1Theoretical Division, Los Alamos National Laboratory, Los Alamos, NM 87545, USA
2Department of Physics, Imperial College London, London, SW7 2AZ, UK
3Faculty of Physics, Astronomy, and Applied Computer Science, Jagiellonian University, Kraków, Poland
4Mark Kac Center for Complex Systems Research, Jagiellonian University, Kraków, Poland
5Quantum Science Center, Oak Ridge, TN 37931, USA
6Center for Nonlinear Studies, Los Alamos National Laboratory, Los Alamos, NM 87545, USA
Find this paper interesting or want to discuss? Scite or leave a comment on SciRate.
Abstract
Variational Quantum Algorithms (VQAs) are often viewed as the best hope for near-term quantum advantage. However, recent studies have shown that noise can severely limit the trainability of VQAs, e.g., by exponentially flattening the cost landscape and suppressing the magnitudes of cost gradients. Error Mitigation (EM) shows promise in reducing the impact of noise on near-term devices. Thus, it is natural to ask whether EM can improve the trainability of VQAs. In this work, we first show that, for a broad class of EM strategies, exponential cost concentration cannot be resolved without committing exponential resources elsewhere. This class of strategies includes as special cases Zero Noise Extrapolation, Virtual Distillation, Probabilistic Error Cancellation, and Clifford Data Regression. Second, we perform analytical and numerical analysis of these EM protocols, and we find that some of them (e.g., Virtual Distillation) can make it harder to resolve cost function values compared to running no EM at all. As a positive result, we do find numerical evidence that Clifford Data Regression (CDR) can aid the training process in certain settings where cost concentration is not too severe. Our results show that care should be taken in applying EM protocols as they can either worsen or not improve trainability. On the other hand, our positive results for CDR highlight the possibility of engineering error mitigation methods to improve trainability.
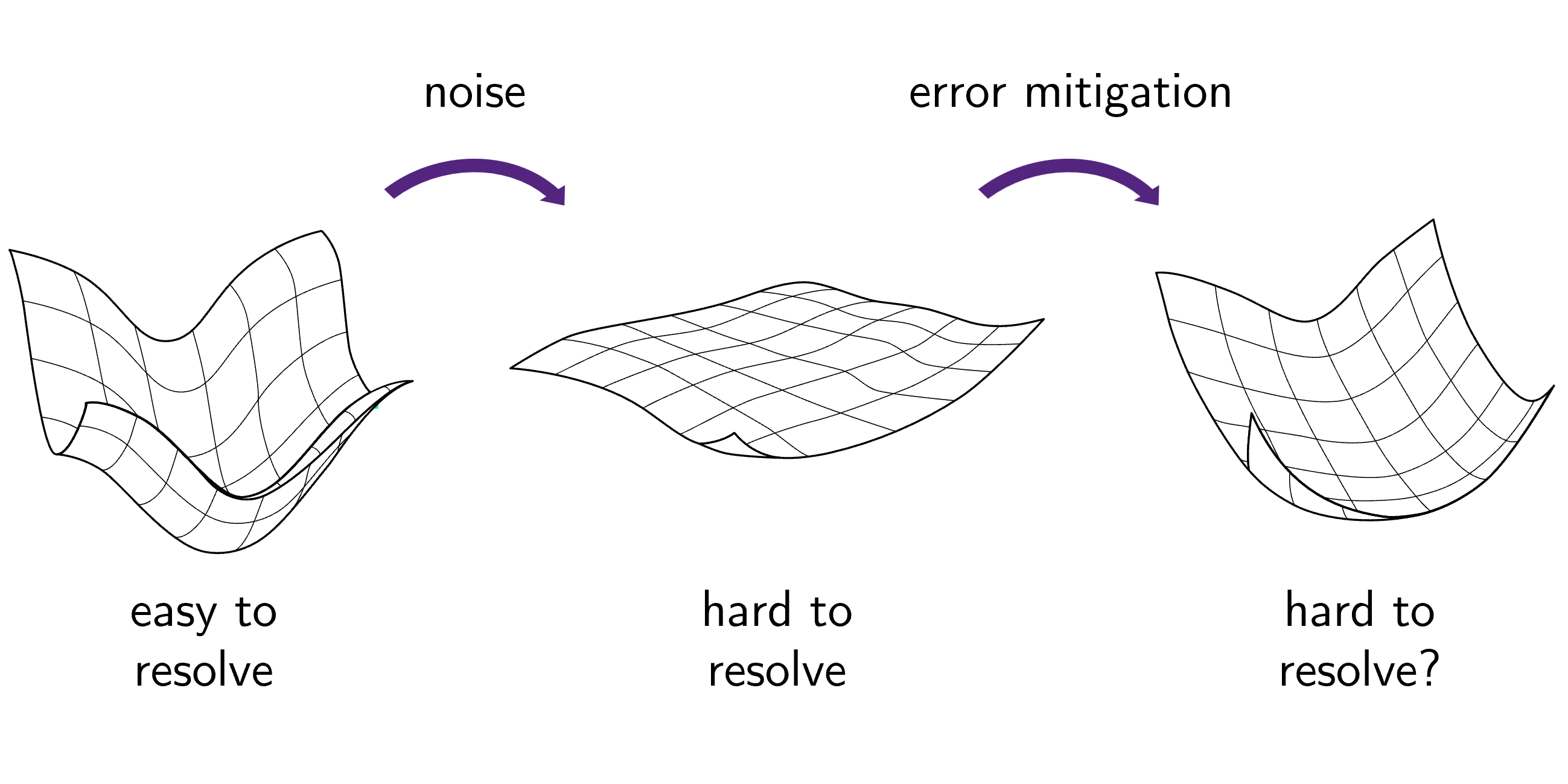
Featured image: Certain types of hardware noise have been found to exponentially impair the resolvability of cost landscapes in variational quantum algorithms. Thus, accurate and reliable optimization of noisy variational quantum algorithms can be burdened with exponential sample overhead. In this work we ask whether error mitigation can mitigate this phenomenon, both from a scaling point of view (in the limit of large system sizes), and for fixed problem sizes.
â–º BibTeX data
â–º References
[1] Jarrod R McClean, Jonathan Romero, Ryan Babbush, and Alán Aspuru-Guzik. “The theory of variational hybrid quantum-classical algorithms”. New Journal of Physics 18, 023023 (2016).
https:/​/​doi.org/​10.1007/​978-94-015-8330-5_4
[2] M. Cerezo, Andrew Arrasmith, Ryan Babbush, Simon C Benjamin, Suguru Endo, Keisuke Fujii, Jarrod R McClean, Kosuke Mitarai, Xiao Yuan, Lukasz Cincio, and Patrick J. Coles. “Variational quantum algorithms”. Nature Reviews Physics 3, 625–644 (2021).
https:/​/​doi.org/​10.1038/​s42254-021-00348-9
[3] Sam McArdle, Tyson Jones, Suguru Endo, Ying Li, Simon C Benjamin, and Xiao Yuan. “Variational ansatz-based quantum simulation of imaginary time evolution”. npj Quantum Information 5, 1–6 (2019).
https:/​/​doi.org/​10.1038/​s41534-019-0187-2
[4] Harper R Grimsley, Sophia E Economou, Edwin Barnes, and Nicholas J Mayhall. “An adaptive variational algorithm for exact molecular simulations on a quantum computer”. Nature Communications 10, 1–9 (2019).
https:/​/​doi.org/​10.1038/​s41467-019-10988-2
[5] Cristina Cirstoiu, Zoe Holmes, Joseph Iosue, Lukasz Cincio, Patrick J. Coles, and Andrew Sornborger. “Variational fast forwarding for quantum simulation beyond the coherence time”. npj Quantum Information 6, 1–10 (2020).
