Introduction
In the expansive landscape of data analytics, one of the most profound developments changing the game is Generative Artificial Intelligence (GAI). It’s an exciting time where AI goes beyond just processing and predicting based on historical data; it’s creating something entirely new, revolutionizing data storytelling and analytical processes. During a recent session, I had the chance to explore this technological innovation’s fundamentals, architectures, and potential impact. Here’s a concise summary of what we covered.
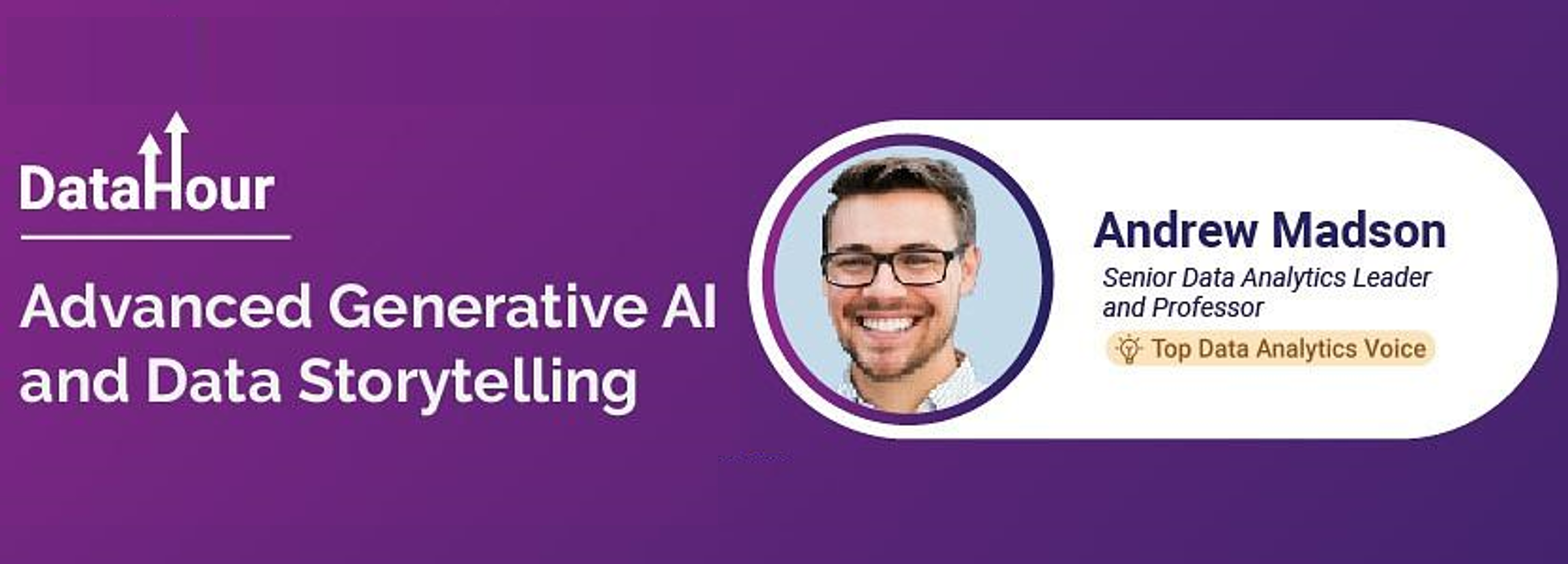
Learning Objectives:
- Understand the fundamentals of generative AI.
- Learn various data storytelling techniques with generative AI.
- Acknowledge the ethical implementation of generative AI in data analytics.
Table of Contents
Understanding Generative AI
Generative AI represents a subset of artificial intelligence that focuses on creating novel content. Traditional AI trains on historical data and makes inferences or predictions. In contrast, generative AI synthesizes new content, spanning visual, audio, and text creation. Several architectures define this field, including Generative Adversarial Networks (GANs), Variational Autoencoders (VAEs), and Autoregressive Models or Transformers.
