Key Laboratory for Information Science of Electromagnetic Waves (Ministry of Education), Fudan University, Shanghai 200433, China
Find this paper interesting or want to discuss? Scite or leave a comment on SciRate.
Abstract
Shadow estimation is an efficient method for predicting many observables of a quantum state with a statistical guarantee. In the multi-shot scenario, one performs projective measurement on the sequentially prepared state for $K$ times after the same unitary evolution, and repeats this procedure for $M$ rounds of random sampled unitary. As a result, there are $MK$ times measurements in total. Here we analyze the performance of shadow estimation in this multi-shot scenario, which is characterized by the variance of estimating the expectation value of some observable $O$. We find that in addition to the shadow-norm $|O |_{mathrm{shadow}}$ introduced in [1], the variance is also related to another norm, and we denote it as the cross-shadow-norm $|O |_{mathrm{Xshadow}}$. For both random Pauli and Clifford measurements, we analyze and show the upper bounds of $|O |_{mathrm{Xshadow}}$. In particular, we figure out the exact variance formula for Pauli observable under random Pauli measurements. Our work gives theoretical guidance for the application of multi-shot shadow estimation.
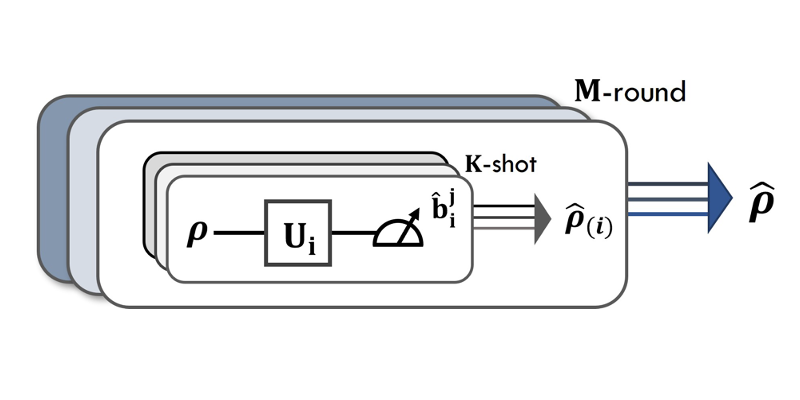
Featured image: Multi-shot shadow estimation: To compensate for the cost of the realization of random unitary evolution, one can conduct projective measurements on the sequentially prepared state for K times under the same unitary evolution, and this procedure is repeated for M rounds of randomly sampled unitary. The current work systematically analyzes the statistical performance of shadow estimation in this multi-shot scenario, as a supplement to the original shadow estimation [Huang et.al. Nat. Phys. 2020] (K=1).
Popular summary
Shadow estimation is an efficient approach to this task via randomized measurements. To compensate for the cost of the realization of random unitary evolution, one can conduct multi-shot shadow estimation, where projective measurements are performed on the sequentially prepared state for many times under the $same$ unitary evolution, and this procedure is repeated for a few rounds of randomly sampled unitary.
The current work systematically analyzes the statistical performance of shadow estimation in this multi-shot scenario. We introduce a key quantity——the cross-shadow-norm, which supplements the original shadow-norm. For both random Pauli and Clifford measurement settings, we analyze and give the estimation of the cross-shadow-norm. In particular, we figure out the exact variance for Pauli observables under random Pauli measurements and find the advantage of this multi-shot framework. In the meantime, we indicate, with the help of numerical simulation, that the advantage of multi-shot in Clifford measurements is not significant.
Our work gives theoretical guidance for multi-shot shadow estimation, which could be further applied to various quantum learning tasks, from quantum chemistry to quantum many-body physics.