https:/​/​doi.org/​10.1038/​s41534-020-00302-0
[6] Benjamin Commeau, M. Cerezo, Zoë Holmes, Lukasz Cincio, Patrick J. Coles, and Andrew Sornborger. “Variational hamiltonian diagonalization for dynamical quantum simulation”. arXiv preprint arXiv:2009.02559 (2020).
https:/​/​doi.org/​10.48550/​arXiv.2009.02559
[7] Joe Gibbs, Kaitlin Gili, Zoë Holmes, Benjamin Commeau, Andrew Arrasmith, Lukasz Cincio, Patrick J. Coles, and Andrew Sornborger. “Long-time simulations with high fidelity on quantum hardware”. arXiv preprint arXiv:2102.04313 (2021).
https:/​/​doi.org/​10.48550/​arXiv.2102.04313
[8] Yong-Xin Yao, Niladri Gomes, Feng Zhang, Thomas Iadecola, Cai-Zhuang Wang, Kai-Ming Ho, and Peter P Orth. “Adaptive variational quantum dynamics simulations”. arXiv preprint arXiv:2011.00622 (2020).
https:/​/​doi.org/​10.1103/​PRXQuantum.2.030307
[9] Suguru Endo, Jinzhao Sun, Ying Li, Simon C Benjamin, and Xiao Yuan. “Variational quantum simulation of general processes”. Physical Review Letters 125, 010501 (2020).
https:/​/​doi.org/​10.1103/​PhysRevLett.125.010501
[10] Y. Li and S. C. Benjamin. “Efficient variational quantum simulator incorporating active error minimization”. Phys. Rev. X 7, 021050 (2017).
https:/​/​doi.org/​10.1103/​PhysRevX.7.021050
[11] Jonathan Wei Zhong Lau, Kishor Bharti, Tobias Haug, and Leong Chuan Kwek. “Quantum assisted simulation of time dependent hamiltonians”. arXiv preprint arXiv:2101.07677 (2021).
https:/​/​doi.org/​10.48550/​arXiv.2101.07677
[12] Kentaro Heya, Ken M Nakanishi, Kosuke Mitarai, and Keisuke Fujii. “Subspace variational quantum simulator”. arXiv preprint arXiv:1904.08566 (2019).
https:/​/​doi.org/​10.48550/​arXiv.1904.08566
[13] Xiao Yuan, Suguru Endo, Qi Zhao, Ying Li, and Simon C Benjamin. “Theory of variational quantum simulation”. Quantum 3, 191 (2019).
https:/​/​doi.org/​10.22331/​q-2019-10-07-191
[14] Maria Schuld, Alex Bocharov, Krysta M Svore, and Nathan Wiebe. “Circuit-centric quantum classifiers”. Physical Review A 101, 032308 (2020).
https:/​/​doi.org/​10.1103/​PhysRevA.101.032308
[15] Guillaume Verdon, Michael Broughton, and Jacob Biamonte. “A quantum algorithm to train neural networks using low-depth circuits”. arXiv preprint arXiv:1712.05304 (2017).
https:/​/​doi.org/​10.48550/​arXiv.1712.05304
[16] Jonathan Romero and Alán Aspuru-Guzik. “Variational quantum generators: Generative adversarial quantum machine learning for continuous distributions”. Advanced Quantum Technologies 4, 2000003 (2021).
https:/​/​doi.org/​10.1002/​qute.202000003
[17] Edward Farhi and Hartmut Neven. “Classification with quantum neural networks on near term processors”. arXiv preprint arXiv:1802.06002 (2018).
https:/​/​doi.org/​10.48550/​arXiv.1802.06002
[18] Kerstin Beer, Dmytro Bondarenko, Terry Farrelly, Tobias J. Osborne, Robert Salzmann, Daniel Scheiermann, and Ramona Wolf. “Training deep quantum neural networks”. Nature Communications 11, 808 (2020).
https:/​/​doi.org/​10.1038/​s41467-020-14454-2
[19] Iris Cong, Soonwon Choi, and Mikhail D Lukin. “Quantum convolutional neural networks”. Nature Physics 15, 1273–1278 (2019).
https:/​/​doi.org/​10.1038/​s41567-019-0648-8
[20] Edward Grant, Marcello Benedetti, Shuxiang Cao, Andrew Hallam, Joshua Lockhart, Vid Stojevic, Andrew G Green, and Simone Severini. “Hierarchical quantum classifiers”. npj Quantum Information 4, 1–8 (2018).
https:/​/​doi.org/​10.1038/​s41534-018-0116-9
[21] Alberto Peruzzo, Jarrod McClean, Peter Shadbolt, Man-Hong Yung, Xiao-Qi Zhou, Peter J Love, Alán Aspuru-Guzik, and Jeremy L O’brien. “A variational eigenvalue solver on a photonic quantum processor”. Nature Communications 5, 1–7 (2014).
https:/​/​doi.org/​10.1038/​ncomms5213
[22] Bela Bauer, Dave Wecker, Andrew J Millis, Matthew B Hastings, and Matthias Troyer. “Hybrid quantum-classical approach to correlated materials”. Physical Review X 6, 031045 (2016).
https:/​/​doi.org/​10.1103/​PhysRevX.6.031045
[23] Tyson Jones, Suguru Endo, Sam McArdle, Xiao Yuan, and Simon C Benjamin. “Variational quantum algorithms for discovering hamiltonian spectra”. Physical Review A 99, 062304 (2019).
https:/​/​doi.org/​10.1103/​PhysRevA.99.062304
[24] Edward Farhi, Jeffrey Goldstone, and Sam Gutmann. “A quantum approximate optimization algorithm”. arXiv preprint arXiv:1411.4028 (2014).
https:/​/​doi.org/​10.48550/​arXiv.1411.4028
[25] Zhihui Wang, S. Hadfield, Z. Jiang, and E. G. Rieffel. “Quantum approximate optimization algorithm for MaxCut: A fermionic view”. Physical Review A 97, 022304 (2018).
https:/​/​doi.org/​10.1103/​PhysRevA.97.022304
[26] Gavin E Crooks. “Performance of the quantum approximate optimization algorithm on the maximum cut problem”. arXiv preprint arXiv:1811.08419 (2018).
https:/​/​doi.org/​10.48550/​arXiv.1811.08419
[27] Stuart Hadfield, Zhihui Wang, Bryan O’Gorman, Eleanor G Rieffel, Davide Venturelli, and Rupak Biswas. “From the quantum approximate optimization algorithm to a quantum alternating operator ansatz”. Algorithms 12, 34 (2019).
https:/​/​doi.org/​10.3390/​a12020034
[28] Carlos Bravo-Prieto, Ryan LaRose, M. Cerezo, Yigit Subasi, Lukasz Cincio, and Patrick Coles. “Variational quantum linear solver”. arXiv preprint arXiv:1909.05820 (2019).
https:/​/​doi.org/​10.22331/​q-2023-11-22-1188
[29] Xiaosi Xu, Jinzhao Sun, Suguru Endo, Ying Li, Simon C Benjamin, and Xiao Yuan. “Variational algorithms for linear algebra”. Science Bulletin 66, 2181–2188 (2021).
https:/​/​doi.org/​10.1016/​j.scib.2021.06.023
[30] Bálint Koczor, Suguru Endo, Tyson Jones, Yuichiro Matsuzaki, and Simon C Benjamin. “Variational-state quantum metrology”. New Journal of Physics (2020).
https:/​/​doi.org/​10.1088/​1367-2630/​ab965e
[31] Johannes Jakob Meyer, Johannes Borregaard, and Jens Eisert. “A variational toolbox for quantum multi-parameter estimation”. NPJ Quantum Information 7, 1–5 (2021).
https:/​/​doi.org/​10.1038/​s41534-021-00425-y
[32] Eric Anschuetz, Jonathan Olson, Alán Aspuru-Guzik, and Yudong Cao. “Variational quantum factoring”. Quantum Technology and Optimization Problems (2019).