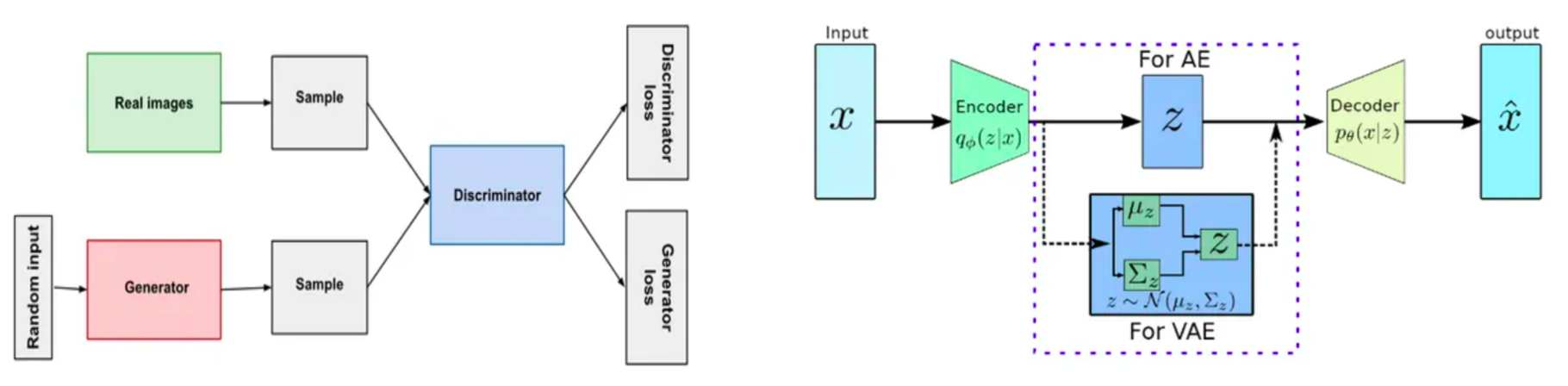
GANs employ two neural networks, a generator and a discriminator, training together. This adversarial process refines both networks by generating data that closely mimics real data while distinguishing between authentic and generated data. VAEs differ slightly but serve the same generative purpose.
Most commonly seen in today’s AI models are Autoregressive Models like ChatGPT, based on Transformers. These models create data sequentially, conditioning on previous elements, and allowing them to predict the next sequence element. Understanding these models provides a strategic edge in leveraging AI effectively.
Data Storytelling: Uniting Generative AI and Analytics
The impact of data analytics lies in data storytelling. While the initial phases focus on defining, collecting, cleaning, and analyzing data, the crux lies in the presentation phase. Here, we must communicate findings effectively. Crafting a narrative, preparing visuals, and examining logic play pivotal roles in storytelling. Using generative AI can significantly impact steps one and two of this process.
This is where storytelling enters the scene. Storytelling in data presentation involves connecting with stakeholders, understanding their needs, and presenting the analysis to facilitate decision-making. However, this phase is often underemphasized in analytical courses, despite being crucial in conveying the impact of the data.
Case Study: Generative AI Driving Business Efficiency Narratives
This case study exemplifies how generative AI, particularly GPT-4, aids an analyst in determining the purpose of their presentation and role clarity. By asking ChatGPT specific questions, such as ‘how to focus on strategically reducing operational costs without layoffs?’, the AI’s suggestions can help guide and refine the narrative and presentation strategy.
