<!—->
<!– –>
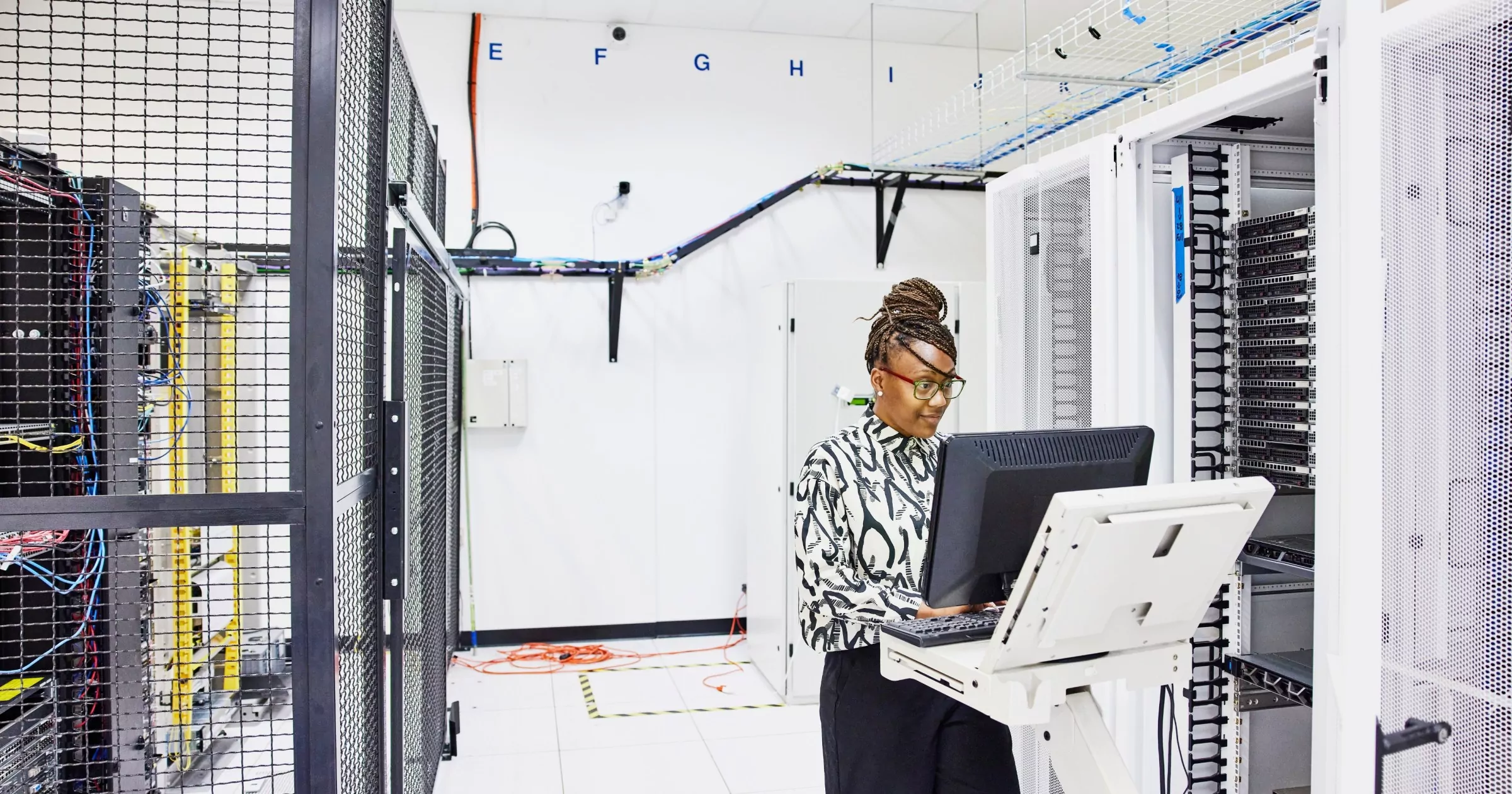
Artificial intelligence (AI) is revolutionizing industries by enabling advanced analytics, automation and personalized experiences. Enterprises have reported a 30% productivity gain in application modernization after implementing Gen AI. However, the success of AI initiatives heavily depends on the underlying infrastructure’s ability to support demanding workloads efficiently. In this blog, we’ll explore seven key strategies to optimize infrastructure for AI workloads, empowering organizations to harness the full potential of AI technologies.