â–º BibTeX data
â–º References
[1] Hsin-Yuan Huang, Richard Kueng, and John Preskill. Predicting many properties of a quantum system from very few measurements. Nature Physics, 16 (10): 1050–1057, 2020. 10.1038/​s41567-020-0932-7.
https:/​/​doi.org/​10.1038/​s41567-020-0932-7
[2] Jeongwan Haah, Aram W Harrow, Zhengfeng Ji, Xiaodi Wu, and Nengkun Yu. Sample-optimal tomography of quantum states. IEEE Transactions on Information Theory, 63 (9): 5628–5641, 2017. 10.1109/​TIT.2017.2719044.
https:/​/​doi.org/​10.1109/​TIT.2017.2719044
[3] Steven T Flammia, David Gross, Yi-Kai Liu, and Jens Eisert. Quantum tomography via compressed sensing: error bounds, sample complexity and efficient estimators. New Journal of Physics, 14 (9): 095022, 2012. 10.1088/​1367-2630/​14/​9/​095022.
https:/​/​doi.org/​10.1088/​1367-2630/​14/​9/​095022
[4] Martin Kliesch and Ingo Roth. Theory of quantum system certification. PRX Quantum, 2 (1): 010201, 2021. 10.1103/​PRXQuantum.2.010201.
https:/​/​doi.org/​10.1103/​PRXQuantum.2.010201
[5] Ehud Altman, Kenneth R Brown, Giuseppe Carleo, Lincoln D Carr, Eugene Demler, Cheng Chin, Brian DeMarco, Sophia E Economou, Mark A Eriksson, Kai-Mei C Fu, et al. Quantum simulators: Architectures and opportunities. PRX Quantum, 2 (1): 017003, 2021. 10.1103/​PRXQuantum.2.017003.
https:/​/​doi.org/​10.1103/​PRXQuantum.2.017003
[6] Yuri Alexeev, Dave Bacon, Kenneth R Brown, Robert Calderbank, Lincoln D Carr, Frederic T Chong, Brian DeMarco, Dirk Englund, Edward Farhi, Bill Fefferman, et al. Quantum computer systems for scientific discovery. PRX Quantum, 2 (1): 017001, 2021. 10.1103/​PRXQuantum.2.017001.
https:/​/​doi.org/​10.1103/​PRXQuantum.2.017001
[7] Andreas Elben, Steven T Flammia, Hsin-Yuan Huang, Richard Kueng, John Preskill, Benoı̂t Vermersch, and Peter Zoller. The randomized measurement toolbox. Nature Reviews Physics, 5 (1): 9–24, 2023. 10.1038/​s42254-022-00535-2.
https:/​/​doi.org/​10.1038/​s42254-022-00535-2
[8] Scott Aaronson. Shadow tomography of quantum states. SIAM Journal on Computing, 49 (5): STOC18–368, 2019. 10.1137/​18M120275X.
https:/​/​doi.org/​10.1137/​18M120275X
[9] Andreas Elben, Richard Kueng, Hsin-Yuan Robert Huang, Rick van Bijnen, Christian Kokail, Marcello Dalmonte, Pasquale Calabrese, Barbara Kraus, John Preskill, Peter Zoller, et al. Mixed-state entanglement from local randomized measurements. Physical Review Letters, 125 (20): 200501, 2020. 10.1103/​PhysRevLett.125.200501.
https:/​/​doi.org/​10.1103/​PhysRevLett.125.200501
[10] Aniket Rath, Cyril Branciard, Anna Minguzzi, and Benoı̂t Vermersch. Quantum fisher information from randomized measurements. Phys. Rev. Lett., 127: 260501, Dec 2021. 10.1103/​PhysRevLett.127.260501.
https:/​/​doi.org/​10.1103/​PhysRevLett.127.260501
[11] Zhenhuan Liu, Yifan Tang, Hao Dai, Pengyu Liu, Shu Chen, and Xiongfeng Ma. Detecting entanglement in quantum many-body systems via permutation moments. Physical Review Letters, 129 (26): 260501, 2022a. 10.1103/​PhysRevLett.129.260501.
https:/​/​doi.org/​10.1103/​PhysRevLett.129.260501
[12] Roy J. Garcia, You Zhou, and Arthur Jaffe. Quantum scrambling with classical shadows. Phys. Rev. Research, 3: 033155, Aug 2021. 10.1103/​PhysRevResearch.3.033155.