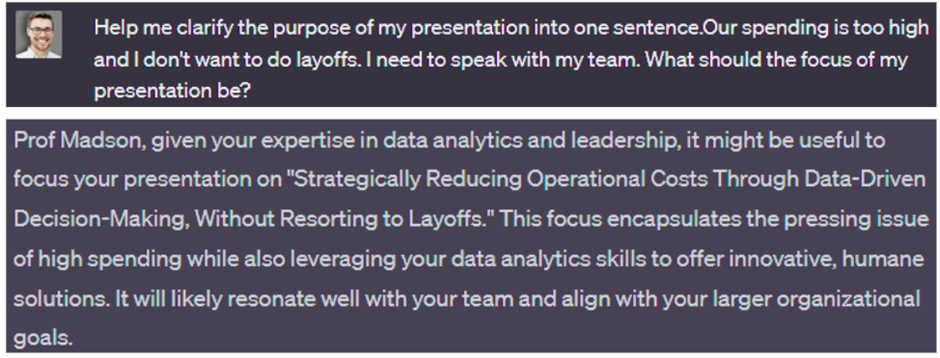
It’s essential to understand that Generative AI doesn’t entirely create the content but rather acts as a brainstorming partner, offering directions and ideas, and allowing analysts to fine-tune their strategies. Here’s how generative AI helps in data analytics and storytelling that drives business efficiency.
Advanced Data Analysis with GPT-4
The advanced capabilities of GPT-4 unlock a wealth of possibilities. In my experience, I’ve opted to use ChatGPT due to its trustworthiness and precision. While there are alternative AI models like LlaMA, each has its unique strengths. I’ve found ChatGPT to be a solid choice, but the others might suit different requirements equally well.
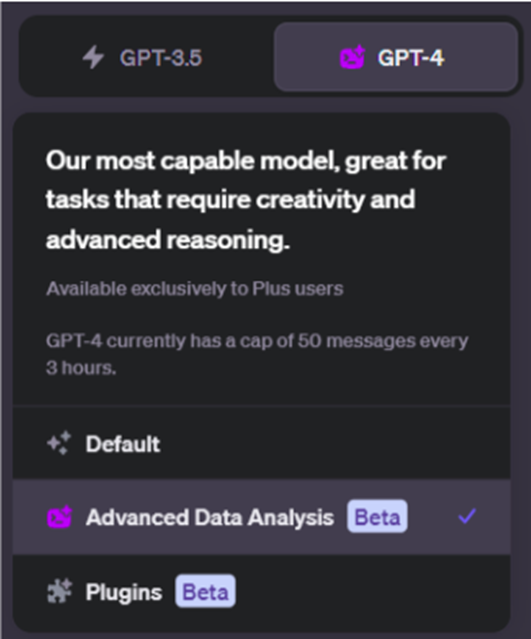
Evaluating Overspending with AI and Prototype Speed
When addressing overspending, AI prototypes the analysis remarkably fast. While Python or SQL could perform the same tasks, AI expedites the process significantly, enabling swift prototyping. However, it’s crucial to emphasize that all output requires thorough verification and review, given our responsibility for the accuracy of the results.
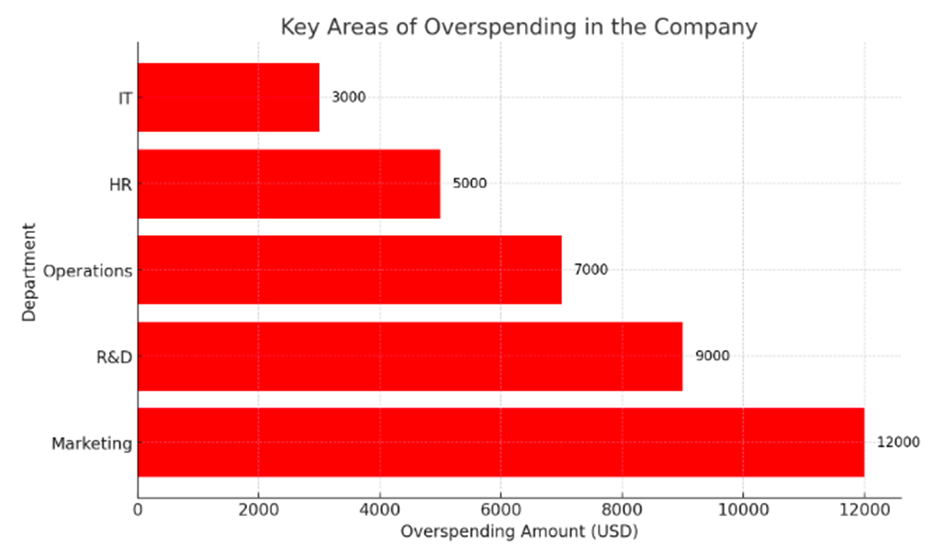
Analyzing ROI and Crafting Strategic Cuts with ChatGPT
Determining the Return on Investment (ROI) entails specific calculation methods. I instructed ChatGPT on the ROI calculations for different areas of expenditure. It revealed an interesting landscape. While certain sectors exhibited substantial overspending, they also delivered commendable ROI, suggesting efficiency despite the overspending. This calls for strategic evaluations to identify areas for potential cuts.
