Media In our everyday life, it has become increasingly hard to avoid hearing the words ‘Artificial Intelligence (AI)’ and ‘Machine Learning (ML)’ whether in industry or academia. These technologies have entered our daily lives and are transforming most sectors of the economy, building new spheres of knowledge and practice, and ushering a new era in human history. Yet even as these forms of rapidly evolving intelligence become more visible inside and outside the academy, their imprecise definitions, vagueness about their modalities and scope of applications impedes their full understanding. This article aims to clarify these new technologies, distinguish them from each other, and outline their sweeping implications.
1. AI/ML in the world of IoT
Artificial Intelligence (AI), Machine Learning (ML) and the Internet of Things (IoT) are intricately linked and together represent a powerful triad, ushering in a new wave of innovation. The trio is enabling a new generation of smart, self-adjusting and self-optimising autonomous products and machines, which in turn are disrupting and transforming every sector from manufacturing to health care. The link between AI and ML and IoT is a natural one:
-
Data-driven intelligence:
The generators of this data are sensors and smart devices embedded in everyday objects, in contexts as diverse as traffic networks or kitchen appliances. It is the power and prowess of AI and ML that provide the computational intelligence to process, transform and analyse the data, and turn it into actionable information. IoT forms the data-capturing layer, while AI and ML represent the analysis engine that constitutes the computational brain.
In industry, IoT devices track sensors of equipment and machinery. ML algorithms can identify links between its current data and historical data, and then predict machine or equipment failure, maintenance needs and other issues. The entire process is continuous, and the ML algorithm can predict machine conditions based on real-time data from IoT devices. For instance, if oil levels are low or there has been excessive vibration, the systems could predict a potential machine breakdown. In this way, predictive maintenance can minimise downtime and lower material costs without significantly increasing labour costs.
-
Enhanced user experience and personalisation:
Examples of these consumer applications can be found in IoT devices that collect information about users’ interactions and preferences. For instance, by analysing how you use a smart home, AI could control your lighting and temperature based on your behaviour, with machine learning algorithms improving the predictive efforts over time if you continue to use it. Fitness trackers can also use ML algorithms to personalise health recommendations.
-
Autonomous decision-making:
Using AI and ML, IoT devices can begin to take autonomous decisions based on real-time data. Autonomous vehicles (an ecosystem of IoT devices), for example, use ML to understand sensor data and decide which driving actions to take moment-by-moment on the road. In our homes and offices, energy grids use AI to balance grid loads and intelligently optimise energy distribution based on IoT data provided in real time.
-
Enhanced security:
Security and cyber attacks can creep into IoT networks. AI and ML can work like security radar and detect anomalies in the state of IoT networks or in the data generated by IoT devices to tell whether an attack is happening or about to happen. AI-powered security can therefore make IoT more secure – these systems can always learn from the data coming from the networks and update the measures to adopt.
-
Operational efficiency:
In business and manufacturing, IoTs input a lot of variables and parameters which are analysed by ML algorithms to optimise operations by cutting down on the amount of waste and improving efficiency. AI at the same time can be used to automate more complex decision-making processes, and in this way to optimise, in real time, the operation parameters.
In short, AI and ML are indispensable to IoT, and a clever system will integrate all three as an intelligent ecosystem of learning, adapting, and deciding: an IoT driver and accelerator of future innovations, itself helped along by smart regulation.