https:/​/​doi.org/​10.1007/​978-3-030-14082-3_7
[33] Sumeet Khatri, Ryan LaRose, Alexander Poremba, Lukasz Cincio, Andrew T Sornborger, and Patrick J Coles. “Quantum-assisted quantum compiling”. Quantum 3, 140 (2019).
https:/​/​doi.org/​10.22331/​q-2019-05-13-140
[34] Kunal Sharma, Sumeet Khatri, M. Cerezo, and Patrick J Coles. “Noise resilience of variational quantum compiling”. New Journal of Physics 22, 043006 (2020).
https:/​/​doi.org/​10.1088/​1367-2630/​ab784c
[35] Tyson Jones and Simon C Benjamin. “Quantum compilation and circuit optimisation via energy dissipation”. arXiv preprint arXiv:1811.03147 (2018).
https:/​/​doi.org/​10.22331/​q-2022-01-24-628
[36] Andrew Arrasmith, Lukasz Cincio, Andrew T Sornborger, Wojciech H Zurek, and Patrick J Coles. “Variational consistent histories as a hybrid algorithm for quantum foundations”. Nature Communications 10, 1–7 (2019).
https:/​/​doi.org/​10.1038/​s41467-019-11417-0
[37] M. Cerezo, Kunal Sharma, Andrew Arrasmith, and Patrick J Coles. “Variational quantum state eigensolver”. arXiv preprint arXiv:2004.01372 (2020).
https:/​/​doi.org/​10.1038/​s41534-022-00611-6
[38] Ryan LaRose, Arkin Tikku, Étude O’Neel-Judy, Lukasz Cincio, and Patrick J Coles. “Variational quantum state diagonalization”. npj Quantum Information 5, 1–10 (2019).
https:/​/​doi.org/​10.1038/​s41534-019-0167-6
[39] Guillaume Verdon, Jacob Marks, Sasha Nanda, Stefan Leichenauer, and Jack Hidary. “Quantum Hamiltonian-based models and the variational quantum thermalizer algorithm”. arXiv preprint arXiv:1910.02071 (2019).
https:/​/​doi.org/​10.48550/​arXiv.1910.02071
[40] Peter D Johnson, Jonathan Romero, Jonathan Olson, Yudong Cao, and Alán Aspuru-Guzik. “Qvector: an algorithm for device-tailored quantum error correction”. arXiv preprint arXiv:1711.02249 (2017).
https:/​/​doi.org/​10.48550/​arXiv.1711.02249
[41] John Preskill. “Quantum computing in the NISQ era and beyond”. Quantum 2, 79 (2018).
https:/​/​doi.org/​10.22331/​q-2018-08-06-79
[42] Kristan Temme, Sergey Bravyi, and Jay M. Gambetta. “Error mitigation for short-depth quantum circuits”. Phys. Rev. Lett. 119, 180509 (2017).
https:/​/​doi.org/​10.1103/​PhysRevLett.119.180509
[43] Suguru Endo, Simon C Benjamin, and Ying Li. “Practical quantum error mitigation for near-future applications”. Physical Review X 8, 031027 (2018).
https:/​/​doi.org/​10.1103/​PhysRevX.8.031027
[44] Abhinav Kandala, Kristan Temme, Antonio D. Córcoles, Antonio Mezzacapo, Jerry M. Chow, and Jay M. Gambetta. “Error mitigation extends the computational reach of a noisy quantum processor”. Nature 567, 491–495 (2019).
https:/​/​doi.org/​10.1038/​s41586-019-1040-7
[45] Piotr Czarnik, Andrew Arrasmith, Patrick J. Coles, and Lukasz Cincio. “Error mitigation with Clifford quantum-circuit data”. Quantum 5, 592 (2021).
https:/​/​doi.org/​10.22331/​q-2021-11-26-592
[46] William J Huggins, Sam McArdle, Thomas E O’Brien, Joonho Lee, Nicholas C Rubin, Sergio Boixo, K Birgitta Whaley, Ryan Babbush, and Jarrod R McClean. “Virtual distillation for quantum error mitigation”. Physical Review X 11, 041036 (2021).
https:/​/​doi.org/​10.1103/​PhysRevX.11.041036
[47] Bálint Koczor. “Exponential error suppression for near-term quantum devices”. Physical Review X 11, 031057 (2021).
https:/​/​doi.org/​10.1103/​PhysRevX.11.031057
[48] Jarrod R McClean, Mollie E Kimchi-Schwartz, Jonathan Carter, and Wibe A De Jong. “Hybrid quantum-classical hierarchy for mitigation of decoherence and determination of excited states”. Physical Review A 95, 042308 (2017).
https:/​/​doi.org/​10.1103/​PhysRevA.95.042308
[49] Thomas E. O’Brien, Stefano Polla, Nicholas C. Rubin, William J. Huggins, Sam McArdle, Sergio Boixo, Jarrod R. McClean, and Ryan Babbush. “Error mitigation via verified phase estimation”. PRX Quantum 2, 020317 (2021).
https:/​/​doi.org/​10.1103/​PRXQuantum.2.020317
[50] Sam McArdle, Xiao Yuan, and Simon Benjamin. “Error-mitigated digital quantum simulation”. Phys. Rev. Lett. 122, 180501 (2019).
https:/​/​doi.org/​10.1103/​PhysRevLett.122.180501
[51] Xavi Bonet-Monroig, Ramiro Sagastizabal, M Singh, and TE O’Brien. “Low-cost error mitigation by symmetry verification”. Physical Review A 98, 062339 (2018).
https:/​/​doi.org/​10.1103/​PhysRevA.98.062339
[52] William J Huggins, Jarrod R McClean, Nicholas C Rubin, Zhang Jiang, Nathan Wiebe, K Birgitta Whaley, and Ryan Babbush. “Efficient and noise resilient measurements for quantum chemistry on near-term quantum computers”. npj Quantum Information 7, 1–9 (2021).
https:/​/​doi.org/​10.1038/​s41534-020-00341-7
[53] George S Barron and Christopher J Wood. “Measurement error mitigation for variational quantum algorithms”. arXiv preprint arXiv:2010.08520 (2020).
https:/​/​doi.org/​10.48550/​arXiv.2010.08520
[54] Alistair W. R. Smith, Kiran E. Khosla, Chris N. Self, and M. S. Kim. “Qubit readout error mitigation with bit-flip averaging”. Science Advances 7 (2021).
https:/​/​doi.org/​10.1126/​sciadv.abi8009
[55] Daiqin Su, Robert Israel, Kunal Sharma, Haoyu Qi, Ish Dhand, and Kamil Brádler. “Error mitigation on a near-term quantum photonic device”. Quantum 5, 452 (2021).
https:/​/​doi.org/​10.22331/​q-2021-05-04-452
[56] Samson Wang, Enrico Fontana, M. Cerezo, Kunal Sharma, Akira Sone, Lukasz Cincio, and Patrick J Coles. “Noise-induced barren plateaus in variational quantum algorithms”. Nature Communications 12, 1–11 (2021).
https:/​/​doi.org/​10.1038/​s41467-021-27045-6
[57] Daniel Stilck França and Raul Garcia-Patron. “Limitations of optimization algorithms on noisy quantum devices”. Nature Physics 17, 1221–1227 (2021).
https:/​/​doi.org/​10.1038/​s41567-021-01356-3
[58] Jarrod R McClean, Sergio Boixo, Vadim N Smelyanskiy, Ryan Babbush, and Hartmut Neven. “Barren plateaus in quantum neural network training landscapes”. Nature Communications 9, 1–6 (2018).
https:/​/​doi.org/​10.1038/​s41467-018-07090-4
[59] M. Cerezo, Akira Sone, Tyler Volkoff, Lukasz Cincio, and Patrick J Coles. “Cost function dependent barren plateaus in shallow parametrized quantum circuits”. Nature Communications 12, 1–12 (2021).
