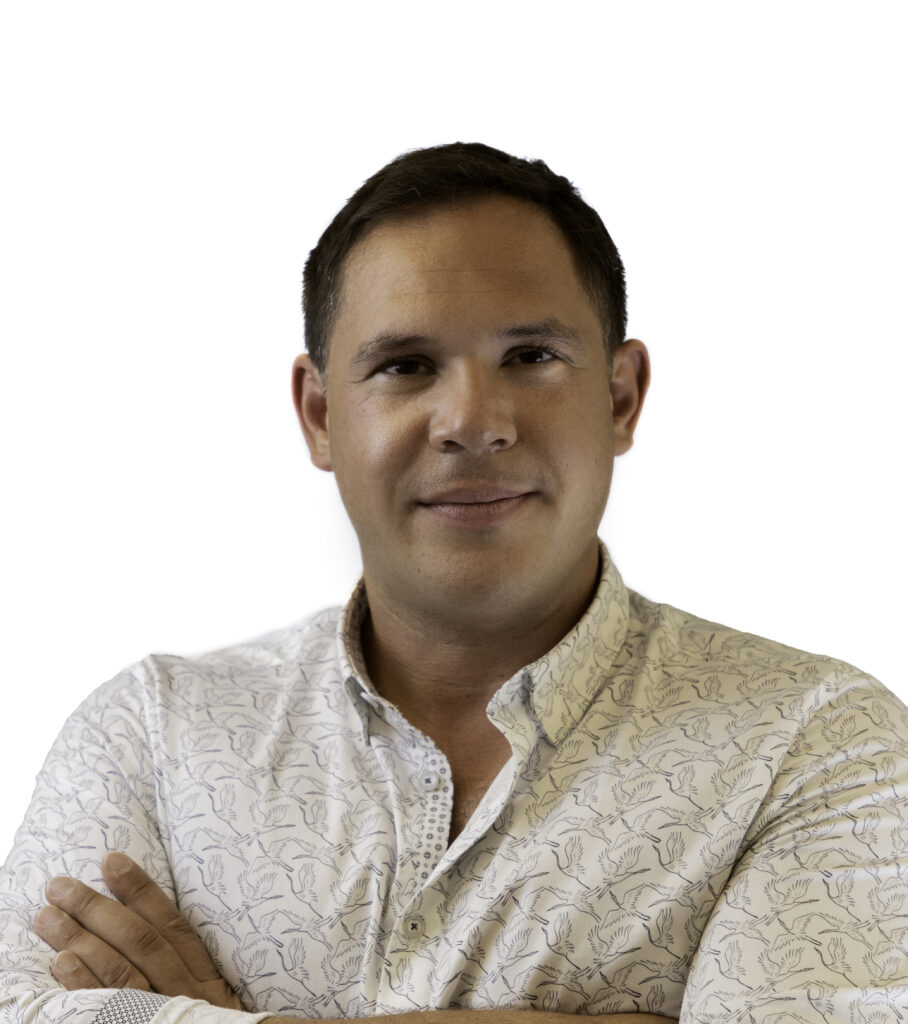
Creditors cannot use CFPB sample adverse action forms and checklists if they do not reflect the reason for denying the loan application. Those lists are not exhaustive or automatically cover a creditor’s legal requirements.
“Technology marketed as artificial intelligence is expanding the data used for lending decisions and growing the list of potential reasons why credit is denied,” said CFPB director Rohit Chopra. “Creditors must be able to specifically explain their reasons for denial. There is no special exemption for artificial intelligence.”
The CFPB said many algorithms are fed with data sets that can include data that could be harvested from consumer surveillance. That could lead to application rejections for reasons the consumer may not consider relevant to their finances.
“Creditors that simply select the closest factors from the checklist of sample reasons are not in compliance with the law if those reasons do not sufficiently reflect the actual reason for the action taken,” the circular states. “Creditors must disclose the specific reasons, even if consumers may be surprised, upset, or angered to learn their credit applications were being graded on data that may not intuitively relate to their finances.”