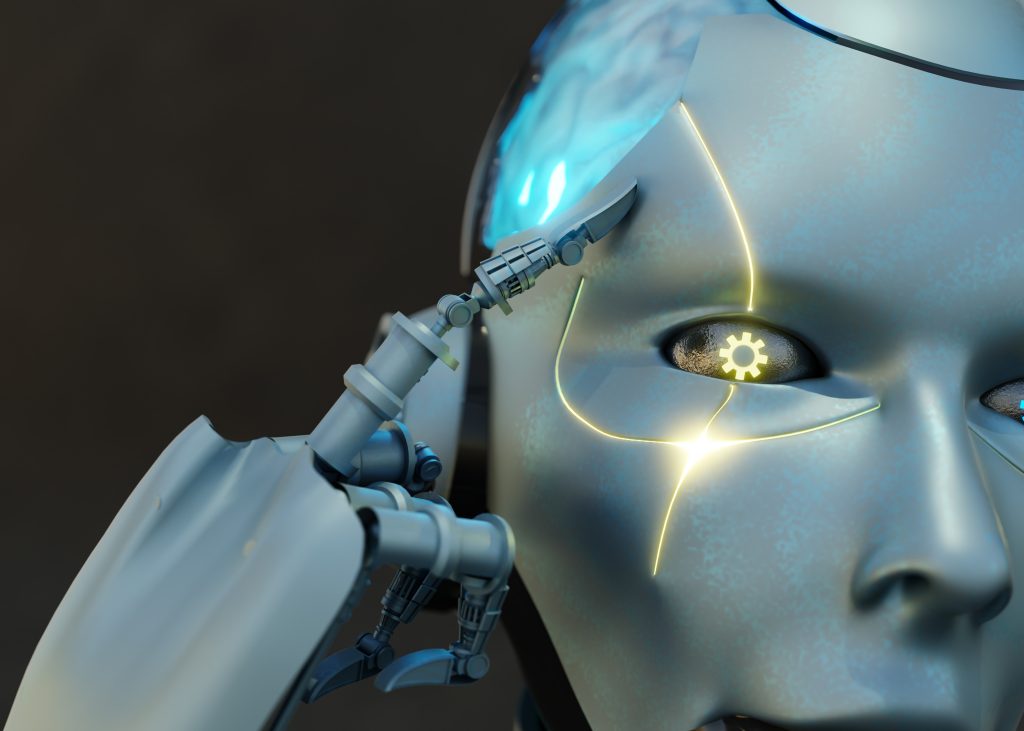
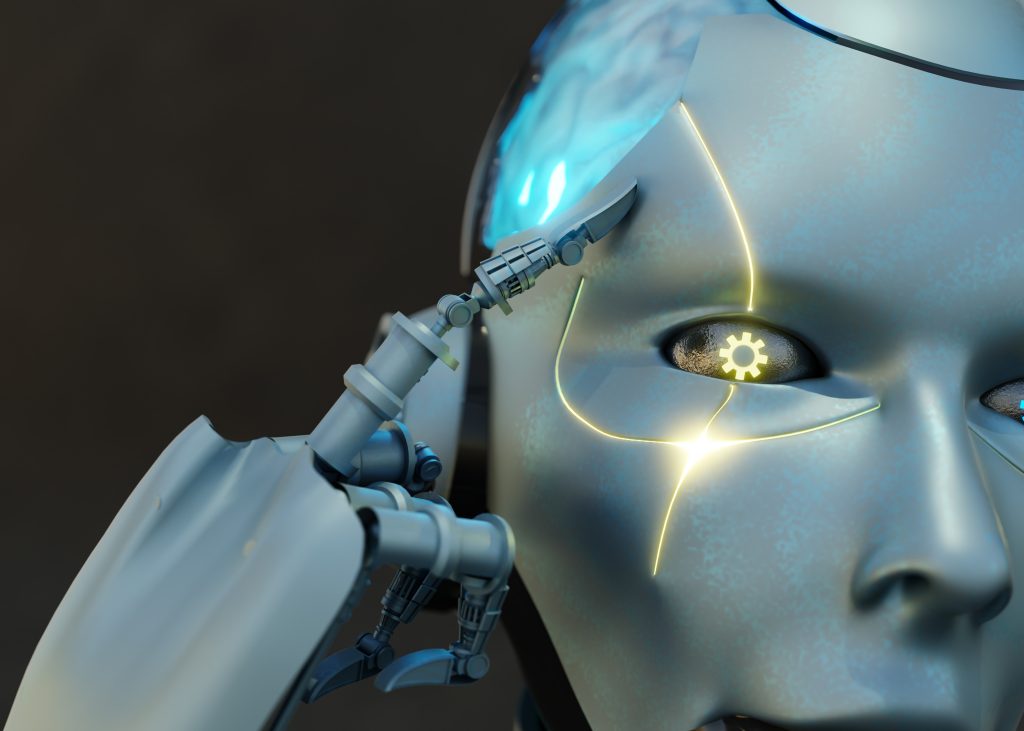
2. Decoding AI and Machine Learning: A comparative overview
Artificial Intelligence (AI)
Artificial Intelligence is a field – or we may even say discipline – of computer science that aims to create systems capable of performing tasks that are commonly regarded as requiring human intelligence. Some of its essential marks revolve around the use of concepts such as intelligence and learning, by which one correlates the AI’s task-performing capability with the human’s faculty of cognition. Examples of such tasks include the understanding of natural language – which might resonate with the human ability to speak; the pattern recognition – closely related to the human faculty of perception; and the inherently comparable ability to solve complex problems that involve unforeseeable difficulties and inexorable vagueness and uncertainty as to their solutions – like puzzles drawn from the real world for the intellectually curious human. It is widely held that, while chatbots are more narrowly goal-oriented, AIs involve the ability of computers to perform any of the abovementioned tasks in a way that would cause us to say the computer is ‘smart’. This is what is sometimes called ‘intellectual mimicry’ or ‘mimesis’ of the human intelligence – in short, to learn from experience and ‘act cleverly’.
Machine Learning (ML)
Machine Learning concerns a particularly active area of Artificial Intelligence (AI) that tries to codify the ability of computers to learn, make choices or predictions based on data, bypassing the need for human input or guidance. Algorithms are trained on previously gathered data sets until they understand the underlying patterns of that data, make informed choices based on what they have learned, and are capable of continuing to incrementally improve their predictive capacity autonomously going forward. ML’s objective is to develop programmes that are capable of exploiting data in order to become better, more adaptable at learning on their own, without intervention, task by task.
