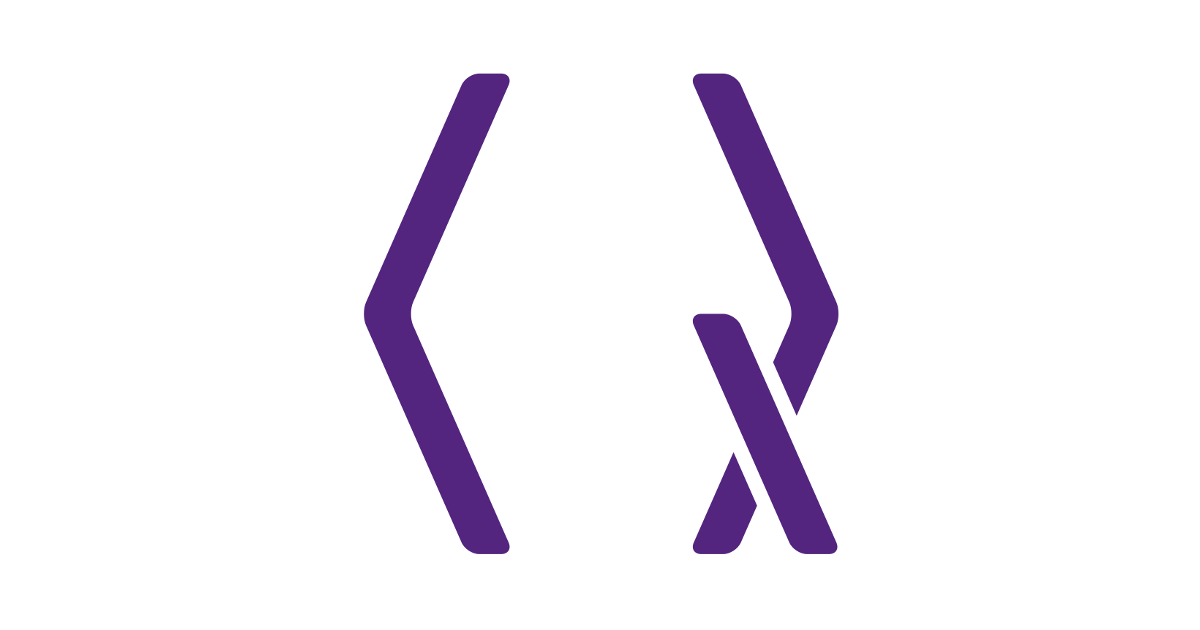
QuOne Lab, Phanous Research & Innovation Centre, Tehran, Iran
Find this paper interesting or want to discuss? Scite or leave a comment on SciRate.
Abstract
In the training of over-parameterized model functions via gradient descent, sometimes the parameters do not change significantly and remain close to their initial values. This phenomenon is called $textit{lazy training}$ and motivates consideration of the linear approximation of the model function around the initial parameters. In the lazy regime, this linear approximation imitates the behavior of the parameterized function whose associated kernel, called the $textit{tangent kernel}$, specifies the training performance of the model. Lazy training is known to occur in the case of (classical) neural networks with large widths. In this paper, we show that the training of $textit{geometrically local}$ parameterized quantum circuits enters the lazy regime for large numbers of qubits. More precisely, we prove bounds on the rate of changes of the parameters of such a geometrically local parameterized quantum circuit in the training process, and on the precision of the linear approximation of the associated quantum model function; both of these bounds tend to zero as the number of qubits grows. We support our analytic results with numerical simulations.
► BibTeX data
► References
[1] John Preskill. Quantum Computing in the NISQ era and beyond. Quantum, 2:79, 2018. doi:10.22331/q-2018-08-06-79.
https://doi.org/10.22331/q-2018-08-06-79
[2] Marco Cerezo, Andrew Arrasmith, Ryan Babbush, Simon C Benjamin, Suguru Endo, Keisuke Fujii, Jarrod R McClean, Kosuke Mitarai, Xiao Yuan, Lukasz Cincio, et al. Variational quantum algorithms. Nature Reviews Physics, 3(9):625–644, 2021. doi:10.1038/s42254-021-00348-9.
https://doi.org/10.1038/s42254-021-00348-9
[3] Jarrod R McClean, Sergio Boixo, Vadim N Smelyanskiy, Ryan Babbush, and Hartmut Neven. Barren plateaus in quantum neural network training landscapes. Nature communications, 9(1):1–6, 2018. doi:10.1038/s41467-018-07090-4.
