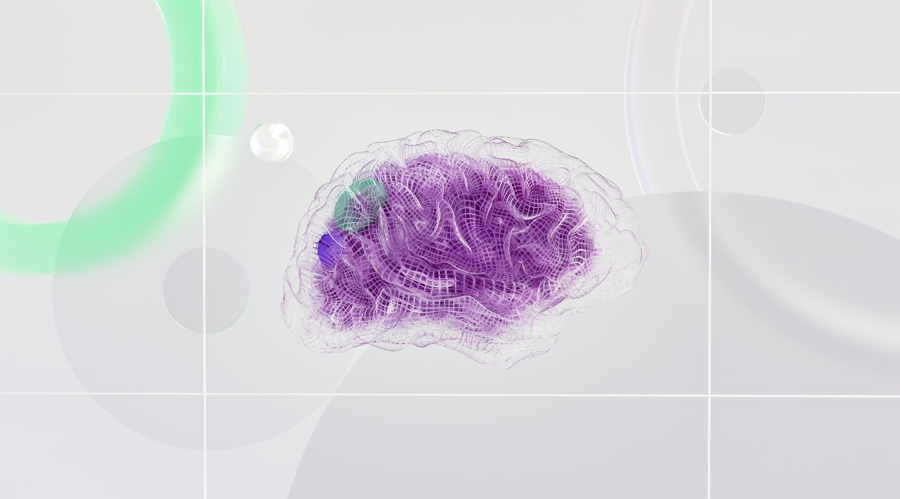
Data has
emerged as a critical resource for training clever algorithms in the
fast-evolving world of artificial intelligence (AI). As businesses work to
develop and improve AI systems, the topic of whether AIs should be trained on
free data arises.
This article
delves into the discussion, presenting reasons for and against providing data
for free, as well as exploring the benefits and ethical considerations raised
by this issue.
The Benefits
of Free AI Training Data
Proponents of
open data say that it supports innovation, expands access to AI technology, and
promotes societal advantages. Here are some significant points in support of
this point of view:
Access to
Diverse Data: Making training data available for free helps AI developers to
access a wide range of datasets, improving the accuracy and efficacy of AI
models across many domains.
Free data
enables smaller organizations and individual researchers to explore and develop
creative AI solutions that can address societal concerns more efficiently by
decreasing the barriers to entry.
Open access to
training data encourages knowledge sharing and collaboration across the AI
community, facilitating joint growth and eliminating redundancy in data
collection operations.
The
Opposition to Free AI Training Data
Critics believe
that offering free data raises serious ethical and economic concerns,
potentially leading to exploitation, privacy violations, and restricting
chances for data-driven firms. The following are the main arguments against
open AI training data:
Ownership
and Control of Data
Allowing
unfettered access to data raises concerns about who owns and controls the
valuable information. This can lead to exploitation, in which data creators are
not fairly compensated for their efforts.
Data Bias and Representational Issues
Free AI
training datasets, often collected from various online sources, can suffer from
inherent biases and representational issues. These biases reflect the
characteristics and viewpoints of the data sources and may perpetuate existing
societal biases or stereotypes. Biased training data can lead to discriminatory
or inaccurate AI models, causing harm or unfair treatment to individuals or
groups.
Additionally,
free AI training datasets may not be representative of the real-world
population, resulting in skewed or incomplete models. This lack of diversity
can limit the AI system’s ability to handle edge cases, recognize
underrepresented groups, or provide accurate predictions in diverse scenarios.
Data Quality and Reliability
Ensuring the
quality and reliability of training data is essential for building robust and
effective AI models. Free datasets often lack the necessary quality control
measures and standards. They may contain inaccuracies, noise, or
inconsistencies that can negatively impact the performance of AI systems.
Inadequate data quality can lead to unreliable predictions, reduced accuracy,
and poor generalization to new scenarios.
Moreover, the
provenance and authenticity of free training data can be questionable. Without
proper verification and validation processes, there is a higher risk of
incorporating misleading or fraudulent data into AI models. Reliance on
unverified data sources can undermine the credibility and integrity of AI
systems.
Privacy and
security risks
Making data
available for free may jeopardize individuals’ privacy by allowing sensitive
personal information to be utilized without consent or sufficient safeguards.
Data leaks and illegal access are two potential hazards of broad data sharing.
Market
Distortions
Making data
available for free may impede competition by favoring large firms with the
capabilities to handle large datasets. This could result in an unequal playing
field, deterring smaller businesses from entering the market and stifling
innovation.