Key Differences:
AI is for building an intelligent computer that solves the problems in the analogous way as a human does, while ML allows for a robot to learn from the data so as to draw an accurate forecast.
Functionality: the machine uses a pre-written rulebook (often the system flexes and ‘tweaks’ rules based on results) versus the ML system follows a pattern-cloud of expected inputs that leads to an answer.
3. What they bring to the table: Capabilities and applications
AI’s contributions:
Artificial intelligence is quite good at automating routine tasks – whether these are straightforward jobs such as data entry, or the hidden processes that feed those decisions, it maximises efficiency and productivity.
- Cognitive services:
Thanks to cognitive services (for language understanding, speech, and vision), the computer is equipped with a wider range of interaction with humans.
- Decision making:
AI systems can reach meaningful conclusions about the present by comparing and contrasting past and present data, making informed connections and syntheses of reams of input.
ML’s contributions:
- Predictive analysis:
ML models are excellent at prediction and forecasting trends and behaviours from past data, and this is where they can be applied, like in the financial, medical and marketing sectors.
- Pattern recognition:
One of the most successful ML applications is learning to recognise buried patterns in data, such as anomalous activity in cybersecurity or tell-tale signs of disease in diagnostic medicine.
- Individualisation:
ML produces custom user experiences depending on how an individual user has interacted with the service in the past, and might be used to improve services for e-commerce, entertainment and much more.
