Google DeepMind’s recent breakthrough with SIMA (Self-Instructing Multimodal Agent) shines a spotlight on the rapid progress in making generalist AI agents, specifically designed for 3D virtual environments, a reality.
This progress carries transformative potential, not just for the gaming industry, but for the way we interact with virtual spaces across a broad spectrum of applications.
With enhanced capabilities in understanding instructions, adapting to new tasks, and reasoning within the constraints of virtual worlds, SIMA-like agents offer the potential to reshape several key areas.
SIMA’s massive success
DeepMind’s latest innovation is SIMA, which stands for Scalable Instructable Multiworld Agent. Unlike previous AI focused on mastering a single game, SIMA is a generalist AI.
SIMA isn’t limited to pixels on the screen. It can process both visual information (what it sees in the game) and natural language instructions (what a human tells it to do). This multimodal learning allows for a more nuanced understanding of the game world.
A generalist AI agent strives to be a versatile tool within virtual environments. Unlike traditional AI focuses on a single task, a generalist AI aims to learn and perform a wide range of actions. This allows it to adapt to new situations and environments it hasn’t been specifically trained for.
SIMA isn’t trained on just one game. DeepMind collaborated with several game developers, exposing SIMA to a variety of titles like No Man’s Sky and Teardown. This diversity strengthens its ability to adapt to new environments.
SIMA doesn’t need to be spoon-fed every rule. By following instructions, it can learn new skills within a game, like navigating a new area, crafting an item, or using in-game menus. This makes it far more versatile than traditional AI agents.
Don’t be fooled by the lack of focus on achieving top scores. While impressive, that’s not the main objective.
SIMA’s true success lies in its ability to understand and act on human instructions within a game environment. This research signifies a HUGE step has been taken to create an AI that can be helpful to us in the real world.
Some of the games where Google DeepMind runs this groundbreaking AI model are:
- Goat Simulator 3
- Hydroneer
- No Man’s Sky
- Satisfactory
- Teardown
- Valheim
- Wobbly Life
Apart from all these games, the Google DeepMind team also tested SIMA’s capabilities in realistic simulations created by them called: “Research Environments“. These environments, consisting of Construction Lab, Playhouse, ProcTHOR, and WorldLab, simulate many areas where artificial intelligence is considered to be integrated in the near future.
The magic behind SIMA
Multimodal input processing
SIMA utilizes large language models (LLMs), likely based on the Transformer architecture, to process and understand natural language instructions given by a user. LLMs excel at handling sequential data like text, making them well-suited for this task. To make sense of its surroundings, SIMA employs convolutional neural networks (CNNs) to process visual input from the 3D environment.
CNNs are exceptionally good at extracting spatial features and patterns from images or video streams. SIMA likely uses multiple CNNs to create different levels of representation within the visual input for comprehensive understanding.
Self-instruction
One of the key innovations underlying SIMA is its ability to break down complex instructions into a sequence of simpler sub-tasks. This is likely achieved through a combination of natural language processing (to analyze the instructions) and hierarchical reinforcement learning (RL).
Hierarchical RL allows agents to learn complex behaviors by building upon sequences of lower-level actions.
Additionally, SIMA can generate its own training data and targets by observing its actions within the environment and the resulting changes. This self-supervision technique is crucial for enabling continuous learning and adaptation in new environments, giving it flexibility.