https:/​/​doi.org/​10.1103/​PhysRevResearch.3.033155
[13] Max McGinley, Sebastian Leontica, Samuel J Garratt, Jovan Jovanovic, and Steven H Simon. Quantifying information scrambling via classical shadow tomography on programmable quantum simulators. Physical Review A, 106 (1): 012441, 2022. 10.1103/​PhysRevA.106.012441.
https:/​/​doi.org/​10.1103/​PhysRevA.106.012441
[14] Alireza Seif, Ze-Pei Cian, Sisi Zhou, Senrui Chen, and Liang Jiang. Shadow distillation: Quantum error mitigation with classical shadows for near-term quantum processors. PRX Quantum, 4 (1): 010303, 2023. 10.1103/​PRXQuantum.4.010303.
https:/​/​doi.org/​10.1103/​PRXQuantum.4.010303
[15] Hong-Ye Hu, Ryan LaRose, Yi-Zhuang You, Eleanor Rieffel, and Zhihui Wang. Logical shadow tomography: Efficient estimation of error-mitigated observables. arXiv preprint arXiv:2203.07263, 2022. 10.48550/​arXiv.2203.07263.
https:/​/​doi.org/​10.48550/​arXiv.2203.07263
arXiv:2203.07263
[16] Hsin-Yuan Huang, Michael Broughton, Jordan Cotler, Sitan Chen, Jerry Li, Masoud Mohseni, Hartmut Neven, Ryan Babbush, Richard Kueng, John Preskill, et al. Quantum advantage in learning from experiments. Science, 376 (6598): 1182–1186, 2022a. 10.1126/​science.abn7293.
https:/​/​doi.org/​10.1126/​science.abn7293
[17] Hsin-Yuan Huang, Richard Kueng, Giacomo Torlai, Victor V Albert, and John Preskill. Provably efficient machine learning for quantum many-body problems. Science, 377 (6613): eabk3333, 2022b. 10.1126/​science.abk3333.
https:/​/​doi.org/​10.1126/​science.abk3333
[18] Stefan H. Sack, Raimel A. Medina, Alexios A. Michailidis, Richard Kueng, and Maksym Serbyn. Avoiding barren plateaus using classical shadows. PRX Quantum, 3: 020365, Jun 2022. 10.1103/​PRXQuantum.3.020365.
https:/​/​doi.org/​10.1103/​PRXQuantum.3.020365
[19] Hsin-Yuan Huang, Richard Kueng, and John Preskill. Efficient estimation of pauli observables by derandomization. Physical review letters, 127 (3): 030503, 2021. 10.1103/​PhysRevLett.127.030503.
https:/​/​doi.org/​10.1103/​PhysRevLett.127.030503
[20] Charles Hadfield, Sergey Bravyi, Rudy Raymond, and Antonio Mezzacapo. Measurements of quantum hamiltonians with locally-biased classical shadows. Communications in Mathematical Physics, 391 (3): 951–967, 2022. 10.1007/​s00220-022-04343-8.
https:/​/​doi.org/​10.1007/​s00220-022-04343-8
[21] Bujiao Wu, Jinzhao Sun, Qi Huang, and Xiao Yuan. Overlapped grouping measurement: A unified framework for measuring quantum states. Quantum, 7: 896, 2023. 10.22331/​q-2023-01-13-896.
https:/​/​doi.org/​10.22331/​q-2023-01-13-896
[22] You Zhou and Zhenhuan Liu. A hybrid framework for estimating nonlinear functions of quantum states. arXiv preprint arXiv:2208.08416, 2022. 10.48550/​arXiv.2208.08416.
https:/​/​doi.org/​10.48550/​arXiv.2208.08416
arXiv:2208.08416
[23] Roman Stricker, Michael Meth, Lukas Postler, Claire Edmunds, Chris Ferrie, Rainer Blatt, Philipp Schindler, Thomas Monz, Richard Kueng, and Martin Ringbauer. Experimental single-setting quantum state tomography. PRX Quantum, 3: 040310, Oct 2022. 10.1103/​PRXQuantum.3.040310.
