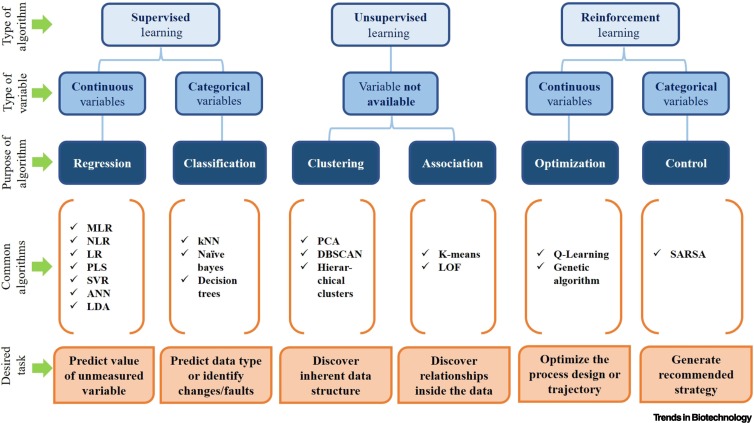
Introduction to Artificial Intelligence.
Pearson Education India, 1985
Artificial Intelligence: A Philosophical Introduction.
John Wiley & Sons, 2015
Machine learning: trends, perspectives, and prospects.
Science. 2015; 349: 255-260
Artificial intelligence in healthcare market by offering (hardware, software, services), technology (machine learning, NLP, context-aware computing, end-use application, end user, and geography: Global forecast to 2025.
() MarketsandMarkets, 2018
Artificial intelligence in drug development: present status and future prospects.
Drug Discov. Today. 2019; 24: 773-780
How artificial intelligence is changing drug discovery.
Nature. 2018; 557: S55-S57
In silico clinical trials: how computer simulation will transform the biomedical industry.
Int. J. Clin. Trials. 2016; 3: 37-46
An AI boost for clinical trials.
Nature. 2019; 573: S100-S102
Artificial intelligence for clinical trial design.
Trends Pharmacol. Sci. 2019; 40: 577-591
Artificial Intelligence in pharmaceuticals, biologics, and medical devices: present and future regulatory models.
Fordham L. Rev. 2019; 88: 553
Artificial intelligence and arthroplasty at a single institution: real-world applications of machine learning to big data, value-based care, mobile health, and remote patient monitoring.
J. Arthroplast. 2019; 34: 2204-2209
The move toward biopharma 4.0: in silico biotechnology develops “smart” processes that benefit biomanufacturing through Digital Twins.
Genet. Eng. Biotechnol. News. 2019; 39: 53-55
Applications of artificial intelligence in intelligent manufacturing: a review.
Front. Inf. Technol. Electron. Eng. 2017; 18: 86-96
Intelligent biopharma – forging the links across the value chain, Deloitte Insights.
2019 ()
Key cost drivers of pharmaceutical clinical trials in the United States.
Clin. Trials. 2016; 13: 117-126
Machine learning in manufacturing: advantages, challenges, and applications.
Prod. Manufact. Res. 2016; 4: 23-45
PAT — A Framework for Innovative Pharmaceutical Development, Manufacturing, and Quality Assurance.
Hybrid Models for the simulation and prediction of chromatographic processes for protein capture.
J. Chromatogr. A. 2021; 1650462248https://doi.org/10.1016/j.chroma.2021.462248
Hybrid modeling for quality by design and PAT-benefits and challenges of applications in biopharmaceutical industry.
Biotechnol. J. 2014; 9: 719-726
Hybrid modeling and intensified DoE: an approach to accelerate upstream process characterization.
Biotechnol. J. 2020; 15: 2000121
Digital twin application for model-based DoE to rapidly identify ideal process conditions for space-time yield optimization.
Processes. 2021; 9: 1109
Hybrid modeling for simultaneous prediction of flux, rejection factor and concentration in two-component crossflow ultrafiltration.
Processes. 2020; 8: 1625
Process analytical technology (PAT) for biopharmaceuticals.
Biotechnol. J. 2011; 6: 369
Guidance for performing multivariate data analysis of bioprocessing data: pitfalls and recommendations.
Biotechnol. Prog. 2014; 30: 967-973
Towards a digital bioprocess replica: computational approaches in biopharmaceutical development and manufacturing.