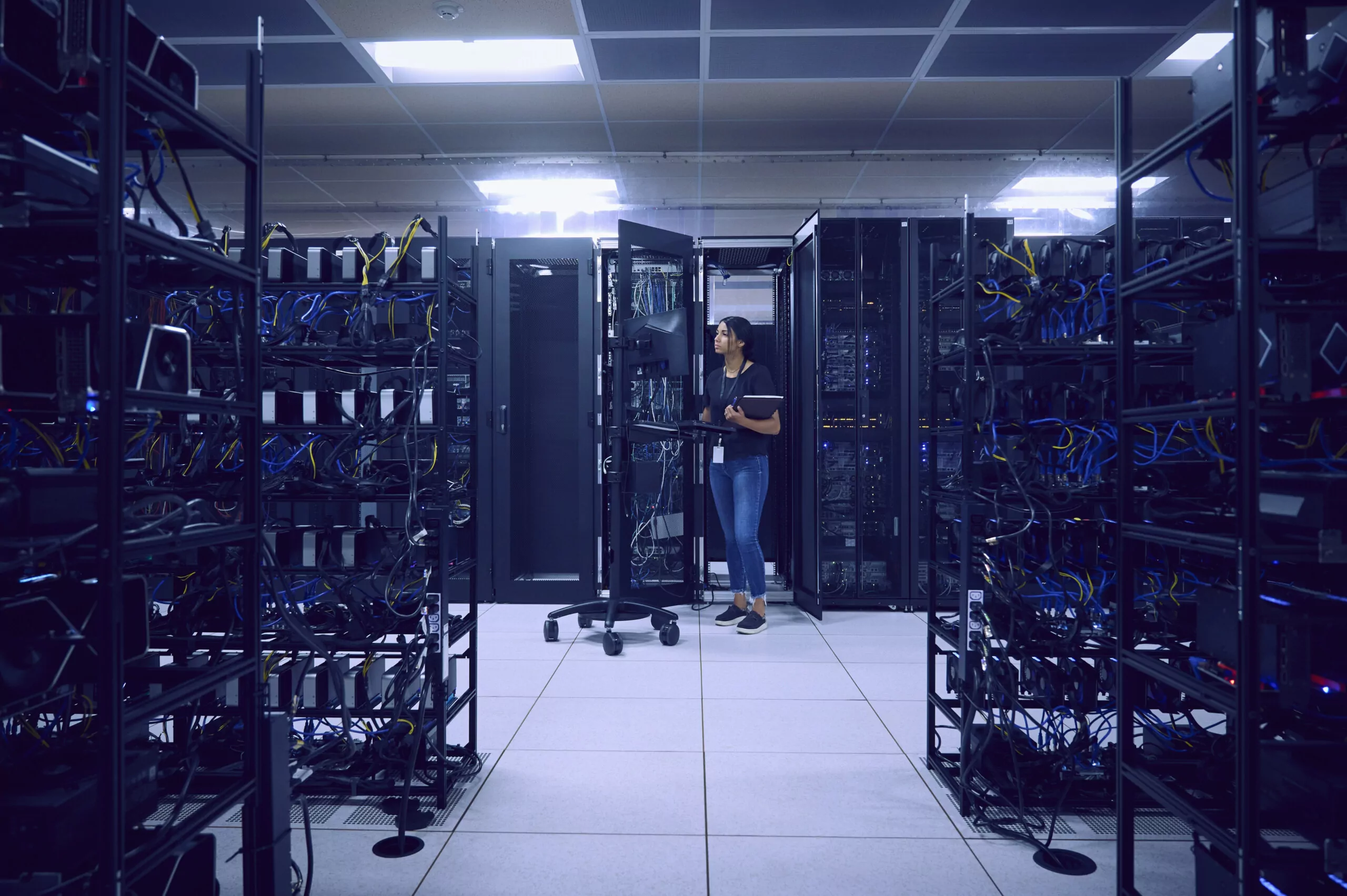
1. High-performance computing systems
Investing in high-performance computing systems tailored for AI accelerates model training and inference tasks. GPUs (graphics processing units) and TPUs (tensor processing units) are specifically designed to handle complex mathematical computations central to AI algorithms, offering significant speedups compared with traditional CPUs.
2. Scalable and elastic resources
Scalability is paramount for handling AI workloads that vary in complexity and demand over time. Cloud platforms and container orchestration technologies provide scalable, elastic resources that dynamically allocate compute, storage and networking resources based on workload requirements. This flexibility ensures optimal performance without over-provisioning or underutilization.