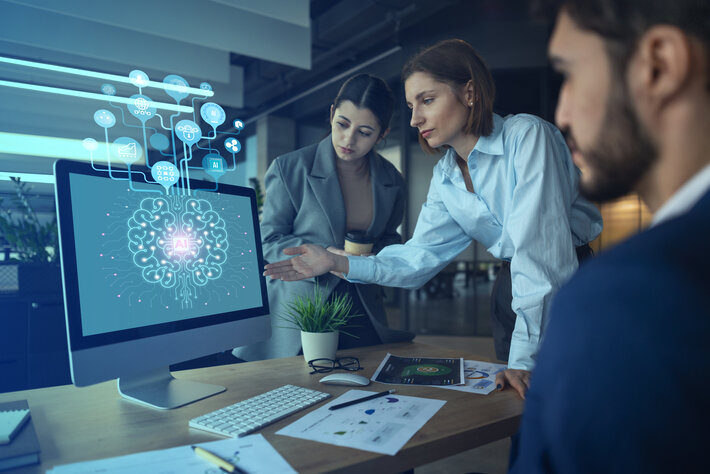
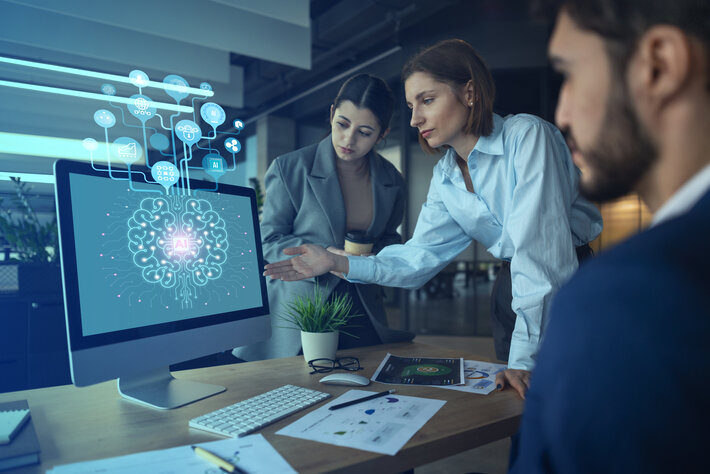
4. The synergistic relationship: How AI and ML complement each other
That relationship is also mutually supportive, with the science in one feeding back to improve and inform the other nascent science, and the resulting systems growing more capable and cognitively powerful over time. AI is the organiser: the field of artificial intelligence defines the overall goals and architectures for building machines that, in principle, can exhibit aspects of human intelligence. ML is the toolkit: the field of machine learning supplies the methods and techniques that allow these machines to learn things from data, get better with practice, and make decisions.
- Enhanced learning capabilities: So tuning into real-world ‘vibrations’ of humanity is crucial. AI systems are meant to be informed by human intelligence and ML allows machines to learn from experience, in the way that humans do. If statistical learning bridges machines and humans, then ML has some promise – the data-driven systems must learn how to ‘recalibrate’ (as humans do) when faced with new examples of ‘human’ behaviour (for eg, driving, interacting with other humans, and so on).
- Data-driven decision making: Being ‘smart’ in AI means ‘being a good decision-maker’. ML is (probably) a null hypothesis for how best to make AI fast at making decisions by giving it the tools to analyse a lot of data about whatever the AI objects are doing each moment, figure out what the patterns are in that data, and then (makes a prediction) use analysis and pattern-recognition to make the next decision.
- Predictive power and personalisation: In many other ways, ML is an effective tool to make possible what AIs are designed to achieve for users: personalised experience and outcome prediction. ML is great in delivering a personalised experience to a user of an e-commerce website, a video streaming service or a customer-service platform because it takes data points about what the user has done in the past and predicts what that user will eventually do.
- Autonomous improvement: A fundamental aspect of the concept of AI is the ability to design an autonomous system. ML takes this a step further, since systems are not just being designed to operate autonomously, but to optimise performance autonomously (for example, by learning from the data obtained after starting up). In the case of a system such as an autonomous car that needs to ‘learn’ how to deal with an unknown environment, such an improvement loop is essential. Complex.
- Problem-solving: Aupiter AI seeks to tackle the intractable in the aforementioned computational space of real-world problems, where all practical solutions appear to be hopelessly complicated, and the obvious, easy avenues run aground. ML compounds the complexity by delivering a paradigm based on blending multiple modalities (eg, sets of algorithms such as neural nets) to match real-world complexity and harness high complexity, largely unstructured data mostly available in the real world.
Put the two together, and you have an exponentially accelerating technological ecosystem – in which ML’s ability to build ‘inductive’ models, and learn to learn by iterative development from data, can be allied with AI’s even more ambitious agenda, of modelling human intelligence to create ever more generalist ‘generative’ systems that can master a wide variety of complex tasks, blast through innovation boundaries, and turbocharge entire industries.