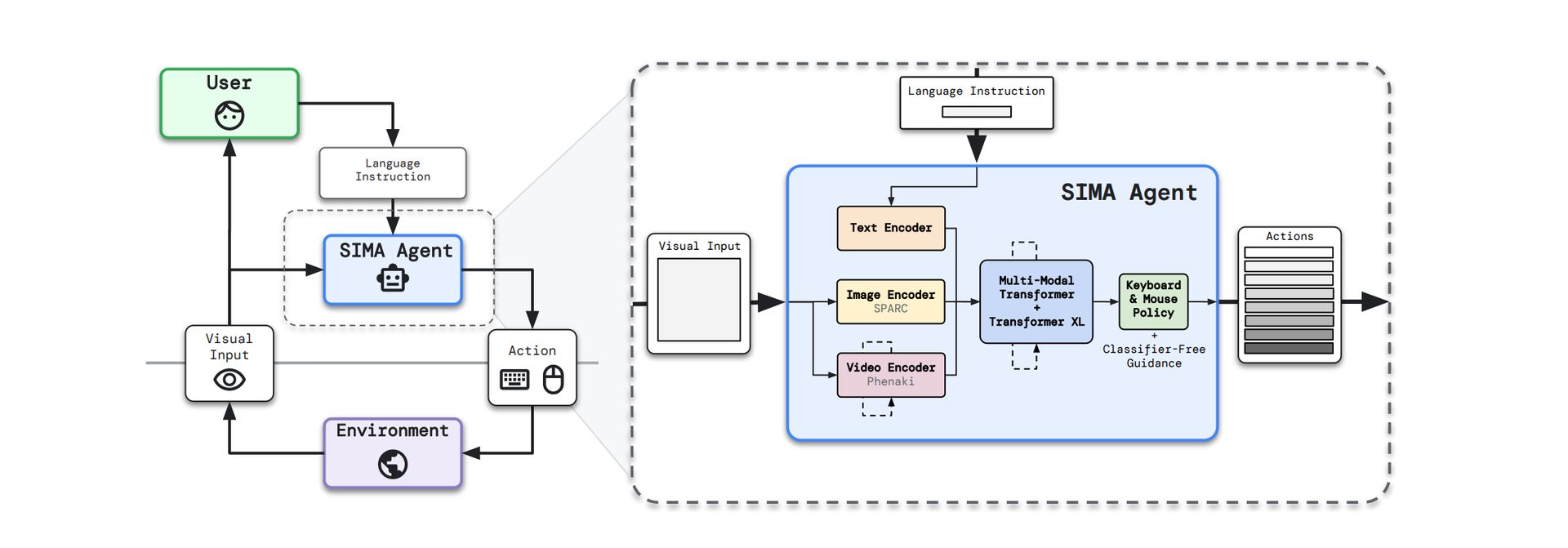
Zero-shot generalization
SIMA’s impressive ability to perform new tasks without explicit training likely stems from extensive pre-training on a massive dataset of diverse 3D environments and associated instructions. This pre-training allows the model to build a rich internal representation of virtual worlds and common instructions, enabling it to generalize knowledge.
It’s probable that a meta-learning approach is used during pre-training, encouraging SIMA to develop a strategy for “learning how to learn“.
This allows the agent to acquire new skills quickly within unseen environments.
You may learn more about Google DeepMind’s work on generalist AI agent training using games from their research paper.
Learn from games to shine in the real world
Believe it or not, SIMA marks a turning point in the development of AI.
Video games offer the ideal training ground for AI because they are dynamic, self-contained worlds with clear goals, rules, and feedback mechanisms.
Within these virtual spaces, AI agents can experiment, make mistakes, and learn from their successes and failures – all without the risks or limitations of the real world. As SIMA explores more intricate game worlds and its underlying models become more powerful, it develops the ability to adapt, understand instructions, and strategize to achieve goals.
These skills, honed in the safe sandbox of a game, translate into a versatile and capable AI that can potentially navigate the complexities of our real world.
This is just the beginning of what’s possible when AI learns through play.
Actually, the potential of AI to address real-world challenges becomes clear when we examine the prompts used by Google DeepMind in various games.