https:/​/​doi.org/​10.1103/​PRXQuantum.3.040310
[24] H. Chau Nguyen, Jan Lennart Bönsel, Jonathan Steinberg, and Otfried Gühne. Optimizing shadow tomography with generalized measurements. Phys. Rev. Lett., 129: 220502, Nov 2022. 10.1103/​PhysRevLett.129.220502.
https:/​/​doi.org/​10.1103/​PhysRevLett.129.220502
[25] Hong-Ye Hu, Soonwon Choi, and Yi-Zhuang You. Classical shadow tomography with locally scrambled quantum dynamics. Physical Review Research, 5 (2): 023027, 2023. 10.1103/​PhysRevResearch.5.023027.
https:/​/​doi.org/​10.1103/​PhysRevResearch.5.023027
[26] Christian Bertoni, Jonas Haferkamp, Marcel Hinsche, Marios Ioannou, Jens Eisert, and Hakop Pashayan. Shallow shadows: Expectation estimation using low-depth random clifford circuits. arXiv preprint arXiv:2209.12924, 2022. 10.48550/​arXiv.2209.12924.
https:/​/​doi.org/​10.48550/​arXiv.2209.12924
arXiv:2209.12924
[27] Mirko Arienzo, Markus Heinrich, Ingo Roth, and Martin Kliesch. Closed-form analytic expressions for shadow estimation with brickwork circuits. arXiv preprint arXiv:2211.09835, 2022. 10.48550/​arXiv.2211.09835.
https:/​/​doi.org/​10.48550/​arXiv.2211.09835
arXiv:2211.09835
[28] Hong-Ye Hu and Yi-Zhuang You. Hamiltonian-driven shadow tomography of quantum states. Phys. Rev. Research, 4: 013054, Jan 2022. 10.1103/​PhysRevResearch.4.013054.
https:/​/​doi.org/​10.1103/​PhysRevResearch.4.013054
[29] Minh C Tran, Daniel K Mark, Wen Wei Ho, and Soonwon Choi. Measuring arbitrary physical properties in analog quantum simulation. Physical Review X, 13 (1): 011049, 2023. 10.1103/​PhysRevX.13.011049.
https:/​/​doi.org/​10.1103/​PhysRevX.13.011049
[30] Max McGinley and Michele Fava. Shadow tomography from emergent state designs in analog quantum simulators. arXiv preprint arXiv:2212.02543, 2022. 10.48550/​arXiv.2212.02543.
https:/​/​doi.org/​10.48550/​arXiv.2212.02543
arXiv:2212.02543
[31] Katherine Van Kirk, Jordan Cotler, Hsin-Yuan Huang, and Mikhail D Lukin. Hardware-efficient learning of quantum many-body states. arXiv preprint arXiv:2212.06084, 2022. 10.48550/​arXiv.2212.06084.
https:/​/​doi.org/​10.48550/​arXiv.2212.06084
arXiv:2212.06084
[32] Senrui Chen, Wenjun Yu, Pei Zeng, and Steven T. Flammia. Robust shadow estimation. PRX Quantum, 2: 030348, Sep 2021. 10.1103/​PRXQuantum.2.030348.
https:/​/​doi.org/​10.1103/​PRXQuantum.2.030348
[33] Dax Enshan Koh and Sabee Grewal. Classical shadows with noise. Quantum, 6: 776, 2022. 10.22331/​q-2022-08-16-776.
https:/​/​doi.org/​10.22331/​q-2022-08-16-776
[34] Matthias Ohliger, Vincent Nesme, and Jens Eisert. Efficient and feasible state tomography of quantum many-body systems. New Journal of Physics, 15 (1): 015024, 2013. 10.1088/​1367-2630/​15/​1/​015024.