3. Accelerated data processing
Efficient data processing pipelines are critical for AI workflows, especially those involving large datasets. Leveraging distributed storage and processing frameworks such as Apache Hadoop, Spark or Dask accelerates data ingestion, transformation and analysis. Additionally, using in-memory databases and caching mechanisms minimizes latency and improves data access speeds.
4. Parallelization and distributed computing
Parallelizing AI algorithms across multiple compute nodes accelerates model training and inference by distributing computation tasks across a cluster of machines. Frameworks like TensorFlow, PyTorch and Apache Spark MLlib support distributed computing paradigms, enabling efficient utilization of resources and faster time-to-insight.
5. Hardware acceleration
Hardware accelerators like FPGAs (field-programmable gate arrays) and ASICs (application-specific integrated circuits) optimize performance and energy efficiency for specific AI tasks. These specialized processors offload computational workloads from general-purpose CPUs or GPUs, delivering significant speedups for tasks like inferencing, natural language processing and image recognition.
6. Optimized networking infrastructure
Low-latency, high-bandwidth networking infrastructure is essential for distributed AI applications that rely on data-intensive communication between nodes. Deploying high-speed interconnects, such as InfiniBand or RDMA (Remote Direct Memory Access), minimizes communication overhead and accelerates data transfer rates, enhancing overall system performance
7. Continuous monitoring and optimization
Implementing comprehensive monitoring and optimization practices confirm that AI workloads run efficiently and cost-effectively over time. Utilize performance monitoring tools to identify bottlenecks, resource contention and underutilized resources. Continuous optimization techniques, including auto-scaling, workload scheduling and resource allocation algorithms, adapt infrastructure dynamically to evolving workload demands, maximizing resource utilization and cost savings.

Conclusion
Optimizing infrastructure for AI workloads is a multifaceted endeavor that requires a holistic approach encompassing hardware, software and architectural considerations. By embracing high-performance computing systems, scalable resources, accelerated data processing, distributed computing paradigms, hardware acceleration, optimized networking infrastructure and continuous monitoring and optimization practices, organizations can unleash the full potential of AI technologies. Empowered by optimized infrastructure, businesses can drive innovation, unlock new insights and deliver transformative AI-driven solutions that propel them ahead in today’s competitive landscape.
IBM AI infrastructure solutions
IBM® clients can harness the power of multi-access edge computing platform with IBM’s AI solutions and Red Hat hybrid cloud capabilities. With IBM, clients can bring their own existing network and edge infrastructure, and we provide the software that runs on top of it to create a unified solution.
Red Hat OpenShift enables the virtualization and containerization of automation software to provide advanced flexibility in hardware deployment, optimized according to application needs. It also provides efficient system orchestration, enabling real-time, data-based decision making at the edge and further processing in the cloud.
IBM offers a full range of solutions optimized for AI from servers and storage to software and consulting. The latest generation of IBM servers, storage and software can help you modernize and scale on-premises and in the cloud with security-rich hybrid cloud and trusted AI automation and insights.
Learn more about IBM IT Infrastructure Solutions
Was this article helpful?
YesNo
More from IT infrastructure
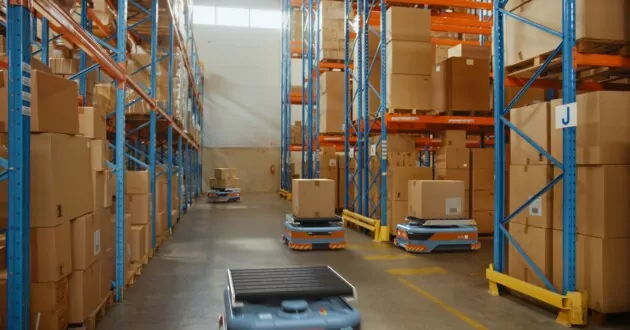
March 20, 2024
Migrate and modernize enterprise integration using IBM Cloud Pak for Integration with Red Hat OpenShift Service on AWS (ROSA)
