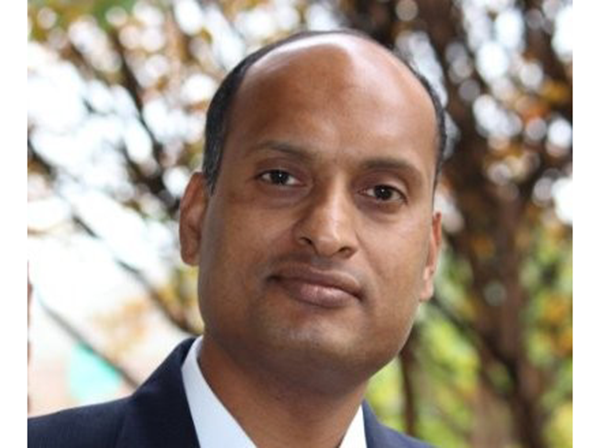
Most enterprises suffer from spotty deployment and management of artificial intelligence (AI) initiatives. As different parts of the organization experiment with AI in silos, they waste both resources and the opportunity to learn from the experience of others. When my company commissioned an independent third-party survey of more than 2,500 AI practitioners across industries and geographies, it found that organizations have invested big sums in AI but only acquired basic capabilities; as many as three out of four respondents said they were still looking to scale AI across the enterprise.
Until that happens, the real benefits of AI will remain elusive; on the flip side, even small investments in AI initiatives that find scale earn disproportionately high returns. One estimate says that organizations that embed AI in business processes, workflows, and customer experiences could increase their profitability by as much as 20%.
According to observers, the companies that have scaled AI owe their success to certain good practices such as:
Prioritizing and scaling the right initiatives to accelerate value: Typically, AI leaders scale many more use cases – and much faster – than other firms. Focusing on building AI-powered business capabilities that power multiple use cases serving key business priorities, making sure new AI capabilities are quickly available to those who need them, and leveraging proven execution models along with agile development enable them to scale more than two out of five use cases, where others manage to scale just one (in five).
Establishing structure and governance to keep programs on track: A majority of companies that have scaled AI have well-defined strategies and processes, a good operating model, and a robust governance framework for pinning responsibility and accountability on the right owners.
Organizations must also build trust in AI before they can scale adoption among employees and customers; AI that is not transparent, explained, or understood is likely to disappoint, breed doubt, or remain unused. Often, organizations prioritize based on business value and ease of implementation. For AI, however, the trustworthiness of the solution is the most crucial element driving adoption and success. To produce reliable, trustworthy, and fair outputs, it is necessary to feed AI models the right kind of training data – that which is clean and accurate, but also ethical and free of bias.
Supporting programs with leadership and talent: The backing of leaders, and the availability of strong skills, are huge factors of success in scaling AI. Typically, organizations where more than 10% of technical staff work on AI and more than 30% of staff use AI every day, achieve much greater profitability improvements than other firms. Their leadership provides crucial support, including prioritizing the right use cases and defining the roadmap to scale.
Besides technical staff and senior executives, people with business expertise – those who are closest to the business problem being solved – should be part of the AI team; their knowledge is critical to devising the right AI models and the data to go with it. They also become key champions in evangelizing and driving the adoption of AI within business communities. Just as senior leaders ensure AI is aligned with strategic objectives, business experts make sure it is tethered to business priorities.
Being data-ready and being savvy about using data: AI leaders know which data to pick – that which is critical to their business – from the unlimited sources that are out there, and how to manage it efficiently. Making data available, accessible, and trustworthy for AI use enables scaled implementations of AI. While AI leaders typically handle more data by volume and variety than other companies, they also manage to achieve higher data quality. Using various digital tools and technologies – cloud, data science, and analytics – they are able to optimize their data and take AI initiatives to success.
To scale AI, organizations need to share data across the enterprise; however, they also need to have some amount of control over it. This puts them in a dilemma about which data management strategy to pursue: centralized or federated. But the answer is not so simple. The view of industry experts is that while centralized data management can improve profits, so can a fully federated data management strategy. A combination “hub and spoke” strategy, where the organization centralizes the platform and technology but allows the teams operational autonomy, seems to offer the best of both worlds.
In Closing: AI and the Data Economy
Data gives life to AI. But AI can return the favor by mitigating two key challenges confronting the data economy: silos, and lack of transparency in how personal data is used. AI tools draw on disparate information sources across an enterprise (or ecosystem), structure and format data so it is usable, and counteract silos by making the data visible, and its insights available, to all parts of the organization. This sets off a virtuous cycle where the breaking down of silos improves the performance of AI, which helps to lower the barriers between the organization’s data even further. With scale, this cycle becomes bigger and better. Hence, for enterprises, the way forward is to prioritize the right AI initiatives, become data-ready for AI, build governance and trust, provide leadership and talent, and adopt good data practices.