Trends Biotechnol. 2020; 38: 1141-1153
Green simulation assisted reinforcement learning with model risk for biomanufacturing learning and control.
2006 ()
Reinforcement learning based optimization of process chromatography for continuous processing of biopharmaceuticals.
Chem. Eng. Sci. 2021; 230116171
Digital Twins: A General Overview of the Biopharma Industry.
Adv. Biochem. Eng. Biotechnol. 2021; 2021: 167-184
Neural Networks In Bioprocessing And Chemical Engineering.
Academic Press, 1995
Pattern Recognition and Machine Learning.
Springer, 2006
Artificial Intelligence: A Modern Approach.
Pearson, 2010
Evolving artificial neural networks.
Proc. IEEE. 1999; 87: 1423-1447
Principles of Artificial Neural Networks.
Vol. 7. World Scientific, 2013
Artificial Neural Networks: Learning Algorithms, Performance Evaluation, and Applications.
Vol. 209. Springer Science & Business Media, 1992
Reinforcement learning: a survey.
J. Artif. Intell. Res. 1996; 4: 237-285
Mastering the game of go without human knowledge.
Nature. 2017; 550: 354-359
Deep Reinforcement Learning Hands-On: Apply Modern RL Methods, with Deep Q-networks, Value Iteration, Policy Gradients, TRPO, AlphaGo Zero and More.
Packt Publishing Ltd, 2018
Efficient Exploration in Reinforcement Learning. Technical Report.
Carnegie Mellon University, 1992
Engineering challenges in high density cell culture systems.
Cytotechnology. 1996; 22: 3-16
Online prediction of product titer and solubility of recombinant proteins in Escherichia coli fed-batch cultivations.
J. Chem. Technol. Biotechnol. 2015; 90: 283-290
The potential of random forest and neural networks for biomass and recombinant protein modeling in Escherichia coli fed-batch fermentations.
Biotechnol. J. 2015; 10: 1770-1782
Modeling of bioprocess non-linear fluorescence data for at-line prediction of etanercept based on artificial neural networks optimized by response surface methodology.
Talanta. 2020; 210120664
Artificial neural network associated to UV/Vis spectroscopy for monitoring bioreactions in biopharmaceutical processes.
Bioprocess Biosyst. Eng. 2015; 38: 1045-1054
Accurate and cost-effective prediction of HBsAg titer in industrial scale fermentation process of recombinant Pichia pastoris by using neural network based soft sensor.
Biotechnol. Appl. Biochem. 2019; 66: 681-689
Development of a fuzzy system for dissolved oxygen control in a recombinant Escherichia coli cultivation for heterologous protein expression.
Comput. Aided Chem. Eng. 2018; 43: 1129-1134
Comparative study of m-stat methanol feeding control in fed-batch fermentation of Pichia pastoris producing HBsAg: an open-loop control versus recurrent artificial neural network-based feedback control.
J. Chem. Technol. Biotechnol. 2019; 94: 3924-3931
Online deep neural network-based feedback control of a lutein bioprocess.
J. Process Control. 2021; 98: 41-51
Hybrid modeling as a QbD/PAT tool in process development: an industrial E. coli case study.
Bioprocess Biosyst. Eng. 2016; 39: 773-784
Artificial neural network for bioprocess monitoring based on fluorescence measurements: training without offline measurements.
Eng. Life Sci. 2017; 17: 874-880
The rise of deep learning in drug discovery.
Drug Discov. Today. 2018; 23: 1241-1250
Deep reinforcement learning for de novo drug design.
Sci. Adv. 2018; 4eaap7885
Optimization of molecules via deep reinforcement learning.
Sci. Rep. 2019; 9: 1-10
Deep reinforcement learning for the control of microbial co-cultures in bioreactors.
PLoS Comput. Biol. 2020; 16e1007783
Reinforcement learning under model risk for biomanufacturing fermentation control.
2021 ()
Continuous integrated manufacturing of therapeutic proteins.
Curr. Opin. Biotechnol. 2018; 53: 76-84
Model-based methods in the biopharmaceutical process lifecycle.
Pharm. Res. 2017; 34: 2596-2613
Soft sensors in bioprocessing: a status report and recommendations.