https://doi.org/10.1038/s41467-018-07090-4
[4] Samson Wang, Enrico Fontana, Marco Cerezo, Kunal Sharma, Akira Sone, Lukasz Cincio, and Patrick J Coles. Noise-induced barren plateaus in variational quantum algorithms. Nature communications, 12(1):1–11, 2021. doi:10.1038/s41467-021-27045-6.
https://doi.org/10.1038/s41467-021-27045-6
[5] Francis Bach. Effortless optimization through gradient flows. https://francisbach.com/gradient-flows.
https://francisbach.com/gradient-flows
[6] Arthur Jacot, Franck Gabriel, and Clément Hongler. Neural tangent kernel: Convergence and generalization in neural networks. Advances in neural information processing systems (NeurIPS 2018), 31:8571–8580, 2018. doi:10.1145/3406325.3465355.
https://doi.org/10.1145/3406325.3465355
[7] Lenaic Chizat, Edouard Oyallon, and Francis Bach. On lazy training in differentiable programming. Advances in Neural Information Processing Systems, 32, 2019.
[8] Kouhei Nakaji, Hiroyuki Tezuka, and Naoki Yamamoto. Quantum-enhanced neural networks in the neural tangent kernel framework. 2021. arXiv:2109.03786.
arXiv:2109.03786
[9] Norihito Shirai, Kenji Kubo, Kosuke Mitarai, and Keisuke Fujii. Quantum tangent kernel. 2021. arXiv:2111.02951.
arXiv:2111.02951
[10] Maria Schuld and Nathan Killoran. Quantum machine learning in feature hilbert spaces. Phys. Rev. Lett., 122:040504, Feb 2019. doi:10.1103/PhysRevLett.122.040504.
https://doi.org/10.1103/PhysRevLett.122.040504
[11] Vojtěch Havlíček, Antonio D. Córcoles, Kristan Temme, Aram W. Harrow, Abhinav Kandala, Jerry M. Chow, and Jay M. Gambetta. Supervised learning with quantum-enhanced feature spaces. Nature, 567(7747):209–212, Mar 2019. doi:10.1038/s41586-019-0980-2.
https://doi.org/10.1038/s41586-019-0980-2
[12] Junyu Liu, Francesco Tacchino, Jennifer R. Glick, Liang Jiang, and Antonio Mezzacapo. Representation learning via quantum neural tangent kernels. PRX Quantum, 3:030323, 2022. doi:10.1103/PRXQuantum.3.030323.
https://doi.org/10.1103/PRXQuantum.3.030323
[13] Di Luo and James Halverson. Infinite neural network quantum states. 2021. arXiv:2112.00723.
arXiv:2112.00723
[14] Junyu Liu, Khadijeh Najafi, Kunal Sharma, Francesco Tacchino, Liang Jiang, and Antonio Mezzacapo. An analytic theory for the dynamics of wide quantum neural networks. Phys. Rev. Lett., 130(15):150601, 2023. doi:10.1103/PhysRevLett.130.150601.
https://doi.org/10.1103/PhysRevLett.130.150601
[15] Junyu Liu, Zexi Lin, and Liang Jiang. Laziness, barren plateau, and noise in machine learning, 2022. doi:10.48550/arXiv.2206.09313.
https://doi.org/10.48550/arXiv.2206.09313
[16] Edward Farhi and Hartmut Neven. Classification with quantum neural networks on near term processors. 2018. arXiv:1802.06002.
arXiv:1802.06002
[17] M. Cerezo, Akira Sone, Tyler Volkoff, Lukasz Cincio, and Patrick J. Coles. Cost function dependent barren plateaus in shallow parametrized quantum circuits. Nature Communications, 12(1):1791, 2021. doi:10.1038/s41467-021-21728-w.
https://doi.org/10.1038/s41467-021-21728-w
[18] Adrián Pérez-Salinas, Alba Cervera-Lierta, Elies Gil-Fuster, and José I. Latorre. Data re-uploading for a universal quantum classifier. Quantum, 4:226, 2020. doi:10.22331/q-2020-02-06-226.
https://doi.org/10.22331/q-2020-02-06-226
[19] Maria Schuld, Ryan Sweke, and Johannes Jakob Meyer. Effect of data encoding on the expressive power of variational quantum-machine-learning models. Phys. Rev. A, 103:032430, Mar 2021. doi:10.1103/PhysRevA.103.032430.
https://doi.org/10.1103/PhysRevA.103.032430
[20] Colin McDiarmid. On the method of bounded differences. In Surveys in combinatorics, 1989 (Norwich, 1989), volume 141 of London Math. Soc. Lecture Note Ser., pages 148–188. Cambridge Univ. Press, Cambridge, 1989. doi:10.1017/cbo9781107359949.008.