Removing bias from AI-based lending decisions
Given the wide racial gaps in common credit measures, regulators are right to be worried about how models might impact lending decisions. According to the Urban Institute, more than half of white households have a FICO score above 700. Only 20.6% of Black households do. One in three Black households with credit histories have insufficient credit and lack a credit score, nearly double the 17.9% rate for whites. Similar disparities exist between whites and Hispanics.
This shouldn’t be happening in a society with the means to erase those faulty rationales. AI can help render them obsolete.
“There may be some difference in credit quality because of the historical racism within America, but it’s not that extreme,” Flo said. “That is an overstated difference.
“What we wanted to do as a company is try to close the gap in a way that is just as accurate at predicting credit risk but treats different groups of people much more fairly and much more similarly. And we’ve been able to do that on average as a company for our financial institution clients.”
Using explainable AI
Given the technology available, there is no reason to rely on standard codes and checklists. Flo said Zest AI uses Shapely-based explainability methods. Shapely values originate in game theory and are based on the fair distribution of gains and costs to several actors working in a coalition. They are often applied when contributions are unequal.
“There are players in the market today that will use an AI model to make a decision, but then take reason codes from a credit report and give back to the consumer even though that’s not what the AI model was basing its decision on,” Flo said. “We think that’s wrong; we think that’s harming consumers. And we applaud the CFPB’s efforts to stop it.”
Bias removal efforts are in the early innings
Zest AI published a whitepaper on how to maintain guidance by CFPB regulations. Flo said the agency’s concerns of algorithmic bias are well-founded, given the number of examples of AI models being trained inappropriately.
With AI in its early stages, it’s only natural that efforts to eliminate bias are just as nascent. Flo said Zest AI developed and has updated a patented technique to de-bias credit models. It is an excellent early effort in a movement with a long way to go.
“It’s not always possible to close the gap completely with every underwriting model we built,” Flo admitted. “You could imagine a financial institution situated in a city, for example, with a very affluent white population and a Black population that is not as affluent.
“A simple underwriting model can’t solve the income inequality problem in America. But what it can do is make sure that folks who have comparable incomes or credit histories are approved at similar rates, regardless of their backgrounds, and that is what we do in spades.”
Don’t over-regulate AI
Flo is bullish on AI’s potential to help people who deserve credit get it when they would not have under old measures. That will change lives for the better.
Throwing that all away over addressable regulatory concerns would be a travesty. People are being helped in tangible ways that keep them out of high-interest debt.
“They’re getting their emergency bills paid,” Flo said. “They’re also not being pulled into a cycle of debt. There are so many levels of benefits that this technology creates that missing those benefits for addressable regulatory concerns would be a travesty for individuals and the economy.
“Bias is real in lending. But AI is the solution to reducing and eliminating that bias, not the problem because if it is built thoughtfully and intentionally, that’s something that we have access to.”