Legal and Ethical Concerns
The use of free
AI training data raises legal and ethical concerns related to data ownership,
intellectual property rights, and privacy. Data collected without proper
consent or in violation of privacy regulations can have serious legal
consequences for organizations. Using such data for training AI models can lead
to legal disputes, reputational damage, and regulatory non-compliance.
Furthermore,
free datasets may not adhere to ethical guidelines and standards. They may
include sensitive or private information that should not be used without
explicit consent or proper anonymization. Failing to respect ethical
considerations can erode trust and harm individuals’ privacy rights.
Conclusion
The subject of
whether AIs should be educated on free data raises difficult issues at the
junction of ethics, economics, and technological progress. While supporters
believe that free data may spur innovation and societal advantages, detractors
raise legitimate concerns about privacy, ownership, and market distortions.
To address the
issues connected with data access and AI training, appropriate regulations and
procedures will be required to strike a balance between accessibility and
fairness. As the AI landscape changes, it is critical to keep this debate going
and create equitable solutions that maximize AI’s promise while protecting
individual rights and economic fairness.
Data has
emerged as a critical resource for training clever algorithms in the
fast-evolving world of artificial intelligence (AI). As businesses work to
develop and improve AI systems, the topic of whether AIs should be trained on
free data arises.
This article
delves into the discussion, presenting reasons for and against providing data
for free, as well as exploring the benefits and ethical considerations raised
by this issue.
The Benefits
of Free AI Training Data
Proponents of
open data say that it supports innovation, expands access to AI technology, and
promotes societal advantages. Here are some significant points in support of
this point of view:
Access to
Diverse Data: Making training data available for free helps AI developers to
access a wide range of datasets, improving the accuracy and efficacy of AI
models across many domains.
Free data
enables smaller organizations and individual researchers to explore and develop
creative AI solutions that can address societal concerns more efficiently by
decreasing the barriers to entry.
Open access to
training data encourages knowledge sharing and collaboration across the AI
community, facilitating joint growth and eliminating redundancy in data
collection operations.
The
Opposition to Free AI Training Data
Critics believe
that offering free data raises serious ethical and economic concerns,
potentially leading to exploitation, privacy violations, and restricting
chances for data-driven firms. The following are the main arguments against
open AI training data:
Ownership
and Control of Data
Allowing
unfettered access to data raises concerns about who owns and controls the
valuable information. This can lead to exploitation, in which data creators are
not fairly compensated for their efforts.
Data Bias and Representational Issues
Free AI
training datasets, often collected from various online sources, can suffer from
inherent biases and representational issues. These biases reflect the
characteristics and viewpoints of the data sources and may perpetuate existing
societal biases or stereotypes. Biased training data can lead to discriminatory
or inaccurate AI models, causing harm or unfair treatment to individuals or
groups.
Additionally,
free AI training datasets may not be representative of the real-world
population, resulting in skewed or incomplete models. This lack of diversity
can limit the AI system’s ability to handle edge cases, recognize
underrepresented groups, or provide accurate predictions in diverse scenarios.
Data Quality and Reliability
Ensuring the
quality and reliability of training data is essential for building robust and
effective AI models. Free datasets often lack the necessary quality control
measures and standards. They may contain inaccuracies, noise, or
inconsistencies that can negatively impact the performance of AI systems.
Inadequate data quality can lead to unreliable predictions, reduced accuracy,
and poor generalization to new scenarios.
Moreover, the
provenance and authenticity of free training data can be questionable. Without
proper verification and validation processes, there is a higher risk of
incorporating misleading or fraudulent data into AI models. Reliance on
unverified data sources can undermine the credibility and integrity of AI
systems.
Privacy and
security risks
Making data
available for free may jeopardize individuals’ privacy by allowing sensitive
personal information to be utilized without consent or sufficient safeguards.
Data leaks and illegal access are two potential hazards of broad data sharing.
Market
Distortions
Making data
available for free may impede competition by favoring large firms with the
capabilities to handle large datasets. This could result in an unequal playing
field, deterring smaller businesses from entering the market and stifling
innovation.
Legal and Ethical Concerns
The use of free
AI training data raises legal and ethical concerns related to data ownership,
intellectual property rights, and privacy. Data collected without proper
consent or in violation of privacy regulations can have serious legal
consequences for organizations. Using such data for training AI models can lead
to legal disputes, reputational damage, and regulatory non-compliance.
Furthermore,
free datasets may not adhere to ethical guidelines and standards. They may
include sensitive or private information that should not be used without
explicit consent or proper anonymization. Failing to respect ethical
considerations can erode trust and harm individuals’ privacy rights.