https:/​/​doi.org/​10.1088/​1367-2630/​15/​1/​015024
[35] Ahmed A Akhtar, Hong-Ye Hu, and Yi-Zhuang You. Scalable and flexible classical shadow tomography with tensor networks. Quantum, 7: 1026, 2023. 10.22331/​q-2023-06-01-1026.
https:/​/​doi.org/​10.22331/​q-2023-06-01-1026
[36] Huangjun Zhu, Richard Kueng, Markus Grassl, and David Gross. The clifford group fails gracefully to be a unitary 4-design. arXiv:1609.08172, 2016. 10.48550/​arXiv.1609.08172.
https:/​/​doi.org/​10.48550/​arXiv.1609.08172
arXiv:1609.08172
[37] David Gross, Sepehr Nezami, and Michael Walter. Schur–weyl duality for the clifford group with applications: Property testing, a robust hudson theorem, and de finetti representations. Communications in Mathematical Physics, 385 (3): 1325–1393, 2021. 10.1007/​s00220-021-04118-7.
https:/​/​doi.org/​10.1007/​s00220-021-04118-7
[38] Sam McArdle, Suguru Endo, Alán Aspuru-Guzik, Simon C. Benjamin, and Xiao Yuan. Quantum computational chemistry. Rev. Mod. Phys., 92: 015003, Mar 2020. 10.1103/​RevModPhys.92.015003.
https:/​/​doi.org/​10.1103/​RevModPhys.92.015003
[39] Srilekha Gandhari, Victor V Albert, Thomas Gerrits, Jacob M Taylor, and Michael J Gullans. Continuous-variable shadow tomography. arXiv preprint arXiv:2211.05149, 2022. 10.48550/​arXiv.2211.05149.
https:/​/​doi.org/​10.48550/​arXiv.2211.05149
arXiv:2211.05149
[40] Simon Becker, Nilanjana Datta, Ludovico Lami, and Cambyse Rouzé. Classical shadow tomography for continuous variables quantum systems. arXiv preprint arXiv:2211.07578, 2022. 10.48550/​arXiv.2211.07578.
https:/​/​doi.org/​10.48550/​arXiv.2211.07578
arXiv:2211.07578
[41] Tianren Gu, Xiao Yuan, and Bujiao Wu. Efficient measurement schemes for bosonic systems. arXiv preprint arXiv:2210.13585, 2022. 10.48550/​arXiv.2210.13585.
https:/​/​doi.org/​10.48550/​arXiv.2210.13585
arXiv:2210.13585
[42] Andrew Zhao, Nicholas C. Rubin, and Akimasa Miyake. Fermionic partial tomography via classical shadows. Phys. Rev. Lett., 127: 110504, Sep 2021. 10.1103/​PhysRevLett.127.110504.
https:/​/​doi.org/​10.1103/​PhysRevLett.127.110504
[43] Guang Hao Low. Classical shadows of fermions with particle number symmetry. arXiv preprint arXiv:2208.08964, 2022. 10.48550/​arXiv.2208.08964.
https:/​/​doi.org/​10.48550/​arXiv.2208.08964
arXiv:2208.08964
[44] You Zhou, Pei Zeng, and Zhenhuan Liu. Single-copies estimation of entanglement negativity. Phys. Rev. Lett., 125: 200502, Nov 2020. 10.1103/​PhysRevLett.125.200502.
https:/​/​doi.org/​10.1103/​PhysRevLett.125.200502
[45] Andreas Ketterer, Nikolai Wyderka, and Otfried Gühne. Characterizing multipartite entanglement with moments of random correlations. Phys. Rev. Lett., 122: 120505, Mar 2019. 10.1103/​PhysRevLett.122.120505.
https:/​/​doi.org/​10.1103/​PhysRevLett.122.120505
[46] Xiao-Dong Yu, Satoya Imai, and Otfried Gühne. Optimal entanglement certification from moments of the partial transpose. Phys. Rev. Lett., 127: 060504, Aug 2021. 10.1103/​PhysRevLett.127.060504.