5 min read – Integration is essential to every business. As businesses consider the core of their IT infrastructure, their focus might be on their data and applications. But without integration, the data would be locked into siloes; and the applications would be isolated and overloaded with complexity as fragile, tightly coupled connections were added to allow applications to work together and share information. This impacts business agility—slowing both actions—and the ability to change. Businesses are trying to reduce these data exchange barriers through…
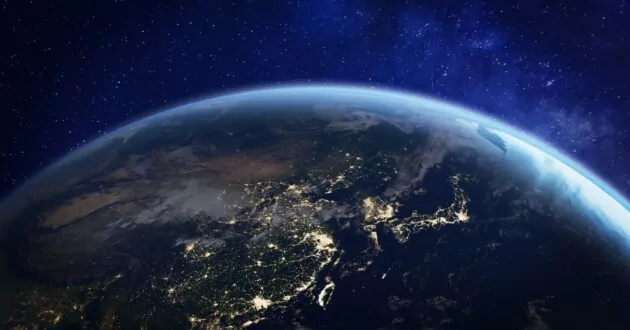
February 22, 2024
IBM Cloud delivers enterprise sovereign cloud capabilities
5 min read – As we see enterprises increasingly face geographic requirements around sovereignty, IBM Cloud® is committed to helping clients navigate beyond the complexity so they can drive true transformation with innovative hybrid cloud technologies. We believe this is particularly important with the rise of generative AI. While AI can undoubtedly offer a competitive edge to organizations that effectively leverage its capabilities, we have seen unique concerns from industry to industry and region to region that must be considered—particularly around data. We strongly…
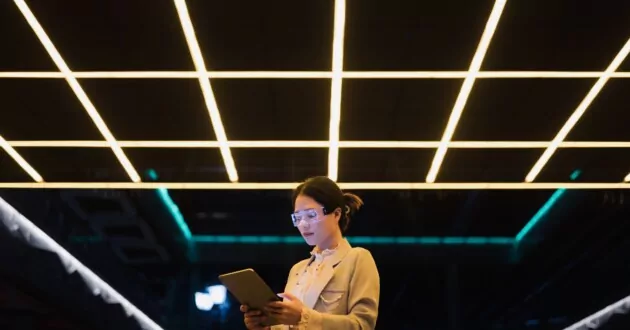
February 14, 2024
Power Virtual Server 2024 edition–Iterative improvements reduce total cost of ownership
4 min read – IBM® has big plans for the Power Virtual Server offering, which is IBM’s virtual machine as-a-service offering based on IBM Power® Systems for AIX®, IBM i and Linux workloads. Over the last year, there’s been a concerted effort to make the offering even more compelling to clients looking to move their Power Systems workload to the cloud. Unlike an on-premises server purchase, a cloud service improves iteratively as new features are delivered behind the scenes and the savings quickly add…
IBM Newsletters
Get our newsletters and topic updates that deliver the latest thought leadership and insights on emerging trends.
Subscribe now
More newsletters
- SEO Powered Content & PR Distribution. Get Amplified Today.
- PlatoData.Network Vertical Generative Ai. Empower Yourself. Access Here.
- PlatoAiStream. Web3 Intelligence. Knowledge Amplified. Access Here.
- PlatoESG. Carbon, CleanTech, Energy, Environment, Solar, Waste Management. Access Here.
- PlatoHealth. Biotech and Clinical Trials Intelligence. Access Here.
- Source: https://www.ibm.com/blog/7-ways-to-optimize-infrastructure-for-ai-workloads/
- :has
- :is
- 00
- 1
- 14
- 2%
- 20
- 2024
- 22
- 28
- 29
- 300
- 32
- 39
- 4
- 400
- 5
- 6
- 7
- 9
- 97
- a
- ability
- About
- accelerated
- accelerates
- acceleration
- accelerators
- access
- According
- across
- adapt
- added
- Additionally
- advanced
- Advertising
- After
- ahead
- AI
- algorithms
- All
- allocate
- allocation
- allow
- also
- amp
- an
- analysis
- analytics
- and
- Apache
- Apache Spark
- Application
- applications
- approach
- architectural
- ARE
- around
- article
- AS
- Asics
- At
- augmented
- Augmented Reality
- augmented reality glasses
- author
- Automation
- AWS
- back
- barriers
- based
- BE
- been
- behind
- behind the scenes
- believe
- between
- Beyond
- Big
- Blog
- Blue
- both
- bottlenecks
- boxes
- bring
- business
- businesses
- but
- button
- by
- caching
- CAN
- capabilities
- carbon
- card
- Cards
- CAT
- Category
- central
- change
- check
- circles
- circuits
- class
- clients
- Cloud
- Cluster
- color
- committed
- Communication
- compared
- compelling
- competitive
- complex
- complexity
- comprehensive
- computation
- computational
- computations
- Compute
- computing
- Concerns
- concerted
- Confirm
- Connections
- Consider
- considerations
- consulting
- Container
- continue
- continuous
- copy
- Core
- Cost
- cost savings
- country
- coupled
- create
- critical
- CSS
- custom
- data
- data access
- Data Exchange
- data processing
- databases
- datasets
- Date
- decision
- Decision Making
- Default
- definitions
- deliver
- delivered