5. Challenges and ethical considerations
With virtually every fast-advancing and potentially disruptive technologies for AI and of machine learning (ML), we rapidly find that concerns about how the technology might change the world evolve almost as quickly as the emerging and rapidly advancing technologies themselves. It is an unprecedented issue: because AI and ML systems need volume of data to operate effectively, we generate worries about data security and privacy. The broader ethical concerns include the issues of bias and fairness in the design of AI (ie, algorithms might produce biased results because they have been trained earlier on biased data) and that intentional decision processes implemented by algorithmic are more interpretable and open than human ones – especially in educational, medical and criminal justice scenarios, where transparency can be as important as a decision itself. There will be jobs eliminated in the automation process, a situation requiring much-needed workforce management and employee retraining strategies – and so on. In fact, this can be paraphrased to: THE BIG WORRIES:
At the leading edge of this work are rising calls to enunciate principles and impose standards for the design and deployment of AI and ML technologies. This is going to require a large-scale partnership across companies, policymakers and other stakeholders to ensure that AI and ML technologies are developed and deployed securely, fairly, transparently and for the public good.


6. The future outlook: Infinite possibilities
Once more, on the cusp of the next technology revolution – in AI and ML – the same applies: medicine will transform as patients are prescribed treatments based on tomographic scanning of their DNA; our urban lifeworlds will be recast in AI-driven ML towns deployed throughout our infrastructure.
Taken together, AI and ML are enabling a future, increasingly seamless and invisible, in which technology underpins so much of our reality. Knowing what separates them, what they can accomplish and where they will continue to hit walls is something that organisations, policy makers and the general population alike will be well served to understand in the years to come. With these technologies still evolving, entirely new worlds will emerge, others fall away, and the world around us will continue changing through eyes that cannot yet begin to see. The AI revolution is only just beginning. The possibilities are as limitless as our imaginations will allow.
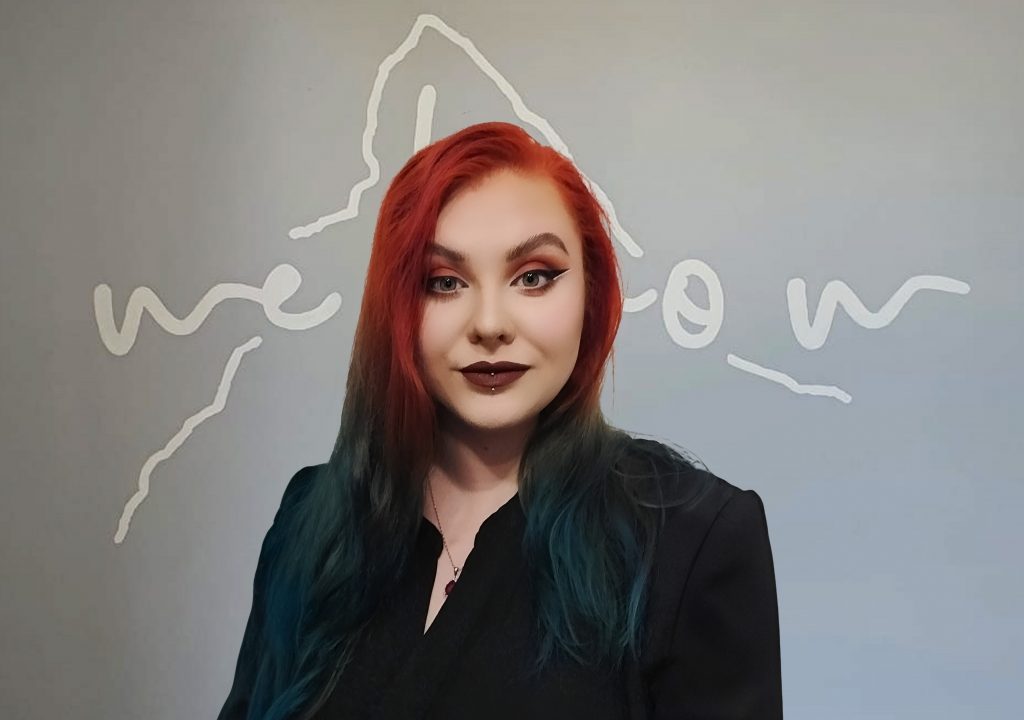
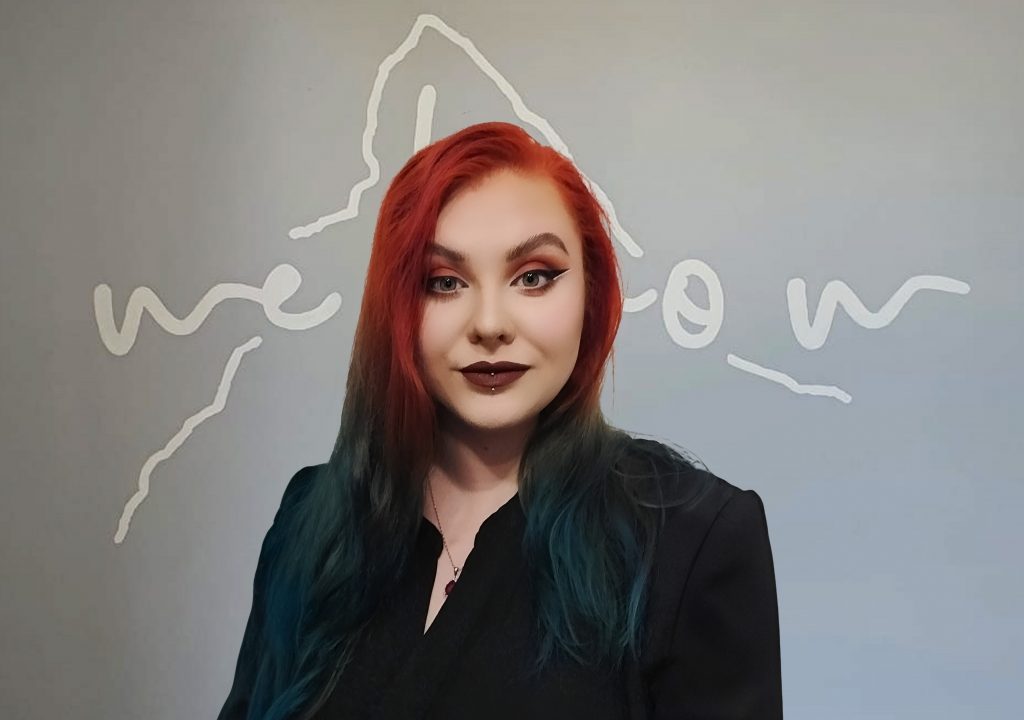
Article by Magda Dąbrowska, a technical writer at WeKnow Media
Comment on this article below or via Twitter: @IoTNow_
- SEO Powered Content & PR Distribution. Get Amplified Today.
- PlatoData.Network Vertical Generative Ai. Empower Yourself. Access Here.
- PlatoAiStream. Web3 Intelligence. Knowledge Amplified. Access Here.
- PlatoESG. Carbon, CleanTech, Energy, Environment, Solar, Waste Management. Access Here.
- PlatoHealth. Biotech and Clinical Trials Intelligence. Access Here.