Also see:
.pp-multiple-authors-boxes-wrapper.box-post-id-45383.pp-multiple-authors-layout-boxed.multiple-authors-target-shortcode.box-instance-id-1 .box-header-title { font-size: 20px !important; } .pp-multiple-authors-boxes-wrapper.box-post-id-45383.pp-multiple-authors-layout-boxed.multiple-authors-target-shortcode.box-instance-id-1 .box-header-title { font-weight: bold !important; } .pp-multiple-authors-boxes-wrapper.box-post-id-45383.pp-multiple-authors-layout-boxed.multiple-authors-target-shortcode.box-instance-id-1 .box-header-title { color: #000000 !important; } .pp-multiple-authors-boxes-wrapper.box-post-id-45383.pp-multiple-authors-layout-boxed.multiple-authors-target-shortcode.box-instance-id-1 .pp-author-boxes-avatar img { border-style: none !important; } .pp-multiple-authors-boxes-wrapper.box-post-id-45383.pp-multiple-authors-layout-boxed.multiple-authors-target-shortcode.box-instance-id-1 .pp-author-boxes-avatar img { border-radius: 5% !important; } .pp-multiple-authors-boxes-wrapper.box-post-id-45383.pp-multiple-authors-layout-boxed.multiple-authors-target-shortcode.box-instance-id-1 .pp-author-boxes-name a { font-size: 24px !important; } .pp-multiple-authors-boxes-wrapper.box-post-id-45383.pp-multiple-authors-layout-boxed.multiple-authors-target-shortcode.box-instance-id-1 .pp-author-boxes-name a { font-weight: bold !important; } .pp-multiple-authors-boxes-wrapper.box-post-id-45383.pp-multiple-authors-layout-boxed.multiple-authors-target-shortcode.box-instance-id-1 .pp-author-boxes-name a { color: #000000 !important; } .pp-multiple-authors-boxes-wrapper.box-post-id-45383.pp-multiple-authors-layout-boxed.multiple-authors-target-shortcode.box-instance-id-1 .pp-author-boxes-description { font-style: none !important; } .pp-multiple-authors-boxes-wrapper.box-post-id-45383.pp-multiple-authors-layout-boxed.multiple-authors-target-shortcode.box-instance-id-1 .pp-author-boxes-description { text-align: left !important; } .pp-multiple-authors-boxes-wrapper.box-post-id-45383.pp-multiple-authors-layout-boxed.multiple-authors-target-shortcode.box-instance-id-1 .pp-author-boxes-meta a span { font-size: 20px !important; } .pp-multiple-authors-boxes-wrapper.box-post-id-45383.pp-multiple-authors-layout-boxed.multiple-authors-target-shortcode.box-instance-id-1 .pp-author-boxes-meta a span { font-weight: normal !important; } .pp-multiple-authors-boxes-wrapper.box-post-id-45383.pp-multiple-authors-layout-boxed.multiple-authors-target-shortcode.box-instance-id-1 .pp-author-boxes-meta { text-align: left !important; } .pp-multiple-authors-boxes-wrapper.box-post-id-45383.pp-multiple-authors-layout-boxed.multiple-authors-target-shortcode.box-instance-id-1 .pp-author-boxes-meta a { background-color: #6adc21 !important; } .pp-multiple-authors-boxes-wrapper.box-post-id-45383.pp-multiple-authors-layout-boxed.multiple-authors-target-shortcode.box-instance-id-1 .pp-author-boxes-meta a { color: #ffffff !important; } .pp-multiple-authors-boxes-wrapper.box-post-id-45383.pp-multiple-authors-layout-boxed.multiple-authors-target-shortcode.box-instance-id-1 .pp-author-boxes-meta a:hover { color: #ffffff !important; } .pp-multiple-authors-boxes-wrapper.box-post-id-45383.pp-multiple-authors-layout-boxed.multiple-authors-target-shortcode.box-instance-id-1 .ppma-author-user_url-profile-data { color: #6adc21 !important; } .pp-multiple-authors-boxes-wrapper.box-post-id-45383.pp-multiple-authors-layout-boxed.multiple-authors-target-shortcode.box-instance-id-1 .ppma-author-twitter-profile-data