Generative AI and Visual Data Representation
AI-generated visuals, such as charts and graphs, play a significant role in facilitating quick exploratory data analysis. They offer a starting point for deeper strategic thinking. However, it’s crucial to assess if the chosen visual representation aligns with the precise data interpretation needs.
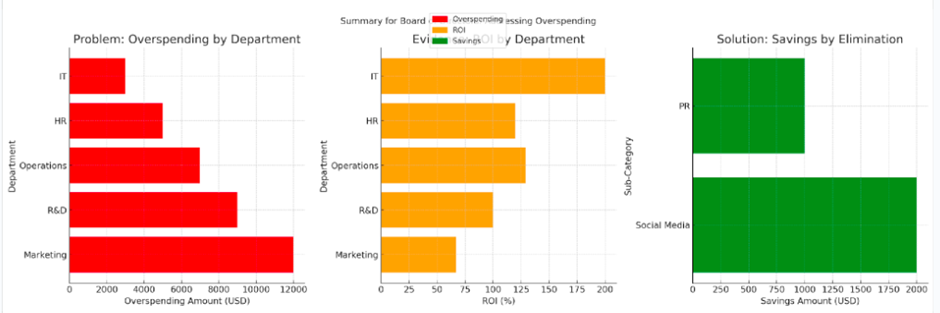
Privacy and Ethical Considerations in Leveraging AI
Generative AI possesses an incredible capacity to access diverse data sources, from online repositories to notebooks. The adaptability is quite remarkable—I’ve fed sizable datasets into AI without hitting any discernible limits. However, for sensitive information, particularly personally identifiable data, it’s imperative to steer clear of incorporating such content into the AI for privacy reasons.
The implementation of AI in daily professional data activities raises other ethical concerns too. AI-generated information can sometimes convincingly portray incorrect data, thus emphasizing our role in verifying and validating the output. Bias in AI systems is a well-documented concern, and it is our responsibility to ensure fair and unbiased analyses. It’s important to balance the power of AI with ethical considerations, particularly regarding data privacy and misinformation.

A pivotal aspect to remember is that while AI significantly enhances our analytical capabilities, the responsibility for accurate and ethical usage ultimately rests on us—the data professionals. AI acts as a tool, and we need to be vigilant in validating the information generated to maintain credibility. Being accountable for the outcomes, we should seek to harmonize AI’s efficacy with ethical and accurate decision-making.
As an experienced professional in data science, I’ve encountered various viewpoints regarding these concerns. It’s essential to consider these aspects while integrating AI into our daily workflow. This includes ethical implications, responsibility, and the potential consequences of using AI-generated content.
Conclusion
Generative AI is transforming data analysis by fostering innovation and redefining storytelling, propelling us into an exciting era of enhanced efficiency and ethical considerations. It amplifies analytical processes while emphasizing accountability and accuracy on our part. The journey of integrating Generative AI not only augments efficiency but also encompasses a spectrum of considerations to navigate for harnessing its potential, ensuring responsible and ethical usage.
This brief yet comprehensive overview emphasizes the broad scope and implications of integrating Generative AI into the realm of data analysis. It’s an exciting journey that not only augments our efficiency but also presents a spectrum of considerations we must address when harnessing its potential. I hope this serves as an enlightening guide, shedding light on how Generative AI can revolutionize your data analytics journey, providing a new perspective on optimizing your business efficiency and impact in the world of data analysis.