https:/​/​doi.org/​10.1038/​s41467-021-21728-w
[60] Andrew Arrasmith, M. Cerezo, Piotr Czarnik, Lukasz Cincio, and Patrick J Coles. “Effect of barren plateaus on gradient-free optimization”. Quantum 5, 558 (2021).
https:/​/​doi.org/​10.22331/​q-2021-10-05-558
[61] M. Cerezo and Patrick J Coles. “Higher order derivatives of quantum neural networks with barren plateaus”. Quantum Science and Technology 6, 035006 (2021).
https:/​/​doi.org/​10.1088/​2058-9565/​abf51a
[62] Kentaro Heya, Yasunari Suzuki, Yasunobu Nakamura, and Keisuke Fujii. “Variational quantum gate optimization”. arXiv preprint arXiv:1810.12745 (2018).
https:/​/​doi.org/​10.48550/​arXiv.1810.12745
[63] Jonathan Romero, Jonathan P Olson, and Alan Aspuru-Guzik. “Quantum autoencoders for efficient compression of quantum data”. Quantum Science and Technology 2, 045001 (2017).
https:/​/​doi.org/​10.1088/​2058-9565/​aa8072
[64] Lennart Bittel and Martin Kliesch. “Training variational quantum algorithms is np-hard”. Phys. Rev. Lett. 127, 120502 (2021).
https:/​/​doi.org/​10.1103/​PhysRevLett.127.120502
[65] Jonas M Kübler, Andrew Arrasmith, Lukasz Cincio, and Patrick J Coles. “An adaptive optimizer for measurement-frugal variational algorithms”. Quantum 4, 263 (2020).
https:/​/​doi.org/​10.22331/​q-2020-05-11-263
[66] Andrew Arrasmith, Lukasz Cincio, Rolando D Somma, and Patrick J Coles. “Operator sampling for shot-frugal optimization in variational algorithms”. arXiv preprint arXiv:2004.06252 (2020).
https:/​/​doi.org/​10.48550/​arXiv.2004.06252
[67] Andi Gu, Angus Lowe, Pavel A Dub, Patrick J. Coles, and Andrew Arrasmith. “Adaptive shot allocation for fast convergence in variational quantum algorithms”. arXiv preprint arXiv:2108.10434 (2021).
https:/​/​doi.org/​10.48550/​arXiv.2108.10434
[68] Zoë Holmes, Kunal Sharma, M. Cerezo, and Patrick J Coles. “Connecting ansatz expressibility to gradient magnitudes and barren plateaus”. PRX Quantum 3, 010313 (2022).
https:/​/​doi.org/​10.1103/​PRXQuantum.3.010313
[69] Zoë Holmes, Andrew Arrasmith, Bin Yan, Patrick J. Coles, Andreas Albrecht, and Andrew T Sornborger. “Barren plateaus preclude learning scramblers”. Physical Review Letters 126, 190501 (2021).
https:/​/​doi.org/​10.1103/​PhysRevLett.126.190501
[70] Carlos Ortiz Marrero, Mária Kieferová, and Nathan Wiebe. “Entanglement-induced barren plateaus”. PRX Quantum 2, 040316 (2021).
https:/​/​doi.org/​10.1103/​PRXQuantum.2.040316
[71] Taylor L Patti, Khadijeh Najafi, Xun Gao, and Susanne F Yelin. “Entanglement devised barren plateau mitigation”. Physical Review Research 3, 033090 (2021).
https:/​/​doi.org/​10.1103/​PhysRevResearch.3.033090
[72] Martin Larocca, Piotr Czarnik, Kunal Sharma, Gopikrishnan Muraleedharan, Patrick J. Coles, and M. Cerezo. “Diagnosing barren plateaus with tools from quantum optimal control”. arXiv preprint arXiv:2105.14377 (2021).
https:/​/​doi.org/​10.48550/​arXiv.2105.14377
[73] Kosuke Mitarai, Makoto Negoro, Masahiro Kitagawa, and Keisuke Fujii. “Quantum circuit learning”. Physical Review A 98, 032309 (2018).
https:/​/​doi.org/​10.1103/​PhysRevA.98.032309
[74] Maria Schuld, Ville Bergholm, Christian Gogolin, Josh Izaac, and Nathan Killoran. “Evaluating analytic gradients on quantum hardware”. Physical Review A 99, 032331 (2019).
https:/​/​doi.org/​10.1103/​PhysRevA.99.032331
[75] John A Nelder and Roger Mead. “A simplex method for function minimization”. The computer journal 7, 308–313 (1965).
https:/​/​doi.org/​10.1093/​comjnl/​7.4.308
[76] M. J. D. Powell. “A direct search optimization method that models the objective and constraint functions by linear interpolation”. Advances in Optimization and Numerical Analysis (1994).
https:/​/​doi.org/​10.1007/​978-94-015-8330-5_4
[77] E. Campos, D. Rabinovich, V. Akshay, and J. Biamonte. “Training saturation in layerwise quantum approximate optimization”. Physical Review A 104 (2021).
https:/​/​doi.org/​10.1103/​PhysRevA.104.L030401
[78] Cheng Xue, Zhao-Yun Chen, Yu-Chun Wu, and Guo-Ping Guo. “Effects of quantum noise on quantum approximate optimization algorithm”. Chinese Physics Letters 38, 030302 (2021).
https:/​/​doi.org/​10.1088/​0256-307X/​38/​3/​030302
[79] Jeffrey Marshall, Filip Wudarski, Stuart Hadfield, and Tad Hogg. “Characterizing local noise in qaoa circuits”. IOP SciNotes 1, 025208 (2020). url: https:/​/​doi.org/​10.1088/​2633-1357/​abb0d7.
https:/​/​doi.org/​10.1088/​2633-1357/​abb0d7
[80] Enrico Fontana, M. Cerezo, Andrew Arrasmith, Ivan Rungger, and Patrick J. Coles. “Non-trivial symmetries in quantum landscapes and their resilience to quantum noise”. Quantum 6, 804 (2022).
https:/​/​doi.org/​10.22331/​q-2022-09-15-804
[81] Suguru Endo, Zhenyu Cai, Simon C Benjamin, and Xiao Yuan. “Hybrid quantum-classical algorithms and quantum error mitigation”. Journal of the Physical Society of Japan 90, 032001 (2021).
https:/​/​doi.org/​10.7566/​JPSJ.90.032001
[82] Angus Lowe, Max Hunter Gordon, Piotr Czarnik, Andrew Arrasmith, Patrick J. Coles, and Lukasz Cincio. “Unified approach to data-driven quantum error mitigation”. Phys. Rev. Research 3, 033098 (2021).
https:/​/​doi.org/​10.1103/​PhysRevResearch.3.033098
[83] Andrea Mari, Nathan Shammah, and William J Zeng. “Extending quantum probabilistic error cancellation by noise scaling”. Physical Review A 104, 052607 (2021).
https:/​/​doi.org/​10.1103/​PhysRevA.104.052607
[84] Daniel Bultrini, Max Hunter Gordon, Piotr Czarnik, Andrew Arrasmith, M. Cerezo, Patrick J. Coles, and Lukasz Cincio. “Unifying and benchmarking state-of-the-art quantum error mitigation techniques”. Quantum 7, 1034 (2023).
https:/​/​doi.org/​10.22331/​q-2023-06-06-1034
[85] Ashley Montanaro and Stasja Stanisic. “Error mitigation by training with fermionic linear optics”. arXiv preprint arXiv:2102.02120 (2021).
https:/​/​doi.org/​10.48550/​arXiv.2102.02120
[86] Joseph Vovrosh, Kiran E Khosla, Sean Greenaway, Christopher Self, Myungshik S Kim, and Johannes Knolle. “Simple mitigation of global depolarizing errors in quantum simulations”. Physical Review E 104, 035309 (2021).