span, .pp-multiple-authors-boxes-wrapper.box-post-id-45383.pp-multiple-authors-layout-boxed.multiple-authors-target-shortcode.box-instance-id-1 .ppma-author-twitter-profile-data i { font-size: 16px !important; } .pp-multiple-authors-boxes-wrapper.box-post-id-45383.pp-multiple-authors-layout-boxed.multiple-authors-target-shortcode.box-instance-id-1 .ppma-author-twitter-profile-data { background-color: #6adc21 !important; } .pp-multiple-authors-boxes-wrapper.box-post-id-45383.pp-multiple-authors-layout-boxed.multiple-authors-target-shortcode.box-instance-id-1 .ppma-author-twitter-profile-data { border-radius: 50% !important; } .pp-multiple-authors-boxes-wrapper.box-post-id-45383.pp-multiple-authors-layout-boxed.multiple-authors-target-shortcode.box-instance-id-1 .ppma-author-twitter-profile-data { text-align: center !important; } .pp-multiple-authors-boxes-wrapper.box-post-id-45383.pp-multiple-authors-layout-boxed.multiple-authors-target-shortcode.box-instance-id-1 .ppma-author-linkedin-profile-data span, .pp-multiple-authors-boxes-wrapper.box-post-id-45383.pp-multiple-authors-layout-boxed.multiple-authors-target-shortcode.box-instance-id-1 .ppma-author-linkedin-profile-data i { font-size: 16px !important; } .pp-multiple-authors-boxes-wrapper.box-post-id-45383.pp-multiple-authors-layout-boxed.multiple-authors-target-shortcode.box-instance-id-1 .ppma-author-linkedin-profile-data { background-color: #6adc21 !important; } .pp-multiple-authors-boxes-wrapper.box-post-id-45383.pp-multiple-authors-layout-boxed.multiple-authors-target-shortcode.box-instance-id-1 .ppma-author-linkedin-profile-data { border-radius: 50% !important; } .pp-multiple-authors-boxes-wrapper.box-post-id-45383.pp-multiple-authors-layout-boxed.multiple-authors-target-shortcode.box-instance-id-1 .pp-author-boxes-recent-posts-title { border-bottom-style: dotted !important; } .pp-multiple-authors-boxes-wrapper.box-post-id-45383.pp-multiple-authors-layout-boxed.multiple-authors-target-shortcode.box-instance-id-1 .pp-multiple-authors-boxes-li { border-style: solid !important; } .pp-multiple-authors-boxes-wrapper.box-post-id-45383.pp-multiple-authors-layout-boxed.multiple-authors-target-shortcode.box-instance-id-1 .pp-multiple-authors-boxes-li { color: #3c434a !important; }
- SEO Powered Content & PR Distribution. Get Amplified Today.
- PlatoData.Network Vertical Generative Ai. Empower Yourself. Access Here.
- PlatoAiStream. Web3 Intelligence. Knowledge Amplified. Access Here.
- PlatoESG. Carbon, CleanTech, Energy, Environment, Solar, Waste Management. Access Here.
- PlatoHealth. Biotech and Clinical Trials Intelligence. Access Here.
- Source: https://www.fintechnexus.com/ai-if-used-properly-can-improve-loan-decisioning/
- :has
- :is
- :not
- 000
- 1
- 17
- 19
- 20
- 2008
- 2018
- 2023
- 700
- a
- Able
- About
- above
- absorbs
- access
- According
- accuracy
- accurate
- Act
- Action
- actors
- actual
- added
- addressable
- adhere
- admitted
- advanced
- adverse
- again
- AI
- AI models
- AI systems
- algorithm
- algorithmic
- algorithmic bias
- algorithms
- All
- also
- always
- america
- an
- analyzed
- and
- Application
- applications
- applied
- approved
- ARE
- articles
- artificial
- artificial intelligence
- Artificial intelligence (AI)
- AS
- At
- automatically
- available
- avatar
- average
- away
- back
- backgrounds
- based
- BE
- because
- becomes
- becoming
- been
- before
- begin
- being
- benefits
- Better
- between
- bias
- biases
- Bills
- Black
- blockchain
- bold
- built
- Bullish
- Bureau
- but
- by
- CAN
- cannot
- care
- Career
- Center
- CFPB
- change
- changed
- chief
- Chopra
- City
- clear
- clients