Key Takeaways:
- AI models like GPT-4 offer innovative solutions, aiding in data access, analysis, and prototype speed, shaping strategic decision-making, and facilitating complex evaluations.
- Uniting Generative AI and analytics for storytelling is essential. Crafting a narrative and presenting data through visuals are crucial to convey findings effectively to stakeholders.
- Verifying AI-generated information is crucial, ensuring ethical implications, responsibility, and accuracy in implementing AI for data analysis.
Frequently Asked Questions
A. Generative AI creates novel content, unlike traditional AI that predicts based on historical data. It synthesizes visuals, audio, and text, shaping storytelling and strategic decision-making.
A. Generative AI content may convincingly mimic incorrect data, emphasizing the need for rigorous verification. AI systems often carry biases, requiring vigilance to ensure fair and unbiased analyses.
A. No, while AI significantly enhances analytics, the responsibility for accurate usage rests on data professionals. AI serves as a tool, requiring validation to maintain credibility and ethical standards in analysis.
About the Author: Andrew Madson
Andrew Madson is the Senior Director of Data Analytics at Arizona State University and a seasoned university professor with over 18 years of experience. His profound expertise covers machine learning, AI governance, and strategic data analytics, having led data initiatives at multiple Fortune 500 companies. As a dedicated educator, Andrew has imparted his knowledge to thousands of graduate students in the fields of data science and data analytics.
DataHour Page: https://community.analyticsvidhya.com/c/datahour/advanced-generative-ai-and-data-storytelling
LinkedIn: https://www.linkedin.com/in/andrew-madson/
Related
- SEO Powered Content & PR Distribution. Get Amplified Today.
- PlatoData.Network Vertical Generative Ai. Empower Yourself. Access Here.
- PlatoAiStream. Web3 Intelligence. Knowledge Amplified. Access Here.
- PlatoESG. Carbon, CleanTech, Energy, Environment, Solar, Waste Management. Access Here.
- PlatoHealth. Biotech and Clinical Trials Intelligence. Access Here.
- Source: https://www.analyticsvidhya.com/blog/2023/11/unveiling-the-impact-of-generative-ai-in-data-storytelling-and-analytics/
- :has
- :is
- :not
- :where
- 11
- 12
- 15%
- 500
- a
- access
- accountability
- accountable
- accuracy
- accurate
- activities
- acts
- adaptability
- address
- addressing
- advanced
- adversarial
- AI
- AI governance
- AI models
- AI systems
- aids
- Aligns
- All
- Allowing
- also
- alternative
- amplifies
- an
- analyses
- analysis
- analyst
- Analysts
- Analytical
- analytics
- analyzing
- and
- Andrew
- any
- ARE
- areas
- arizona
- Arizona State University
- artificial
- artificial intelligence
- AS
- asked
- asking
- aspect
- aspects
- assess
- At
- audio
- augments
- Authentic
- author
- Balance
- based
- BE
- being
- between
- Beyond
- bias
- biases
- both
- broad
- business
- but
- by
- calculation
- calculations
- Calls
- CAN
- capabilities
- Capacity
- carry
- case
- case study
- certain
- Chance
- changing
- Charts
- ChatGPT
- checking
- choice
- chosen
- clarity
- Cleaning
- clear
- closely
- Collecting
- commendable
- commonly
- communicate
- Companies
- complex
- comprehensive
- Concern
- Concerns
- concise
- Connecting
- Consequences
- Consider
- considerations
- content
- contrast
- Costs
- could
- courses
- covered
- covers
- create
- creates
- Creating
- creation
- Credibility
- crucial
- crux
- cuts
- daily
- data
- data access
- data analysis
- Data Analytics
- data privacy
- data science
- datasets
- Decision Making
- dedicated
- deeper
- define
- defining
- delivered
- Despite
- determining
- developments
- differ
- different
- Director
- diverse