Conclusion
The subject of
whether AIs should be educated on free data raises difficult issues at the
junction of ethics, economics, and technological progress. While supporters
believe that free data may spur innovation and societal advantages, detractors
raise legitimate concerns about privacy, ownership, and market distortions.
To address the
issues connected with data access and AI training, appropriate regulations and
procedures will be required to strike a balance between accessibility and
fairness. As the AI landscape changes, it is critical to keep this debate going
and create equitable solutions that maximize AI’s promise while protecting
individual rights and economic fairness.
- SEO Powered Content & PR Distribution. Get Amplified Today.
- PlatoData.Network Vertical Generative Ai. Empower Yourself. Access Here.
- PlatoAiStream. Web3 Intelligence. Knowledge Amplified. Access Here.
- PlatoESG. Automotive / EVs, Carbon, CleanTech, Energy, Environment, Solar, Waste Management. Access Here.
- BlockOffsets. Modernizing Environmental Offset Ownership. Access Here.
- Source: https://www.financemagnates.com//fintech/data/should-ais-be-trained-on-data-for-free/
- :has
- :is
- :not
- a
- ability
- About
- access
- Access to data
- accessibility
- accuracy
- accurate
- across
- address
- adhere
- advantages
- against
- AI
- AI systems
- AI training
- algorithms
- Allowing
- an
- and
- appropriate
- ARE
- arguments
- article
- artificial
- artificial intelligence
- Artificial intelligence (AI)
- AS
- At
- authenticity
- available
- Balance
- banner
- barriers
- BE
- believe
- benefits
- between
- bias
- biased
- biases
- broad
- Building
- businesses
- by
- CAN
- capabilities
- cases
- causing
- chances
- characteristics
- collaboration
- collection
- community
- compensated
- competition
- Concerns
- connected
- consent
- Consequences
- considerations
- control
- controls
- could
- create
- Creative
- creators
- Credibility
- critical
- damage
- data
- data access
- data bias
- data quality
- data sharing
- data-driven
- datasets
- debate
- develop
- developers
- difficult
- discussion
- disputes
- diverse
- Diversity
- domains
- Economic
- Economics
- Edge
- Effective
- efficacy
- efficiently
- efforts
- eliminating
- emerged
- enables
- encourages
- entering
- entry
- equitable
- essential
- ethical
- ethics
- existing
- expands
- exploitation
- explore
- Exploring
- facilitating
- failing
- fairly
- fairness
- field
- firms
- follow
- following
- For
- fraudulent
- Free
- from
- going
- Group’s
- Growth
- guidelines
- handle
- harm
- Have
- helps
- here
- higher
- HTTPS
- Illegal
- Impact
- improve
- improving
- in
- inaccurate
- include
- incorporating
- individual
- individuals
- information
- inherent
- Innovation
- integrity
- intellectual
- intellectual property
- Intelligence
- into
- issue
- issues
- IT
- Jeopardize
- joint
- jpg
- Keep
- knowledge
- Lack
- landscape
- large
- lead
- leading
- Leaks
- Legal
- legitimate
- LIMIT
- Main
- Making
- many
- Market
- Maximize
- May..
- measures
- misleading
- models
- more
- necessary
- negatively
- New
- Noise
- of
- offering
- often
- on
- online
- open
- open data
- Operations
- opposition
- or
- organizations
- ownership
- owns
- performance
- personal
- personal information
- plato
- Plato Data Intelligence
- PlatoData
- playing
- Point
- Point of View
- points
- poor
- population
- potential
- potentially
- Predictions
- privacy
- private
- private information
- procedures
- processes
- Progress
- promise
- promotes
- proper
- property
- Property Rights
- protecting
- provenance
- provide
- providing
- quality
- raise
- raised
- raises
- range
- real world
- reasons
- recognize
- Reduced
- reflect
- regulations
- regulatory
- related
- reliability
- reliance
- representative
- required
- researchers
- resource
- respect
- restricting
- result
- resulting
- rights
- Risk
- robust
- s
- safeguards
- say
- scenarios
- security
- sensitive
- serious
- sharing
- should
- significant
- smaller
- societal
- Solutions
- some
- Sources
- standards
- strike
- subject
- such
- sufficient
- support
- supporters
- Supports
- system
- Systems
- technological
- Technology
- that
- The
- their
- There.
- These
- they
- this
- to
- topic
- trained
- Training
- treatment
- Trust
- two
- Undermine
- underrepresented
- unfair
- use
- used
- using
- utilized
- validation
- Valuable
- Valuable Information
- various
- Verification
- View
- viewpoints
- VIOLATION
- Violations
- WELL
- whether
- which
- while
- WHO
- wide
- Wide range
- will
- with
- without
- Work
- world
- zephyrnet