- delivering
- delivers
- Demand
- demanding
- demands
- depends
- deploying
- deployment
- description
- designed
- direct
- distributed
- distributed computing
- distributing
- drive
- dynamically
- earth
- Edge
- edge computing
- effectively
- efficiency
- efficient
- efficiently
- effort
- embracing
- emerging
- empowered
- empowering
- enables
- enabling
- encompassing
- endeavor
- energy
- energy efficiency
- enhancing
- ensures
- Enter
- Enterprise
- enterprises
- especially
- essential
- Ether (ETH)
- Even
- Every
- evolving
- exchange
- existing
- Exit
- Experiences
- explore
- Face
- false
- faster
- Features
- February
- Flexibility
- Focus
- follow
- fonts
- For
- frameworks
- from
- full
- further
- Gain
- gate
- Gen
- general-purpose
- generation
- generative
- Generative AI
- generator
- geographic
- get
- glasses
- GPUs
- graphics
- Grid
- Hadoop
- handle
- Handling
- Hardware
- harness
- hat
- Have
- Heading
- heavily
- height
- help
- helpful
- helping
- high-performance
- holistic
- However
- HTTPS
- Hybrid
- hybrid cloud
- i
- IBM
- IBM Cloud
- ICO
- ICON
- identify
- image
- Image Recognition
- Impacts
- implementing
- important
- improvements
- improves
- in
- Including
- increasingly
- index
- industries
- industry
- information
- Infrastructure
- initiatives
- Innovation
- innovative
- insights
- integrated
- integration
- Intelligence
- interconnects
- into
- intrinsic
- involving
- isolated
- IT
- ITS
- jpg
- Key
- landscape
- language
- large
- Last
- Last Year
- Latency
- latest
- Leadership
- Leverage
- leveraging
- lights
- like
- linux
- local
- locale
- locked
- looking
- machine
- Machines
- make
- Making
- March
- mathematical
- max-width
- maximizing
- mechanisms
- Memory
- might
- migrate
- min
- minimizes
- minutes
- Mobile
- model
- modernization
- modernize
- monitoring
- more
- move
- multifaceted
- multiple
- must
- Natural
- Natural Language
- Natural Language Processing
- Navigate
- Navigation
- needs
- network
- networking
- New
- New Features
- Newsletters
- night
- nodes
- nothing
- now
- of
- off
- offer
- offering
- Offers
- on
- optimal
- optimization
- Optimize
- optimized
- or
- orchestration
- organizations
- our
- over
- overall
- overhead
- own
- ownership
- page
- paradigms
- Paramount
- particularly
- performance
- person
- Personalized
- PHP
- picture
- plans
- platform
- Platforms
- plato
- Plato Data Intelligence
- PlatoData
- plugin
- policy
- position
- Post
- potential
- power
- practices
- primary
- processing
- processors
- Product
- productivity
- professional
- Propel
- provide
- provides
- purchase
- pytorch
- quickly
- range
- Rates
- Reading
- real-time
- Reality
- recognition
- Red
- Red Hat
- reduce
- region
- rely
- remote
- Reported
- Requirements
- requires
- resource
- Resources
- responsive
- Revolutionizing
- Richard
- Rise
- robots
- Room
- ROSA
- Run
- runs
- Savings
- scalable
- Scale
- scenes
- scheduling
- Screen
- scripts
- see
- seen
- seo
- server
- Servers
- service
- seven
- Share
- share information
- showing
- significant
- site
- small
- So
- Software
- solution
- Solutions
- sovereign
- sovereignty
- Space
- Spark
- specialized
- specific
- specifically
- speeds
- Sponsored
- squares
- start
- storage
- strategies
- subscribe
- success
- such
- support
- SVG
- system
- Systems
- Tablet
- tailored
- tasks
- techniques
- Technologies
- tensorflow
- tertiary
- thanks
- that
- The
- their
- Them
- theme
- These
- they
- this
- those
- thought
- thought leadership
- tightly
- time
- Title
- to
- today’s
- together
- tools
- top
- topic
- Total
- traditional
- Training
- transfer
- Transformation
- transformative
- Trends
- true
- trusted
- trying
- type
- underlying
- undoubtedly
- unified
- unique
- units
- unleash
- unleashing
- unlike
- unlock
- Updates
- URL
- using
- utilization
- utilize
- vary
- Virtual
- virtual machine
- W
- Warehouse
- ways
- we
- were
- which
- while
- with
- without
- WordPress
- Work
- work together
- workflows
- would
- written
- year
- you
- young
- Your
- zephyrnet