- does
- Doesn’t
- drives
- driving
- due
- during
- each
- Edge
- effectively
- efficacy
- efficiency
- element
- elements
- emphasize
- emphasizes
- emphasizing
- enabling
- encompasses
- enhanced
- Enhances
- ensure
- ensuring
- Enters
- entirely
- equally
- Era
- essential
- ethical
- ethics
- evaluating
- evaluations
- Examining
- exciting
- exemplifies
- exhibited
- expansive
- expedites
- experience
- experienced
- expertise
- Exploratory Data Analysis
- explore
- facilitate
- facilitating
- fact
- fair
- FAST
- Fed
- field
- Fields
- findings
- Focus
- focuses
- For
- Fortune
- fostering
- found
- from
- Fundamentals
- game
- GANs
- generated
- generating
- generative
- Generative AI
- generator
- given
- Goes
- governance
- graduate
- graphs
- guide
- had
- Harnessing
- having
- help
- helps
- here
- High
- his
- historical
- hitting
- hope
- How
- However
- HTTPS
- i
- ideas
- identify
- if
- Impact
- imperative
- implementation
- implementing
- implications
- important
- in
- includes
- Including
- incorporating
- incredible
- information
- initial
- initiatives
- Innovation
- innovative
- insights
- Integrating
- Intelligence
- interesting
- interpretation
- into
- investment
- involves
- IT
- ITS
- journey
- just
- knowledge
- landscape
- layoffs
- learning
- Led
- Led Data
- leveraging
- lies
- light
- like
- limits
- Llama
- logic
- machine
- machine learning
- maintain
- MAKES
- max-width
- May..
- methods
- might
- Misinformation
- models
- most
- multiple
- must
- my
- NARRATIVE
- Navigate
- Need
- needs
- networks
- Neural
- neural networks
- New
- next
- no
- notebooks
- novel
- objectives
- of
- offer
- offering
- often
- on
- ONE
- online
- only
- operational
- optimizing
- or
- Other
- Others
- our
- outcomes
- output
- over
- overview
- page
- part
- particularly
- partner
- perform
- Personally
- perspective
- phase
- pivotal
- plato
- Plato Data Intelligence
- PlatoData
- Play
- Point
- possesses
- possibilities
- potential
- power
- precise
- Precision
- predict
- predicting
- Predictions
- Predicts
- preparing
- presentation
- presents
- previous
- privacy
- process
- processes
- processing
- professional
- professionals
- Professor
- profound
- propelling
- prototype
- prototypes
- prototyping
- provides
- providing
- purpose
- Python
- Questions
- Quick
- quite
- raises
- rather
- real
- realm
- reasons
- recent
- Redefining
- reducing
- refine
- regarding
- remember
- representation
- represents
- Requirements
- requires
- responsibility
- responsible
- Results
- return
- Revealed
- review
- revolutionize
- Revolutionizing
- rigorous
- ROI
- Role
- roles
- same
- scene
- Science
- scope
- seasoned
- Sectors
- Seek
- seen
- senior
- sensitive
- Sequence
- serve
- serves
- session
- several
- shaping
- should
- significant
- significantly
- sizable
- solely
- solid
- Solutions
- something
- sometimes
- Sources
- spanning
- specific
- Spectrum
- speed
- SQL
- stakeholders
- standards
- Starting
- State
- steer
- Steps
- storytelling
- Strategic
- Strategically
- strategies
- Strategy
- strengths
- Students
- Study
- substantial
- such
- Suit
- SUMMARY
- SWIFT
- Systems
- Takeaways
- tasks
- techniques
- technological
- text
- that
- The
- the information
- the world
- their
- Them
- There.
- These
- they
- Thinking
- this
- thousands
- Through
- Thus
- time
- to
- today’s
- together
- too
- tool
- traditional
- Training
- trains
- transformers
- transforming
- trustworthiness
- two
- Ultimately
- understand
- understanding
- unique
- university
- unlike
- unlock
- unveiling
- us
- Usage
- use
- using
- validating
- validation
- various
- Verification
- verifying
- viewpoints
- vigilance
- visuals
- we
- Wealth
- webp
- WELL
- What
- when
- while
- with
- without
- workflow
- world
- years
- yet
- Your
- zephyrnet