- SEO Powered Content & PR Distribution. Get Amplified Today.
- PlatoData.Network Vertical Generative Ai. Empower Yourself. Access Here.
- PlatoAiStream. Web3 Intelligence. Knowledge Amplified. Access Here.
- PlatoESG. Carbon, CleanTech, Energy, Environment, Solar, Waste Management. Access Here.
- PlatoHealth. Biotech and Clinical Trials Intelligence. Access Here.
- Source: https://www.dataversity.net/dont-dawdle-dont-dabble-scale-ai-now/
- :is
- :not
- :where
- 500
- a
- Able
- About
- accelerate
- accessible
- accountability
- accurate
- Achieve
- acquired
- across
- adopt
- Adoption
- agile
- AI
- AI in Business
- AI models
- AI-powered
- aligned
- All
- allows
- along
- also
- among
- amount
- an
- analytics
- and
- answer
- ARE
- artificial
- artificial intelligence
- Artificial intelligence (AI)
- AS
- Autonomy
- availability
- available
- backing
- barriers
- based
- basic
- BCG
- BE
- become
- becomes
- before
- being
- benefits
- BEST
- Better
- between
- Big
- bigger
- both
- Breaking
- BREED
- build
- build trust
- Building
- business
- business processes
- but
- by
- CAN
- capabilities
- cases
- centralized
- certain
- challenges
- Champions
- clean
- closest
- closing
- Cloud
- combination
- Communities
- Companies
- company
- control
- could
- counteract
- critical
- crucial
- customer
- Customers
- cycle
- data
- data management
- data quality
- data science
- DATAVERSITY
- day
- defining
- deployment
- Development
- different
- digital
- disparate
- Dont
- doubt
- down
- draw
- driving
- earn
- ease
- economy
- ecosystem
- efficiently
- element
- embed
- employees
- enable
- enables
- ensure
- Enterprise
- enterprises
- estimate
- Even
- Every
- every day
- execution
- executives
- experience
- Experiences
- experiment
- expertise
- experts
- explained
- factors
- fair
- faster
- favor
- Find
- firms
- five
- Flip
- focusing
- For
- format
- Forward
- found
- four
- Framework
- Free
- from
- fully
- further
- geographies
- gives
- Go
- good
- governance
- greater
- handle
- happens
- Have
- helps
- hence
- High
- higher
- How
- How To
- However
- HTTPS
- huge
- implementation
- implementations
- improve
- improvements
- improves
- in
- Including
- Increase
- independent
- industries
- industry
- industry experts
- information
- Infosys
- initiatives
- insights
- Intelligence
- invested
- IT
- ITS
- just
- Keep
- Key
- Kind
- Know
- knowledge
- Lack
- leaders
- Leadership
- LEARN
- leveraging
- Life
- likely
- looking
- lower
- Majority
- make
- Making
- manage
- management
- many
- McKinsey
- mitigating
- model
- models
- more
- most
- much
- multiple
- must
- my
- necessary
- Need
- New
- now
- objectives
- observers
- of
- off
- offer
- often
- on
- ONE
- only
- operating
- operational
- Opportunity
- Optimize
- or
- organization
- organizations
- Other
- Others
- out
- outputs
- over
- owners
- part
- parts
- People
- performance
- personal
- personal data
- pick
- platform
- plato
- Plato Data Intelligence
- PlatoData
- power
- practices
- Prioritize
- prioritizing
- Problem
- processes
- produce
- profitability
- profits
- Programs
- proven
- provide
- provides
- pursue
- Puts
- quality
- quickly
- real
- reliable
- remain
- Resources
- respondents
- responsibility
- return
- returns
- right
- roadmap
- robust
- Said
- savvy
- says
- Scale
- scale ai
- scaling
- Science
- seems
- senior
- serving
- Sets
- Share
- should
- side
- silos
- Simple
- skills
- So
- solution
- some
- Sources
- Staff
- Still
- Strategic
- strategies
- Strategy
- strong
- structure
- success
- such
- sums
- support
- sure
- Survey
- Take
- Talent
- team
- teams
- Technical
- Technologies
- Technology
- than
- that
- The
- their
- Them
- There.
- they
- third-party
- this
- those
- three
- to
- tools
- track
- Training
- Transparency
- transparent
- Trust
- trustworthiness
- trustworthy
- two
- typically
- understood
- unlimited
- unused
- usable
- use
- used
- using
- value
- variety
- various
- View
- visible
- volume
- Waste
- Way..
- well-defined
- were
- when
- which
- while
- WHO
- will
- with
- within
- Work
- workflows
- world’s
- zephyrnet