https:/​/​doi.org/​10.1103/​PhysRevE.104.035309
[87] Eliott Rosenberg, Paul Ginsparg, and Peter L McMahon. “Experimental error mitigation using linear rescaling for variational quantum eigensolving with up to 20 qubits”. Quantum Science and Technology 7, 015024 (2022).
https:/​/​doi.org/​10.1088/​2058-9565/​ac3b37
[88] Andre He, Benjamin Nachman, Wibe A. de Jong, and Christian W. Bauer. “Zero-noise extrapolation for quantum-gate error mitigation with identity insertions”. Physical Review A 102, 012426 (2020).
https:/​/​doi.org/​10.1103/​PhysRevA.102.012426
[89] Andrew Shaw. “Classical-quantum noise mitigation for nisq hardware”. arXiv preprint arXiv:2105.08701 (2021).
https:/​/​doi.org/​10.48550/​arXiv.2105.08701
[90] Frank Arute, Kunal Arya, Ryan Babbush, Dave Bacon, Joseph C Bardin, Rami Barends, Andreas Bengtsson, Sergio Boixo, Michael Broughton, Bob B Buckley, et al. “Observation of separated dynamics of charge and spin in the fermi-hubbard model”. arXiv preprint arXiv:2010.07965 (2020).
https:/​/​doi.org/​10.48550/​arXiv.2010.07965
[91] Armands Strikis, Dayue Qin, Yanzhu Chen, Simon C Benjamin, and Ying Li. “Learning-based quantum error mitigation”. PRX Quantum 2, 040330 (2021).
https:/​/​doi.org/​10.1103/​PRXQuantum.2.040330
[92] Piotr Czarnik, Andrew Arrasmith, Lukasz Cincio, and Patrick J Coles. “Qubit-efficient exponential suppression of errors”. arXiv preprint arXiv:2102.06056 (2021).
https:/​/​doi.org/​10.48550/​arXiv.2102.06056
[93] Yifeng Xiong, Daryus Chandra, Soon Xin Ng, and Lajos Hanzo. “Sampling overhead analysis of quantum error mitigation: Uncoded vs. coded systems”. IEEE Access 8, 228967–228991 (2020).
https:/​/​doi.org/​10.1109/​ACCESS.2020.3045016
[94] Ryuji Takagi. “Optimal resource cost for error mitigation”. Phys. Rev. Res. 3, 033178 (2021).
https:/​/​doi.org/​10.1103/​PhysRevResearch.3.033178
[95] Lukasz Cincio, Kenneth Rudinger, Mohan Sarovar, and Patrick J. Coles. “Machine learning of noise-resilient quantum circuits”. PRX Quantum 2, 010324 (2021).
https:/​/​doi.org/​10.1103/​PRXQuantum.2.010324
[96] P Erdös and A Rényi. “On random graphs $I$”. Publicationes Mathematicae Debrecen 6, 18 (1959). url: http:/​/​snap.stanford.edu/​class/​cs224w-readings/​erdos59random.pdf.
http:/​/​snap.stanford.edu/​class/​cs224w-readings/​erdos59random.pdf
[97] Andrew Wack, Hanhee Paik, Ali Javadi-Abhari, Petar Jurcevic, Ismael Faro, Jay M. Gambetta, and Blake R. Johnson. “Quality, speed, and scale: three key attributes to measure the performance of near-term quantum computers”. arXiv preprint arXiv:2110.14108 (2021).
https:/​/​doi.org/​10.48550/​arXiv.2110.14108
[98] Tudor Giurgica-Tiron, Yousef Hindy, Ryan LaRose, Andrea Mari, and William J Zeng. “Digital zero noise extrapolation for quantum error mitigation”. 2020 IEEE International Conference on Quantum Computing and Engineering (QCE) (2020).
https:/​/​doi.org/​10.1109/​QCE49297.2020.00045
[99] Youngseok Kim, Christopher J. Wood, Theodore J. Yoder, Seth T. Merkel, Jay M. Gambetta, Kristan Temme, and Abhinav Kandala. “Scalable error mitigation for noisy quantum circuits produces competitive expectation values”. arXiv preprint arXiv:2108.09197 (2021).
https:/​/​doi.org/​10.1038/​s41567-022-01914-3
[100] Cristina Cirstoiu, Silas Dilkes, Daniel Mills, Seyon Sivarajah, and Ross Duncan. “Volumetric benchmarking of error mitigation with Qermit”. arXiv preprint arXiv:2204.09725 (2022).
https:/​/​doi.org/​10.48550/​ARXIV.2204.09725
[101] Ryuji Takagi, Suguru Endo, Shintaro Minagawa, and Mile Gu. “Fundamental limits of quantum error mitigation”. npj Quantum Information 8, 114 (2022).
https:/​/​doi.org/​10.1038/​s41534-022-00618-z
[102] Avram Sidi. “Practical extrapolation methods: Theory and applications”. Volume 10. Cambridge University Press. (2003).
[103] Masanori Ohya and Dénes Petz. “Quantum entropy and its use”. Springer Science & Business Media. (2004).
[104] Christoph Hirche, Cambyse Rouzé, and Daniel Stilck França. “On contraction coefficients, partial orders and approximation of capacities for quantum channels”. Quantum 6, 862 (2022).
https:/​/​doi.org/​10.22331/​q-2022-11-28-862
[105] Jeffrey C. Lagarias, James A. Reeds, Margaret H. Wright, and Paul E. Wright. “Convergence properties of the nelder–mead simplex method in low dimensions”. SIAM Journal on Optimization 9, 112–147 (1998).
https:/​/​doi.org/​10.1137/​S1052623496303470
[106] Abhijith J., Adetokunbo Adedoyin, John Ambrosiano, Petr Anisimov, William Casper, Gopinath Chennupati, Carleton Coffrin, Hristo Djidjev, David Gunter, Satish Karra, Nathan Lemons, Shizeng Lin, Alexander Malyzhenkov, David Mascarenas, Susan Mniszewski, Balu Nadiga, Daniel O’malley, Diane Oyen, Scott Pakin, Lakshman Prasad, Randy Roberts, Phillip Romero, Nandakishore Santhi, Nikolai Sinitsyn, Pieter J. Swart, James G. Wendelberger, Boram Yoon, Richard Zamora, Wei Zhu, Stephan Eidenbenz, Andreas Bärtschi, Patrick J. Coles, Marc Vuffray, and Andrey Y. Lokhov. “Quantum algorithm implementations for beginners”. ACM Transactions on Quantum Computing (2022).
https:/​/​doi.org/​10.1145/​3517340
[107] Bálint Koczor. “The dominant eigenvector of a noisy quantum state”. New Journal of Physics 23, 123047 (2021).
https:/​/​doi.org/​10.1088/​1367-2630/​ac37ae
Cited by
[1] Zhenyu Cai, Ryan Babbush, Simon C. Benjamin, Suguru Endo, William J. Huggins, Ying Li, Jarrod R. McClean, and Thomas E. O’Brien, “Quantum error mitigation”, Reviews of Modern Physics 95 4, 045005 (2023).
[2] Ryuji Takagi, Hiroyasu Tajima, and Mile Gu, “Universal Sampling Lower Bounds for Quantum Error Mitigation”, Physical Review Letters 131 21, 210602 (2023).
[3] Louis Schatzki, Andrew Arrasmith, Patrick J. Coles, and M. Cerezo, “Entangled Datasets for Quantum Machine Learning”, arXiv:2109.03400, (2021).
[4] Ryuji Takagi, Suguru Endo, Shintaro Minagawa, and Mile Gu, “Fundamental limits of quantum error mitigation”, npj Quantum Information 8, 114 (2022).
[5] Martin Larocca, Nathan Ju, Diego GarcÃa-MartÃn, Patrick J. Coles, and M. Cerezo, “Theory of overparametrization in quantum neural networks”, arXiv:2109.11676, (2021).