https:/​/​doi.org/​10.1103/​PhysRevLett.127.060504
[47] Zhenhuan Liu, Pei Zeng, You Zhou, and Mile Gu. Characterizing correlation within multipartite quantum systems via local randomized measurements. Phys. Rev. A, 105: 022407, Feb 2022b. 10.1103/​PhysRevA.105.022407.
https:/​/​doi.org/​10.1103/​PhysRevA.105.022407
[48] Jonas Helsen and Michael Walter. Thrifty shadow estimation: re-using quantum circuits and bounding tails. arXiv preprint arXiv:2212.06240, 2022. 10.48550/​arXiv.2212.06240.
https:/​/​doi.org/​10.48550/​arXiv.2212.06240
arXiv:2212.06240
[49] Benoit Collins and Piotr Sniady. Integration with respect to the haar measure on unitary, orthogonal and symplectic group. Communications in Mathematical Physics, 264 (3): 773–795, 2006. ISSN 1432-0916. 10.1007/​s00220-006-1554-3.
https:/​/​doi.org/​10.1007/​s00220-006-1554-3
[50] Daniel A. Roberts and Beni Yoshida. Chaos and complexity by design. Journal of High Energy Physics, 2017 (4): 121, 2017. ISSN 1029-8479. 10.1007/​JHEP04(2017)121.
https:/​/​doi.org/​10.1007/​JHEP04(2017)121
[51] I. Roth, R. Kueng, S. Kimmel, Y.-K. Liu, D. Gross, J. Eisert, and M. Kliesch. Recovering quantum gates from few average gate fidelities. Phys. Rev. Lett., 121: 170502, Oct 2018. 10.1103/​PhysRevLett.121.170502.
https:/​/​doi.org/​10.1103/​PhysRevLett.121.170502
[52] Lorenzo Leone, Salvatore F. E. Oliviero, You Zhou, and Alioscia Hamma. Quantum Chaos is Quantum. Quantum, 5: 453, May 2021. ISSN 2521-327X. 10.22331/​q-2021-05-04-453.
https:/​/​doi.org/​10.22331/​q-2021-05-04-453
Cited by
[1] Christian Bertoni, Jonas Haferkamp, Marcel Hinsche, Marios Ioannou, Jens Eisert, and Hakop Pashayan, “Shallow shadows: Expectation estimation using low-depth random Clifford circuits”, arXiv:2209.12924, (2022).
[2] Benoît Vermersch, Aniket Rath, Bharathan Sundar, Cyril Branciard, John Preskill, and Andreas Elben, “Enhanced estimation of quantum properties with common randomized measurements”, arXiv:2304.12292, (2023).
The above citations are from SAO/NASA ADS (last updated successfully 2023-06-29 13:54:32). The list may be incomplete as not all publishers provide suitable and complete citation data.
Could not fetch Crossref cited-by data during last attempt 2023-06-29 13:54:30: Could not fetch cited-by data for 10.22331/q-2023-06-29-1044 from Crossref. This is normal if the DOI was registered recently.
This Paper is published in Quantum under the Creative Commons Attribution 4.0 International (CC BY 4.0) license. Copyright remains with the original copyright holders such as the authors or their institutions.
- SEO Powered Content & PR Distribution. Get Amplified Today.
- PlatoData.Network Vertical Generative Ai. Empower Yourself. Access Here.
- PlatoAiStream. Web3 Intelligence. Knowledge Amplified. Access Here.
- PlatoESG. Automotive / EVs, Carbon, CleanTech, Energy, Environment, Solar, Waste Management. Access Here.
- BlockOffsets. Modernizing Environmental Offset Ownership. Access Here.