- Source: https://www.iot-now.com/2024/02/02/142229-ai-vs-ml-decoding-the-technologies-shaping-our-world/
- :has
- :is
- :not
- :where
- $UP
- 220
- a
- ability
- About
- Academia
- Academy
- accelerating
- accelerator
- accomplish
- accurate
- Achieve
- across
- actionable
- actions
- active
- activity
- adapting
- adopt
- advancing
- After
- agenda
- AI
- AI systems
- AI-powered
- AI/ML
- aims
- ais
- algorithm
- algorithmic
- algorithms
- alike
- All
- allow
- allows
- almost
- along
- also
- always
- ambitious
- amount
- an
- analyse
- analysing
- analysis
- and
- answer
- any
- appear
- appliances
- applications
- applied
- applies
- architectures
- ARE
- AREA
- around
- article
- artificial
- artificial intelligence
- Artificial intelligence (AI)
- AS
- aspect
- aspects
- At
- attack
- automate
- automating
- Automation
- autonomous
- autonomous car
- autonomous vehicles
- autonomously
- available
- avenues
- avoid
- away
- back
- Balance
- based
- BE
- because
- become
- been
- begin
- Beginning
- behaviour
- behaviours
- being
- below
- BEST
- Better
- between
- bias
- biased
- Big
- blending
- boundaries
- Brain
- Breakdown
- bridges
- bring
- broader
- build
- Building
- business
- but
- by
- called
- Calls
- CAN
- cannot
- capabilities
- capability
- capable
- Capacity
- car
- care
- case
- Cause
- challenges
- change
- changing
- chatbots
- choices
- clever
- closely
- cognition
- cognitive
- collect
- come
- coming
- commonly
- Companies
- comparable
- comparing
- Complement
- complex
- complexity
- complicated
- computational
- computer
- computer science
- computers
- concept
- concepts
- Concerns
- Conclusions
- conditions
- Connections
- considerations
- consumer
- contexts
- continue
- continuing
- continuous
- contributions
- control
- Costs
- could
- create
- Criminal
- criminal justice
- crucial
- curious
- Current
- Cusp
- custom
- cutting
- cyber
- Cybersecurity
- daily
- data
- data entry
- data points
- data security
- data security and privacy
- data sets
- data-driven
- deal
- decide
- Deciding
- decision
- Decision Making
- decisions
- Decoding
- Defines
- definitions
- delivering
- Depending
- deployed
- deployment
- Design
- designed
- detect
- develop
- developed
- Development
- Devices
- diagnostic
- differences
- difficulties
- discipline
- Disease
- disruptive
- distinguish
- distribution
- diverse
- dna
- do
- does
- doing
- done
- down
- downtime
- draw
- drawn
- driver
- driving
- e-commerce
- each
- Earlier
- easy
- economy
- ecosystem
- Edge
- educational
- Effective
- effectively
- efficiency
- efforts
- eliminated
- embedded
- emerge
- emerging
- Employee
- enabling
- energy
- Engine
- ensure
- entered
- Entertainment
- Entire
- entirely
- entry
- Environment
- equipment
- equipped
- Era
- especially
- essential
- ethical
- Even
- eventually
- EVER
- Every
- everyday
- evolve
- evolving
- example
- examples
- excellent
- excessive
- exhibit
- expected
- experience
- Experiences
- exploiting
- exponentially
- Eyes
- faced
- fact
- Failure
- fairly
- fairness
- Fall
- FAST
- feeding
- field
- Figure
- financial
- Find
- fitness
- follows
- For
- Forecast
- forms
- Forward
- found
- from
- full
- fundamental
- further
- future
- gathered
- General
- generate
- generated
- generation
- generators
- get
- Giving
- Goals
- going
- good
- great
- Grid
- Growing
- guidance
- happen
- Happening
- Hard
- harness
- Have
- Health
- Health Care
- hearing
- Held
- helped
- Hidden
- High
- historical
- history
- Hit
- Home
- Homes
- How
- How To
- HTTPS
- human
- human intelligence
- Humanity
- Humans
- IBM
- identify
- ie
- if
- image
- imaginations
- implemented
- implications
- important
- impose
- improve
- improvement
- improving
- in
- include
- increasing
- increasingly
- individual
- industries
- industry
- inform
- information
- informed
- Infrastructure
- inherently
- Innovation
- innovations
- input
- inputs
- inside
- instance
- integrate
- Intelligence
- Intelligent
- Intentional
- interacting
- interaction
- interactions
- intervention
- into
- invisible
- involve
- iot
- iot devices
- issue
- issues
- IT
- ITS
- itself
- Job
- Jobs
- jpg
- just
- Justice
- kitchen
- Knowing
- knowledge
- Labour
- language
- large-scale
- largely
- layer
- leading
- Leads
- LEARN
- learned
- learning
- levels
- Life
- Lighting
- like
- limitless
- LINK
- linked
- links
- Lives
- loads
- Lot
- Low
- lower
- machine
- machine learning
- Machines
- maintenance
- make
- Makers
- MAKES
- Making
- management
- manufacturing
- many
- Marketing
- master
- Match
- material
- max-width
- May..