[6] Valentin Heyraud, Zejian Li, Kaelan Donatella, Alexandre Le Boité, and Cristiano Ciuti, “Efficient Estimation of Trainability for Variational Quantum Circuits”, PRX Quantum 4 4, 040335 (2023).
[7] Patrick J. Coles, Collin Szczepanski, Denis Melanson, Kaelan Donatella, Antonio J. Martinez, and Faris Sbahi, “Thermodynamic AI and the fluctuation frontier”, arXiv:2302.06584, (2023).
[8] Yihui Quek, Daniel Stilck França, Sumeet Khatri, Johannes Jakob Meyer, and Jens Eisert, “Exponentially tighter bounds on limitations of quantum error mitigation”, arXiv:2210.11505, (2022).
[9] Kento Tsubouchi, Takahiro Sagawa, and Nobuyuki Yoshioka, “Universal Cost Bound of Quantum Error Mitigation Based on Quantum Estimation Theory”, Physical Review Letters 131 21, 210601 (2023).
[10] R. Au-Yeung, B. Camino, O. Rathore, and V. Kendon, “Quantum algorithms for scientific applications”, arXiv:2312.14904, (2023).
[11] Yasunari Suzuki, Suguru Endo, Keisuke Fujii, and Yuuki Tokunaga, “Quantum error mitigation as a universal error-minimization technique: applications from NISQ to FTQC eras”, arXiv:2010.03887, (2020).
[12] Gokul Subramanian Ravi, Pranav Gokhale, Yi Ding, William M. Kirby, Kaitlin N. Smith, Jonathan M. Baker, Peter J. Love, Henry Hoffmann, Kenneth R. Brown, and Frederic T. Chong, “CAFQA: A classical simulation bootstrap for variational quantum algorithms”, arXiv:2202.12924, (2022).
[13] He-Liang Huang, Xiao-Yue Xu, Chu Guo, Guojing Tian, Shi-Jie Wei, Xiaoming Sun, Wan-Su Bao, and Gui-Lu Long, “Near-term quantum computing techniques: Variational quantum algorithms, error mitigation, circuit compilation, benchmarking and classical simulation”, Science China Physics, Mechanics, and Astronomy 66 5, 250302 (2023).
[14] Yasunari Suzuki, Suguru Endo, Keisuke Fujii, and Yuuki Tokunaga, “Quantum Error Mitigation as a Universal Error Reduction Technique: Applications from the NISQ to the Fault-Tolerant Quantum Computing Eras”, PRX Quantum 3 1, 010345 (2022).
[15] Supanut Thanasilp, Samson Wang, M. Cerezo, and Zoë Holmes, “Exponential concentration and untrainability in quantum kernel methods”, arXiv:2208.11060, (2022).
[16] Abhinav Deshpande, Pradeep Niroula, Oles Shtanko, Alexey V. Gorshkov, Bill Fefferman, and Michael J. Gullans, “Tight Bounds on the Convergence of Noisy Random Circuits to the Uniform Distribution”, PRX Quantum 3 4, 040329 (2022).
[17] Giacomo De Palma, Milad Marvian, Cambyse Rouzé, and Daniel Stilck França, “Limitations of Variational Quantum Algorithms: A Quantum Optimal Transport Approach”, PRX Quantum 4 1, 010309 (2023).
[18] Ingo Tews, Zohreh Davoudi, Andreas Ekström, Jason D. Holt, Kevin Becker, Raúl Briceño, David J. Dean, William Detmold, Christian Drischler, Thomas Duguet, Evgeny Epelbaum, Ashot Gasparyan, Jambul Gegelia, Jeremy R. Green, Harald W. Grießhammer, Andrew D. Hanlon, Matthias Heinz, Heiko Hergert, Martin Hoferichter, Marc Illa, David Kekejian, Alejandro Kievsky, Sebastian König, Hermann Krebs, Kristina D. Launey, Dean Lee, Petr Navrátil, Amy Nicholson, Assumpta Parreño, Daniel R. Phillips, Marek PÅ‚oszajczak, Xiu-Lei Ren, Thomas R. Richardson, Caroline Robin, Grigor H. Sargsyan, Martin J. Savage, Matthias R. Schindler, Phiala E. Shanahan, Roxanne P. Springer, Alexander Tichai, Ubirajara van Kolck, Michael L. Wagman, André Walker-Loud, Chieh-Jen Yang, and Xilin Zhang, “Nuclear Forces for Precision Nuclear Physics: A Collection of Perspectives”, Few-Body Systems 63 4, 67 (2022).
[19] C. Huerta Alderete, Max Hunter Gordon, Frédéric Sauvage, Akira Sone, Andrew T. Sornborger, Patrick J. Coles, and M. Cerezo, “Inference-Based Quantum Sensing”, Physical Review Letters 129 19, 190501 (2022).
[20] Frédéric Sauvage, MartÃn Larocca, Patrick J. Coles, and M. Cerezo, “Building spatial symmetries into parameterized quantum circuits for faster training”, Quantum Science and Technology 9 1, 015029 (2024).
[21] Adam Callison and Nicholas Chancellor, “Hybrid quantum-classical algorithms in the noisy intermediate-scale quantum era and beyond”, Physical Review A 106 1, 010101 (2022).
[22] Supanut Thanasilp, Samson Wang, Nhat A. Nghiem, Patrick J. Coles, and M. Cerezo, “Subtleties in the trainability of quantum machine learning models”, arXiv:2110.14753, (2021).
[23] Laurin E. Fischer, Daniel Miller, Francesco Tacchino, Panagiotis Kl. Barkoutsos, Daniel J. Egger, and Ivano Tavernelli, “Ancilla-free implementation of generalized measurements for qubits embedded in a qudit space”, Physical Review Research 4 3, 033027 (2022).
[24] Travis L. Scholten, Carl J. Williams, Dustin Moody, Michele Mosca, William Hurley, William J. Zeng, Matthias Troyer, and Jay M. Gambetta, “Assessing the Benefits and Risks of Quantum Computers”, arXiv:2401.16317, (2024).
[25] Benjamin A. Cordier, Nicolas P. D. Sawaya, Gian G. Guerreschi, and Shannon K. McWeeney, “Biology and medicine in the landscape of quantum advantages”, arXiv:2112.00760, (2021).
[26] Manuel S. Rudolph, Sacha Lerch, Supanut Thanasilp, Oriel Kiss, Sofia Vallecorsa, Michele Grossi, and Zoë Holmes, “Trainability barriers and opportunities in quantum generative modeling”, arXiv:2305.02881, (2023).
[27] Zhenyu Cai, “A Practical Framework for Quantum Error Mitigation”, arXiv:2110.05389, (2021).
[28] M. Cerezo, Guillaume Verdon, Hsin-Yuan Huang, Lukasz Cincio, and Patrick J. Coles, “Challenges and Opportunities in Quantum Machine Learning”, arXiv:2303.09491, (2023).
[29] Keita Kanno, Masaya Kohda, Ryosuke Imai, Sho Koh, Kosuke Mitarai, Wataru Mizukami, and Yuya O. Nakagawa, “Quantum-Selected Configuration Interaction: classical diagonalization of Hamiltonians in subspaces selected by quantum computers”, arXiv:2302.11320, (2023).
[30] Tailong Xiao, Xinliang Zhai, Xiaoyan Wu, Jianping Fan, and Guihua Zeng, “Practical advantage of quantum machine learning in ghost imaging”, Communications Physics 6 1, 171 (2023).
[31] Kazunobu Maruyoshi, Takuya Okuda, Juan W. Pedersen, Ryo Suzuki, Masahito Yamazaki, and Yutaka Yoshida, “Conserved charges in the quantum simulation of integrable spin chains”, Journal of Physics A Mathematical General 56 16, 165301 (2023).
[32] Marvin Bechtold, Johanna Barzen, Frank Leymann, Alexander Mandl, Julian Obst, Felix Truger, and Benjamin Weder, “Investigating the effect of circuit cutting in QAOA for the MaxCut problem on NISQ devices”, Quantum Science and Technology 8 4, 045022 (2023).