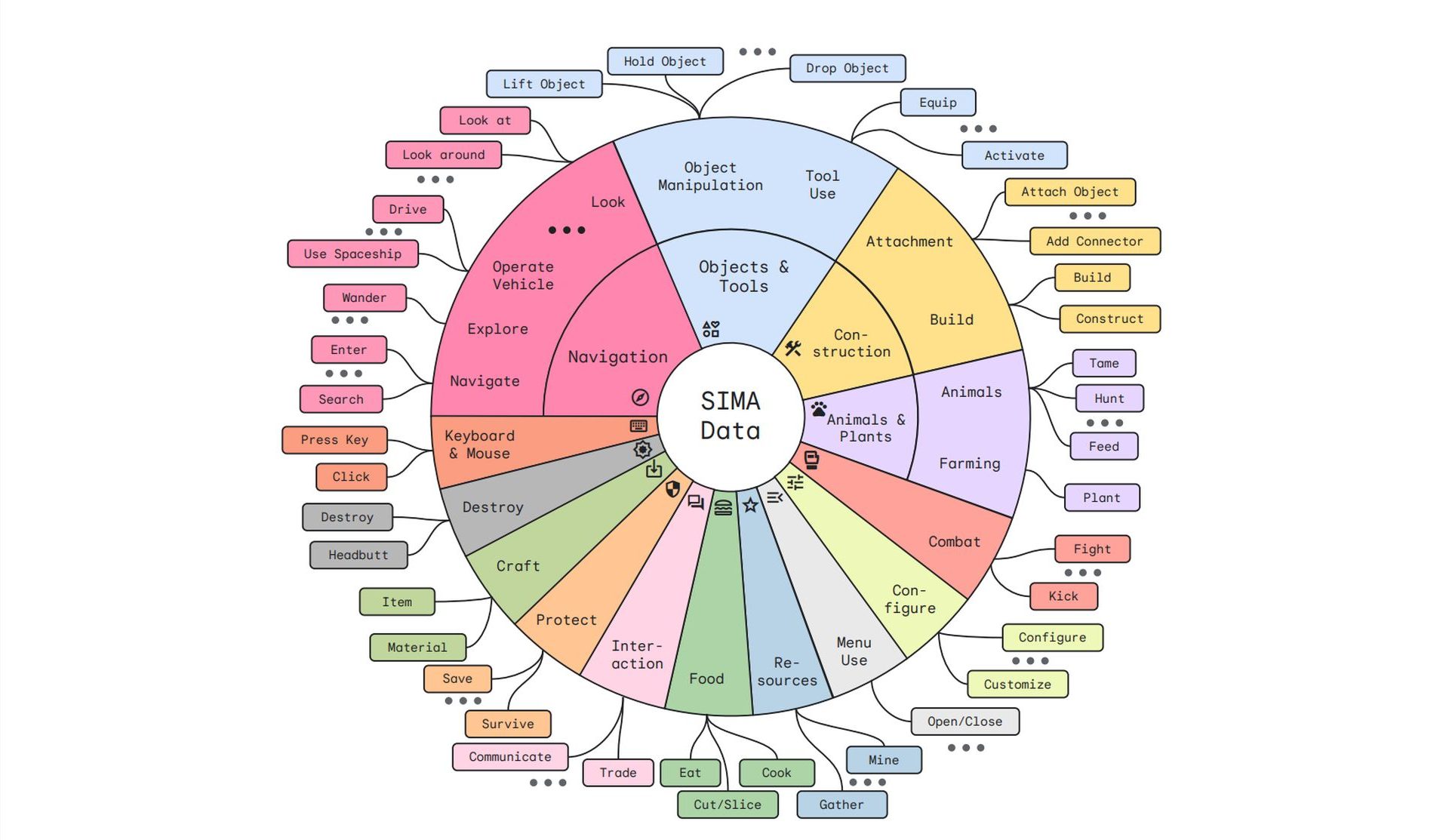
To give a few examples:
The “Pick up iron ore” prompt in Satisfactory hints at the potential for AI to improve safety in hazardous industries like mining. The Bureau of Labor Statistics reports a distressing rise in fatal mining injuries, with a 21.8% increase from 2020 to 2021. Imagine the lives that could be saved if AI-powered robots, less prone to human error or fatigue, were to handle dangerous mining tasks.
In the survival game Valheim, the “Find water” prompt highlights the power of AI in addressing vital issues like water scarcity. The World Bank reports that about 226 million people in Eastern and Southern Africa did not have access to basic water services, and 381 million people lacked access to basic sanitation services.
Another robot that can carry out water research on the natural water source in the region without any interruption can touch the lives of billions of people.
Although artificial intelligence seems to be identified with image generation and incessant chatbots nowadays, believe us, it is much more than that, and studies like these hold immense potential for a better future for all.
Featured image credit: Freepik.
- SEO Powered Content & PR Distribution. Get Amplified Today.
- PlatoData.Network Vertical Generative Ai. Empower Yourself. Access Here.
- PlatoAiStream. Web3 Intelligence. Knowledge Amplified. Access Here.
- PlatoESG. Carbon, CleanTech, Energy, Environment, Solar, Waste Management. Access Here.
- PlatoHealth. Biotech and Clinical Trials Intelligence. Access Here.