- Close
- closest
- coalition
- code
- Codes
- color
- COM
- Common
- company
- comparable
- completely
- complex
- compliance
- Concerns
- conditions
- Consider
- consideration
- considered
- consumer
- consumer finance
- Consumer Financial Protection Bureau
- Consumers
- continually
- contributions
- contributor
- controlled
- Costs
- could
- cover
- created
- creates
- credit
- crisis
- Crowdfunding
- cycle
- data
- data sets
- Debt
- decide
- decision
- decisions
- denied
- deserve
- developed
- difference
- different
- Director
- Disclose
- distribution
- do
- double
- dynamic
- Early
- easily
- economy
- effect
- Effective
- effectiveness
- effort
- efforts
- eliminate
- eliminating
- emergency
- emerging
- emerging technologies
- ensure
- equal
- Ether (ETH)
- evaluating
- Even
- Every
- example
- examples
- excellent
- exist
- expanding
- Explain
- Explainability
- explained
- extreme
- factors
- fair
- fairly
- fairness
- faulty
- Fed
- FICO
- finance
- Finances
- financial
- financial institution
- Financial Protection
- fintech
- FLO
- focused
- For
- form
- forms
- from
- Gains
- game
- gap
- gaps
- generative
- get
- getting
- Give
- given
- Go
- Group’s
- Growing
- guidance
- Half
- Happening
- harming
- Have
- he
- help
- helped
- his
- historical
- Hong
- Hong Kong
- hosted
- households
- housing
- hover
- How
- How To
- HTML
- HTTPS
- i
- if
- imagine
- Impact
- importance
- improve
- in
- include
- Income
- individuals
- Inequality
- information
- Institution
- Intelligence
- intentionally
- into
- introduce
- Issued
- issues
- IT
- ITS
- journalist
- jpg
- just
- Keep
- Kong
- Lack
- Law
- lawyer
- lead
- LEARN
- left
- Legal
- lenders
- lending
- less
- levels
- List
- Lists
- Lives
- loan
- locked
- Long
- mac
- maintain
- make
- many
- Market
- market conditions
- max-width
- Maximize
- May..
- means
- measures
- methods
- might
- missing
- Mitigate
- model
- models
- more
- movement
- much
- must
- nascent
- Natural
- nearly
- needed
- New
- no
- None
- normal
- number
- obsolete
- of
- Officer
- often
- Old
- older
- on
- once
- ONE
- only
- Opportunity
- or
- original
- Other
- our
- out
- output
- over
- overstated
- paid
- panels
- past
- patented
- peer to peer
- peer-to-peer lending
- People
- plato
- Plato Data Intelligence
- PlatoData
- players
- policy
- population
- possible
- potential
- predicting
- Predictions
- PRNewswire
- Problem
- properly
- protection
- provide
- published
- quality
- racism
- Rate
- Rates
- real
- reason
- reasons
- reducing
- reflect
- Regardless
- regulations
- Regulators
- regulatory
- relevant
- rely
- removal
- report
- Requirements
- right
- Risk
- rohit chopra
- s
- Said
- score
- see
- sept
- set
- Sets
- seven
- several
- significantly
- similar
- Similarly
- Simple
- simply
- situated
- So
- Society
- solid
- solution
- SOLVE
- some
- something
- spaces
- span
- special
- specific
- specifically
- stages
- standard
- States
- Stop
- Summit
- sure
- surprised
- surveillance
- Systems
- Take
- taken
- tangible
- technique
- Technologies
- Technology
- test
- tested
- than
- that
- The
- the Law
- their
- Them
- then
- theory
- There.
- thesis
- they
- Think
- this
- those
- though?
- three
- time
- to
- today
- Tony
- tools
- trained
- treats
- try
- Unchained
- under
- underwriting
- Update
- updated
- urban
- use
- used
- uses
- using
- Values
- very
- wanted
- was
- Way..
- ways
- we
- were
- What
- when
- white
- WHO
- why
- wide
- will
- with
- within
- Women
- worked
- working
- worried
- would
- written
- Wrong
- year
- years
- you
- zephyrnet
- Zest AI