[33] Christoph Hirche, Cambyse Rouzé, and Daniel Stilck França, “On contraction coefficients, partial orders and approximation of capacities for quantum channels”, arXiv:2011.05949, (2020).
[34] Cristina Cirstoiu, Silas Dilkes, Daniel Mills, Seyon Sivarajah, and Ross Duncan, “Volumetric Benchmarking of Error Mitigation with Qermit”, Quantum 7, 1059 (2023).
[35] Minh C. Tran, Kunal Sharma, and Kristan Temme, “Locality and Error Mitigation of Quantum Circuits”, arXiv:2303.06496, (2023).
[36] Muhammad Kashif and Saif Al-Kuwari, “The impact of cost function globality and locality in hybrid quantum neural networks on NISQ devices”, Machine Learning: Science and Technology 4 1, 015004 (2023).
[37] Piotr Czarnik, Michael McKerns, Andrew T. Sornborger, and Lukasz Cincio, “Improving the efficiency of learning-based error mitigation”, arXiv:2204.07109, (2022).
[38] Daniel Bultrini, Samson Wang, Piotr Czarnik, Max Hunter Gordon, M. Cerezo, Patrick J. Coles, and Lukasz Cincio, “The battle of clean and dirty qubits in the era of partial error correction”, arXiv:2205.13454, (2022).
[39] Muhammad Kashif and Saif Al-kuwari, “ResQNets: A Residual Approach for Mitigating Barren Plateaus in Quantum Neural Networks”, arXiv:2305.03527, (2023).
[40] N. M. Guseynov, A. A. Zhukov, W. V. Pogosov, and A. V. Lebedev, “Depth analysis of variational quantum algorithms for the heat equation”, Physical Review A 107 5, 052422 (2023).
[41] Olivia Di Matteo and R. M. Woloshyn, “Quantum computing fidelity susceptibility using automatic differentiation”, Physical Review A 106 5, 052429 (2022).
[42] Matteo Robbiati, Alejandro Sopena, Andrea Papaluca, and Stefano Carrazza, “Real-time error mitigation for variational optimization on quantum hardware”, arXiv:2311.05680, (2023).
[43] Piotr Czarnik, Michael McKerns, Andrew T. Sornborger, and Lukasz Cincio, “Robust design under uncertainty in quantum error mitigation”, arXiv:2307.05302, (2023).
[44] Nico Meyer, Daniel D. Scherer, Axel Plinge, Christopher Mutschler, and Michael J. Hartmann, “Quantum Natural Policy Gradients: Towards Sample-Efficient Reinforcement Learning”, arXiv:2304.13571, (2023).
[45] Enrico Fontana, Ivan Rungger, Ross Duncan, and Cristina Cîrstoiu, “Spectral analysis for noise diagnostics and filter-based digital error mitigation”, arXiv:2206.08811, (2022).
[46] Wei-Bin Ewe, Dax Enshan Koh, Siong Thye Goh, Hong-Son Chu, and Ching Eng Png, “Variational Quantum-Based Simulation of Waveguide Modes”, IEEE Transactions on Microwave Theory Techniques 70 5, 2517 (2022).
[47] Zichang He, Bo Peng, Yuri Alexeev, and Zheng Zhang, “Distributionally Robust Variational Quantum Algorithms with Shifted Noise”, arXiv:2308.14935, (2023).
[48] Siddharth Dangwal, Gokul Subramanian Ravi, Poulami Das, Kaitlin N. Smith, Jonathan M. Baker, and Frederic T. Chong, “VarSaw: Application-tailored Measurement Error Mitigation for Variational Quantum Algorithms”, arXiv:2306.06027, (2023).
[49] Jessie M. Henderson, Marianna Podzorova, M. Cerezo, John K. Golden, Leonard Gleyzer, Hari S. Viswanathan, and Daniel O’Malley, “Quantum Algorithms for Geologic Fracture Networks”, arXiv:2210.11685, (2022).
[50] André Melo, Nathan Earnest-Noble, and Francesco Tacchino, “Pulse-efficient quantum machine learning”, Quantum 7, 1130 (2023).
[51] Christoph Hirche, Cambyse Rouzé, and Daniel Stilck França, “On contraction coefficients, partial orders and approximation of capacities for quantum channels”, Quantum 6, 862 (2022).
[52] Jessie M. Henderson, Marianna Podzorova, M. Cerezo, John K. Golden, Leonard Gleyzer, Hari S. Viswanathan, and Daniel O’Malley, “Quantum algorithms for geologic fracture networks”, Scientific Reports 13, 2906 (2023).
[53] Marco Schumann, Frank K. Wilhelm, and Alessandro Ciani, “Emergence of noise-induced barren plateaus in arbitrary layered noise models”, arXiv:2310.08405, (2023).
[54] Sharu Theresa Jose and Osvaldo Simeone, “Error Mitigation-Aided Optimization of Parameterized Quantum Circuits: Convergence Analysis”, arXiv:2209.11514, (2022).
[55] P. Singkanipa and D. A. Lidar, “Beyond unital noise in variational quantum algorithms: noise-induced barren plateaus and fixed points”, arXiv:2402.08721, (2024).
[56] Kevin Lively, Tim Bode, Jochen Szangolies, Jian-Xin Zhu, and Benedikt Fauseweh, “Robust Experimental Signatures of Phase Transitions in the Variational Quantum Eigensolver”, arXiv:2402.18953, (2024).
[57] Yunfei Wang and Junyu Liu, “Quantum Machine Learning: from NISQ to Fault Tolerance”, arXiv:2401.11351, (2024).
[58] Kosuke Ito and Keisuke Fujii, “SantaQlaus: A resource-efficient method to leverage quantum shot-noise for optimization of variational quantum algorithms”, arXiv:2312.15791, (2023).
The above citations are from SAO/NASA ADS (last updated successfully 2024-03-14 15:39:27). The list may be incomplete as not all publishers provide suitable and complete citation data.
Could not fetch Crossref cited-by data during last attempt 2024-03-14 15:39:25: Could not fetch cited-by data for 10.22331/q-2024-03-14-1287 from Crossref. This is normal if the DOI was registered recently.
This Paper is published in Quantum under the Creative Commons Attribution 4.0 International (CC BY 4.0) license. Copyright remains with the original copyright holders such as the authors or their institutions.
- SEO Powered Content & PR Distribution. Get Amplified Today.
- PlatoData.Network Vertical Generative Ai. Empower Yourself. Access Here.
- PlatoAiStream. Web3 Intelligence. Knowledge Amplified. Access Here.
- PlatoESG. Carbon, CleanTech, Energy, Environment, Solar, Waste Management. Access Here.
- PlatoHealth. Biotech and Clinical Trials Intelligence. Access Here.