- meaningful
- means
- meant
- measures
- Media
- medical
- medicine
- methods
- might
- minimise
- ML
- ML algorithms
- modalities
- modelling
- models
- moment
- more
- most
- mostly
- much
- much-needed
- multiple
- must
- mutually
- nascent
- Natural
- Natural Language
- Need
- needs
- networks
- New
- New technologies
- news
- next
- now
- objective
- objects
- obtained
- obvious
- of
- offices
- often
- Oil
- on
- ONE
- ones
- only
- open
- operate
- operation
- Operations
- optimise
- or
- order
- Organisations
- Other
- Others
- our
- out
- Outcome
- outline
- Outlook
- outside
- over
- overall
- overview
- own
- paradigm
- parameters
- particularly
- Partnership
- past
- patients
- patterns
- perception
- perform
- performance
- performing
- person
- Personalised
- platform
- plato
- Plato Data Intelligence
- PlatoData
- points
- policy
- Policy Makers
- policymakers
- population
- possibilities
- possible
- potential
- potentially
- power
- powerful
- Practical
- practice
- predict
- prediction
- Predictions
- predictive
- Predicts
- preferences
- present
- previously
- principle
- principles
- privacy
- probably
- problems
- process
- processes
- produce
- produces
- productivity
- Products
- programmes
- promise
- provide
- provided
- prowess
- public
- Puzzles
- quickly
- quite
- radar
- range
- rapidly
- reach
- real
- real world
- real-time
- real-time data
- Reality
- recognise
- recognition
- recommendations
- regarded
- Regulation
- related
- relationship
- Reports
- represent
- require
- Resonate
- resulting
- Results
- retraining
- Revolution
- rising
- road
- robot
- routine
- rules
- Run
- same
- say
- scanning
- scenarios
- Science
- scope
- seamless
- sector
- Sectors
- secure
- securely
- security
- see
- Seeks
- sensors
- served
- service
- Services
- Sets
- shaping
- Short
- significantly
- Signs
- since
- situation
- smart
- Smart home
- So
- Solutions
- SOLVE
- Solves
- some
- something
- sometimes
- Space
- speak
- speech
- stakeholders
- standards
- Starting
- State
- statistical
- Step
- Still
- straightforward
- strategies
- streaming
- streaming service
- successful
- such
- supplies
- supportive
- synergistic
- system
- Systems
- table
- tackle
- Take
- takes
- Task
- tasks
- Technical
- techniques
- technological
- Technologies
- Technology
- tell
- than
- that
- The
- The Future
- The State
- the world
- their
- Them
- themselves
- then
- There.
- therefore
- These
- they
- things
- this
- those
- three
- Through
- throughout
- time
- to
- together
- tool
- tools
- towns
- Trackers
- traffic
- trained
- Transform
- transforming
- Transparency
- transparently
- treatments
- Trends
- tries
- trio
- tuning
- TURN
- two
- Uncertainty
- underlying
- understand
- understanding
- unknown
- unprecedented
- unstructured
- until
- Update
- urban
- us
- use
- used
- User
- User Experience
- users
- uses
- ushering
- using
- variables
- variety
- Vehicles
- Versus
- via
- Video
- virtually
- visible
- vision
- volume
- vs
- Waste
- Wave
- Way..
- ways
- we
- Website
- WELL
- What
- What is
- whatever
- when
- whether
- which
- while
- wide
- widely
- wider
- will
- with
- without
- words
- Work
- Workforce
- world
- world’s
- would
- writer
- years
- yet
- you
- Your
- zephyrnet