- Source: https://quantum-journal.org/papers/q-2023-06-29-1044/
- :is
- :not
- :where
- 1
- 10
- 11
- 12
- 13
- 14
- 15%
- 16
- 17
- 19
- 20
- 2006
- 2012
- 2013
- 2016
- 2017
- 2018
- 2019
- 2020
- 2021
- 2022
- 2023
- 22
- 23
- 24
- 25
- 26
- 27
- 28
- 30
- 31
- 32
- 33
- 385
- 39
- 40
- 46
- 49
- 50
- 51
- 7
- 8
- 9
- a
- above
- ABSTRACT
- access
- addition
- ADvantage
- affiliations
- After
- AL
- alireza
- All
- also
- an
- analysis
- Analytic
- analyze
- analyzes
- and
- Andrew
- anna
- Another
- Application
- applications
- applied
- approach
- ARE
- Arthur
- AS
- Aug
- author
- authors
- average
- avoiding
- barren
- BE
- Benjamin
- Bill
- Block
- both
- Break
- Brian
- by
- CAN
- Certification
- Chaos
- characterized
- Charles
- chemistry
- chen
- Cheng
- chin
- chong
- Chris
- Collins
- comment
- Common
- Commons
- Communications
- complete
- complexity
- computer
- computing
- Conduct
- continuous
- copyright
- Correlation
- correlations
- Cost
- could
- Current
- DAI
- Daniel
- data
- Dave
- David
- de
- Den
- Design
- designs
- discovery
- discuss
- document
- during
- dynamics
- e
- E&T
- Education
- Edward
- efficient
- energy
- enhanced
- error
- Ether (ETH)
- eugene
- evolution
- expectation
- experiments
- expressions
- fails
- feasible
- Feb
- few
- Figure
- Find
- flexible
- For
- formula
- Framework
- from
- fu
- functions
- fundamental
- further
- Gates
- giuseppe
- Give
- gives
- gross
- Group
- guarantee
- guidance
- harvard
- help
- here
- High
- holders
- HTTPS
- huang
- Hybrid
- i
- IEEE
- if
- image
- in
- indicate
- information
- institutions
- integration
- interest
- interesting
- International
- introduce
- introduced
- IT
- Jan
- JavaScript
- John
- Jordan
- journal
- kenneth
- Key
- laboratory
- Last
- learning
- Leave
- li
- License
- Lincoln
- List
- local
- locally
- logical
- Low
- machine
- machine learning
- many
- mark
- Martin
- mathematical
- max
- max-width
- May..
- meantime
- measure
- measurement
- measurements
- measuring
- method
- Michael
- mikhail
- ministry
- mitigation
- Moments
- Month
- Nature
- networks
- New
- Nguyen
- Noise
- normal
- number
- Oct
- of
- on
- ONE
- open
- opportunities
- optimal
- optimizing
- or
- original
- our
- out
- Paper
- particle
- particular
- performance
- performed
- performs
- Peter
- physical
- Physics
- plato
- Plato Data Intelligence
- PlatoData
- Practical
- predicting
- prepared
- problems
- procedure
- processors
- properties
- property
- provably
- provide
- published
- publisher
- publishers
- Qi
- Quantum
- quantum advantage
- Quantum Computer
- quantum systems
- random
- Randomized
- realization
- recently
- recovering
- references
- registered
- related
- remains
- repeated
- research
- respect
- result
- review
- Reviews
- Richard
- ROBERT
- robust
- rounds
- roy
- Ryan
- s
- Sam
- same
- scalable
- scenario
- schemes
- Science
- scientific
- scott
- scott aaronson
- settings
- Shadow
- shallow
- shanghai
- show
- siam
- significant
- Simon
- simulation
- some
- State
- States
- statistical
- steven
- style
- Successfully
- such
- suitable
- Sun
- supplement
- system
- Systems
- Task
- tasks
- Testing
- that
- The
- their
- theoretical
- theory
- There.
- this
- times
- Title
- to
- tomography
- Toolbox
- Total
- Transactions
- under
- unified
- university
- updated
- URL
- using
- value
- variables
- various
- very
- via
- vincent
- volume
- W
- want
- was
- waves
- we
- which
- with
- within
- Work
- wu
- X
- year
- you
- Yuan
- zephyrnet
- Zhao