https://doi.org/10.1017/cbo9781107359949.008
[21] Ville Bergholm, Josh Izaac, Maria Schuld, Christian Gogolin, M Sohaib Alam, Shahnawaz Ahmed, Juan Miguel Arrazola, Carsten Blank, Alain Delgado, Soran Jahangiri, et al. Pennylane: Automatic differentiation of hybrid quantum-classical computations. 2018. arXiv:1811.04968.
arXiv:1811.04968
[22] Kerstin Beer, Dmytro Bondarenko, Terry Farrelly, Tobias J Osborne, Robert Salzmann, Daniel Scheiermann, and Ramona Wolf. Training deep quantum neural networks. Nature communications, 11(1):1–6, 2020. doi:10.1038/s41467-020-14454-2.
https://doi.org/10.1038/s41467-020-14454-2
Cited by
[1] Junyu Liu, Zexi Lin, and Liang Jiang, “Laziness, Barren Plateau, and Noise in Machine Learning”, arXiv:2206.09313, (2022).
[2] Yuxuan Du, Min-Hsiu Hsieh, Tongliang Liu, Shan You, and Dacheng Tao, “Erratum: Learnability of Quantum Neural Networks [PRX QUANTUM 2, 040337 (2021)]”, PRX Quantum 3 3, 030901 (2022).
The above citations are from SAO/NASA ADS (last updated successfully 2023-04-29 00:32:34). The list may be incomplete as not all publishers provide suitable and complete citation data.
On Crossref’s cited-by service no data on citing works was found (last attempt 2023-04-29 00:32:32).
This Paper is published in Quantum under the Creative Commons Attribution 4.0 International (CC BY 4.0) license. Copyright remains with the original copyright holders such as the authors or their institutions.
- SEO Powered Content & PR Distribution. Get Amplified Today.
- PlatoAiStream. Web3 Data Intelligence. Knowledge Amplified. Access Here.
- Minting the Future w Adryenn Ashley. Access Here.
- Source: https://quantum-journal.org/papers/q-2023-04-27-989/
- :is
- :not
- 1
- 10
- 11
- 12
- 13
- 14
- 20
- 2018
- 2019
- 2020
- 2021
- 2022
- 2023
- 22
- 7
- 8
- 9
- a
- above
- ABSTRACT
- access
- advances
- affiliations
- AL
- algorithms
- All
- an
- Analytic
- and
- Andrew
- apr
- ARE
- around
- Arthur
- AS
- associated
- author
- authors
- Automatic
- BE
- beer
- Benjamin
- Beyond
- both
- Break
- by
- called
- cambridge
- case
- centre
- change
- Changes
- classification
- Close
- comment
- Commons
- Communications
- complete
- computations
- computing
- consideration
- Convergence
- copyright
- Cost
- Daniel
- data
- deep
- Den
- dependent
- differences
- discuss
- do
- dynamics
- E&T
- Edward
- effect
- Enters
- Era
- Ether (ETH)
- expressive
- Feature
- Feb
- Flows
- For
- found
- Framework
- Francis
- from
- function
- functions
- Grows
- harvard
- holders
- HTTPS
- Hybrid
- hybrid quantum-classical
- i
- in
- information
- initial
- Innovation
- institutions
- interesting
- International
- JavaScript
- Jennifer
- John
- journal
- known
- lab
- large
- Last
- learning
- Leave
- Lecture
- License
- List
- local
- London
- machine
- machine learning
- Marco
- math
- May..
- method
- Meyer
- model
- models
- Month
- more
- Nature
- Near
- network
- networks
- Neural
- neural network
- neural networks
- NeurIPS
- no
- Noise
- note
- number
- numbers
- of
- on
- open
- optimization
- or
- original
- our
- Paper
- parameters
- performance
- phenomenon
- Physics
- plato
- Plato Data Intelligence
- PlatoData
- power
- precisely
- Precision
- press
- process
- processing
- processors
- Programming
- Prove
- provide
- published
- publisher
- publishers
- Quantum
- quantum algorithms
- quantum computing
- quantum machine learning
- qubits
- Rate
- references
- regime
- remain
- remains
- representation
- research
- Results
- Reviews
- ROBERT
- Ryan
- s
- shallow
- Sharma
- show
- significantly
- Simon
- spaces
- States
- Successfully
- such
- suitable
- supervised learning
- support
- Systems
- tehran
- term
- Tezuka
- that
- The
- their
- These
- this
- Through
- Title
- to
- Training
- under
- Universal
- updated
- URL
- Values
- via
- volume
- W
- want
- was
- we
- wide
- with
- Wolf
- works
- year
- you
- Yuan
- zephyrnet
- zero