- Source: https://quantum-journal.org/papers/q-2024-03-14-1287/
- :is
- :not
- :where
- ][p
- $UP
- 06
- 1
- 10
- 100
- 101
- 102
- 107
- 11
- 114
- 12
- 125
- 127
- 13
- 14
- 15%
- 16
- 17
- 19
- 1994
- 1998
- 2%
- 20
- 2009
- 2010
- 2011
- 2014
- 2016
- 2017
- 2018
- 2019
- 2020
- 2021
- 2022
- 2023
- 2024
- 21
- 22
- 2204
- 23
- 24
- 25
- 26
- 27
- 28
- 29
- 30
- 31
- 32
- 33
- 34
- 35%
- 36
- 39
- 4
- 40
- 41
- 42
- 45
- 46
- 48
- 49
- 5
- 50
- 51
- 52
- 54
- 55
- 58
- 6
- 60
- 62
- 65
- 66
- 67
- 7
- 70
- 72
- 73
- 74
- 75
- 77
- 8
- 80
- 804
- 84
- 87
- 89
- 9
- 90
- 91
- 97
- 98
- a
- above
- ABSTRACT
- access
- accurate
- ACM
- active
- Adam
- adaptive
- advanced
- advances
- ADvantage
- advantages
- adversarial
- affiliations
- AI
- Aid
- AL
- Alan
- alejandro
- alex
- Alexander
- algebra
- algorithm
- algorithms
- All
- allocation
- amy
- an
- analysis
- Analytic
- Analytical
- and
- andre
- andrea
- Andrew
- applications
- applied
- Applying
- approach
- approximate
- arbitrary
- ARE
- arya
- AS
- ask
- Assessing
- assisted
- astronomy
- At
- attempt
- attributes
- author
- authors
- Automatic
- averaging
- b
- baker
- barren
- barriers
- based
- Battle
- BE
- been
- beer
- Beginners
- benchmarking
- benefits
- Benjamin
- BEST
- Beyond
- Bill
- BIN
- biology
- Bo
- bob
- Bootstrap
- both
- bound
- bounds
- Break
- broad
- brown
- Bryan
- Building
- bulletin
- business
- by
- cambridge
- camino
- CAN
- cannot
- cao
- capacities
- care
- Carl
- carlos
- caroline
- cases
- casper
- Center
- certain
- chains
- challenges
- channels
- charge
- charges
- chemistry
- chen
- Cheng
- China
- chinese
- chong
- chow
- Chris
- christian
- Christopher
- circuit
- circuits
- class
- clean
- coded
- coefficients
- collection
- College
- comment
- committing
- Commons
- Communications
- compared
- competitive
- complete
- complex
- computational
- computer
- computer science
- computers
- computing
- concentration
- Conference
- Configuration
- consistent
- constraint
- continuous
- contraction
- control
- Convergence
- copyright
- correlated
- Cost
- could
- Crooks
- Cut
- cutting
- Daniel
- data
- data-driven
- datasets
- Dave
- David
- de
- deep
- Den
- dependent
- depth
- Derivatives
- Design
- determination
- device
- Devices
- Diagnostics
- Diane
- Diego
- digital
- dimensions
- direct
- discovering
- discuss
- distribution
- distributions
- Division
- do
- dominant
- duncan
- during
- dynamics
- e
- E&T
- Edward
- Edwin
- effect
- efficiency
- efficient
- either
- elsewhere
- embedded
- emergence
- energy
- Engineering
- equation
- Era
- eric
- error
- Errors
- Ether (ETH)
- evidence
- evolution
- exact
- excited
- expectation
- experimental
- exponential
- exponentially
- extends
- factoring
- fan
- FAST
- faster
- fault
- fidelity
- Find
- First
- fixed
- flattening
- fluctuation
- For
- Forces
- found
- Foundations
- fracture
- Framework
- frank
- from
- Frontier
- function
- functions
- fundamental
- GAO
- gate
- gavin
- General
- generalized
- generative
- generators
- George
- Ghost
- Global
- Golden
- gordon
- gradients
- grant
- graphs
- Green
- hand
- harder
- Hardware
- harvard
- Have
- he
- henry
- hierarchy
- High
- High Fidelity
- Highlight
- histories
- holders
- hope
- However
- http
- HTTPS
- huang
- hunter
- Hybrid
- hybrid quantum-classical
- Identity
- IEEE
- if
- image
- imaginary
- Imaging
- Impact
- Imperial
- Imperial College
- Imperial College London
- implementation
- implementations
- improve
- improving
- in
- includes
- incorporating
- information
- institutions
- interaction
- interesting
- International
- interpolation
- into
- investigating
- iris
- Israel
- IT
- ITS
- ivan
- jack
- Jack Hidary
- jacob
- james
- Japan
- JavaScript
- jeffrey
- jeremy
- joe
- John
- Johnson
- jonathan
- jones
- joshua
- journal
- juan
- kenneth
- Key
- khosla
- Kim
- kirby
- kiss
- König
- laboratory
- landscape
- landscapes
- large
- Last
- Lau
- layered
- learning
- Leave
- Lee
- leonard
- Leverage
- li
- License
- lidar
- LIMIT
- limitations
- limits
- lin
- linear
- List
- local
- London
- Long
- los
- Los Alamos National Laboratory
- Louis
- love
- Low
- lower
- machine
- machine learning
- make
- manuel
- mar
- Marco
- maria
- Martin
- materials
- mathematical
- matthew
- matthias
- max
- max-width
- maximum
- May..
- mcclean
- measure
- measurement
- measurements
- mechanics
- Media
- medicine
- method
- methods
- Metrology
- Meyer
- Michael
- mikhail
- mile
- Miller
- mills
- minimization
- Mitigate
- mitigating
- mitigation
- model
- modeling
- models
- Modern
- modes
- molecular
- Month
- muhammad
- nathan
- National
- Natural
- Nature
- Near
- network
- networks
- Neural
- neural network
- neural networks
- New
- nicholas
- Nicolas
- no
- Noise
- nonlinear
- normal
- nuclear
- Nuclear physics
- numerical
- oak
- objective
- of
- often
- on
- open
- operator
- opportunities
- optics
- optimal
- optimization
- or
- order
- orders
- original
- Other
- our
- overhead
- pages
- Paper
- parametrized
- partial
- patrick
- Paul
- perform
- performance
- perspectives
- Peter
- phase
- phenomenon
- physical
- Physics
- plato
- Plato Data Intelligence
- PlatoData
- Point
- Point of View
- points
- policy
- positive
- possibility
- Powell
- Practical
- Pradeep
- Precision
- press
- Problem
- problems
- process
- processes
- Processor
- processors
- produces
- promise
- properties
- protocols
- provide
- published
- publisher
- publishers
- Qi
- Quantum
- quantum advantage
- quantum algorithms
- Quantum Computer
- quantum computers
- quantum computing
- quantum error correction
- quantum gate
- quantum information
- quantum machine learning
- quantum technology
- qubits
- R
- RAMI
- random
- reach
- readout
- real-time
- recent
- recently
- reducing
- reduction
- references
- registered
- regression
- reinforcement learning
- reliable
- remains
- ren
- Reports
- research
- resilience
- resilient
- resolve
- resolved
- resource
- Resources
- result
- Results
- review
- Reviews
- Richard
- risks
- ROBERT
- Robin
- robust
- running
- Ryan
- s
- Sam
- sample
- Scale
- scaling
- Science
- Science and Technology
- scientific
- scott
- Sean
- Search
- Second
- selected
- SELF
- settings
- severe
- severely
- shallow
- shannon
- Sharma
- shaw
- shifted
- shot
- should
- show
- shown
- Shows
- siam
- Signatures
- Simon
- Simplex
- simulation
- simulations
- simulator
- sizes
- smith
- Society
- some
- Soon
- sophia
- Space
- Spatial
- special
- Spectral
- speed
- Spin
- stanford
- State
- state-of-the-art
- States
- stefan
- strategies
- studies
- Successfully
- such
- suitable
- Sun
- suppressing
- suppression
- Susan
- susceptibility
- symmetry
- system
- Systems
- T
- taken
- taylor
- technique
- techniques
- Technologies
- Technology
- term
- that
- The
- The Landscape
- their
- Them
- theory
- These
- they
- this
- thomas
- three
- Thus
- tighter
- Tim
- time
- Title
- to
- tolerance
- too
- Toolbox
- tools
- towards
- Train
- Training
- Transactions
- transitions
- transport
- tyler
- types
- Uncertainty
- under
- Universal
- university
- updated
- URL
- use
- using
- Values
- van
- Verification
- verified
- via
- View
- viewed
- Virtual
- volume
- volumetric
- vs
- W
- wang
- want
- was
- we
- whether
- william
- Williams
- with
- without
- Wolf
- wood
- Work
- worsen
- Wright
- wu
- X
- xiao
- year
- YING
- Yuan
- zephyrnet
- zero
- zhang
- Zhao
- Zhong