- Source: https://dataconomy.com/2024/03/13/google-deepmind-sima-generalist-ai-agent/
- :has
- :is
- :not
- :where
- $UP
- 1
- 2%
- 2020
- 3d
- a
- ability
- About
- access
- Achieve
- achieved
- achieving
- acquire
- across
- Act
- actions
- actually
- adapt
- adaptation
- adapting
- address
- addressing
- africa
- Agent
- agents
- AI
- AI-powered
- aims
- All
- allows
- also
- an
- analyze
- and
- any
- applications
- approach
- architecture
- ARE
- AREA
- areas
- artificial
- artificial intelligence
- AS
- associated
- At
- Bank
- based
- basic
- basis
- BE
- because
- become
- becomes
- been
- behaviors
- behind
- believe
- billions
- both
- Break
- breakthrough
- broad
- build
- Building
- Bureau
- bureau of labor statistics
- but
- by
- called
- CAN
- capabilities
- capable
- carry
- challenges
- Changes
- chatbots
- clear
- collaborated
- combination
- Common
- complex
- complexities
- comprehensive
- considered
- Consisting
- constraints
- construction
- continuous
- could
- crafting
- create
- created
- crucial
- Dangerous
- data
- DeepMind
- designed
- develop
- developers
- Development
- develops
- DID
- different
- diverse
- Diversity
- Doesn’t
- down
- during
- dynamic
- eastern
- employs
- enabling
- encouraging
- enhanced
- Environment
- environments
- error
- Ether (ETH)
- Every
- examples
- Excel
- exceptionally
- experiment
- explores
- extensive
- failures
- far
- fatigue
- Features
- feedback
- few
- Flexibility
- Focus
- focused
- focuses
- following
- For
- from
- future
- game
- Games
- gaming
- Gaming industry
- generate
- generation
- Give
- given
- Giving
- Goals
- good
- Ground
- groundbreaking
- handle
- Handling
- Have
- helpful
- High
- highlights
- hints
- hold
- How
- How To
- HTML
- HTTPS
- human
- Humanity
- identified
- if
- image
- image generation
- images
- imagine
- immense
- impressive
- improve
- in
- in-game
- Increase
- industries
- industry
- information
- Innovation
- innovations
- input
- instructions
- integrated
- integration
- Intelligence
- interact
- internal
- into
- intricate
- issues
- IT
- ITS
- jpg
- just
- just one
- Key
- Key Areas
- knowledge
- lab
- labor
- Lack
- language
- latest
- LEARN
- learning
- learns
- less
- levels
- lies
- like
- likely
- limitations
- Limited
- Lives
- machine
- machine learning
- magic
- Main
- make
- MAKES
- Making
- many
- massive
- Mastering
- max-width
- May..
- mechanisms
- million
- Mining
- model
- models
- more
- much
- multiple
- Natural
- Natural Language
- Navigate
- navigating
- Near
- Need
- networks
- Neural
- neural networks
- New
- no
- nowadays
- nuanced
- observing
- of
- offer
- on
- ONE
- or
- our
- out
- own
- patterns
- People
- perform
- performs
- plato
- Plato Data Intelligence
- PlatoData
- Play
- playing
- Point
- possible
- potential
- potentially
- power
- powerful
- previous
- process
- Progress
- prompts
- quickly
- range
- rapid
- real
- real world
- realistic
- Reality
- reasoning
- recent
- region
- reinforcement learning
- representation
- research
- reshape
- resulting
- Rich
- Rise
- robot
- robots
- Rule
- rules
- runs
- safe
- Safety
- sandbox
- Save
- saved
- scalable
- Scarcity
- scores
- Screen
- seems
- sees
- sense
- Sequence
- Services
- several
- shine
- shines
- signifies
- simpler
- simulate
- simulations
- simulator
- single
- situations
- skills
- sky
- Source
- Southern
- spaces
- Spatial
- specifically
- Spectrum
- Spotlight
- stands
- statistics
- stems
- Step
- strategize
- Strategy
- streams
- Strengthens
- strives
- studies
- success
- successes
- survival
- survival game
- taken
- targets
- Task
- tasks
- team
- teardown
- technique
- techniques
- Technologies
- tells
- tested
- text
- than
- that
- The
- their
- Them
- These
- they
- this
- Through
- titles
- to
- tool
- top
- touch
- traditional
- trained
- Training
- transformative
- transformer
- translate
- true
- underlying
- understand
- understanding
- unlike
- upon
- us
- used
- User
- uses
- using
- utilizes
- variety
- various
- versatile
- Video
- video games
- Virtual
- virtual worlds
- visual
- vital
- Water
- Way..
- we
- were
- when
- which
- while
- wide
- Wide range
- Wikipedia
- with
- within
- without
- Work
- world
- World Bank
- world’s
- zephyrnet