7. 2012: 1040-1048
Modelling continuous pharmaceutical and bio-based processes at plant-wide level: a roadmap towards efficient decision-making.
Chem. Today. 2018; 36: 26-30
Microbial technologies for biotherapeutics production: key tools for advanced biopharmaceutical process development and control.
Drug Discov. Today Technol. 2020; 38: 9-24
Root cause investigation of deviations in protein chromatography based on mechanistic models and artificial neural networks.
J. Chromatogr. A. 2017; 1515: 146-153
D.Q. Lin, et al. (2019) Design and analysis of multi-column continuous chromatography based on artificial neural network. China Patent CN110348090A
Artificial neural network (ANN)-based prediction of depth filter loading capacity for filter sizing.
Biotechnol. Prog. 2016; 32: 1436-1443
Hybrid modeling of cross-flow filtration: predicting the flux evolution and duration of ultrafiltration processes.
Sep. Purif. Technol. 2020; 248117064
Hybrid modeling for simultaneous prediction of flux, rejection factor and concentration in two-component crossflow ultrafiltration.
Processes. 2020; 8: 1625
Preliminary modeling of an industrial recombinant human erythropoietin purification process by artificial neural networks.
Braz. J. Chem. Eng. 2015; 32: 725-734
Optimization of biopharmaceutical downstream processes supported by mechanistic models and artificial neural networks.
Biotechnol. Prog. 2017; 33: 696-707
Model-based optimization of integrated purification sequences for biopharmaceuticals.
Chem. Eng. Sci. X. 2019; 3100025
Neural network to understand process capability and process intermediates acceptance criteria in monoclonal antibody production process.
J. Pharm. Innov. 2018; 13: 36-47
Improving biopharmaceutical manufacturing yield using neural network classification.
Bioprocess. J. 2016; 14: 39-50
Design of control framework based on deep reinforcement learning and Monte-Carlo sampling in downstream separation.
Comput. Chem. Eng. 2020; 140106910
Design of smart liquid-liquid extraction columns for downstream separations of biopharmaceuticals using deep Q-learning algorithm.
in: Computer Aided Chemical Engineering. Elsevier, 2019: 271-276
An introduction to machine learning.
Clin. Pharmacol. Ther. 2020; 107: 871-885
Reinforcement Learning: An Introduction.
MIT Press, 2018
Introduction to Machine Learning.
Vol. 32. Cambridge University, 2008: 2008
Introduction to Machine Learning.
MIT press, 2020
Neural Networks With R: Smart Models Using CNN, RNN, Deep Learning, and Artificial Intelligence Principles.
Packt Publishing Ltd, 2017: 2017
MATLAB Machine Learning.
Apress, 2016: 2016
Bioreactor scale-up and oxygen transfer rate in microbial processes: an overview.
Biotechnol. Adv. 2009; 27: 153-176
Rapid Growth in Biopharma: Challenges and Opportunities.
McKinsey & Company, 2014
Quality by design for biopharmaceuticals.
Nat. Biotechnol. 2009; 27: 26-34
Continuous Processing in Pharmaceutical Manufacturing.
John Wiley & Sons, 2014
Applied advanced process analytics in biopharmaceutical manufacturing: challenges and prospects in real-time monitoring and control.
J. Process Control. 2010; 20: 1009-1018
Control of surge tanks for continuous manufacturing of monoclonal antibodies.
Biotechnol. Bioeng. 2021; 118: 1913-1931
Data-driven anomaly detection and diagnostics for changeover processes in biopharmaceutical drug product manufacturing.
Chem. Eng. Res. Des. 2021; 167: 53-62
Comparison of data science workflows for root cause analysis of bioprocesses.
Bioprocess Biosyst. Eng. 2018; 42: 245-256
Process analytical technology implementation for protein refolding: GCSF as a case study.
Biotechnol. Bioeng. 2019; 116: 1039-1052
Angewandte Statistik. Methodensammlung mit R.
Springer, 2006
Another look at measures of forecast accuracy.
Int. J. Forecast. 2006; 22: 679-688
Basic principles of ROC analysis.
Semin. Nucl. Med. 1978; 8: 283-298
Advanced Data Mining Techniques.
Springer Publishing, 2008
Guidance for Industry, Q2B Validation of Analytical Procedures: Methodology; FDA-1996-D-0169.
Center for Drug Evaluation and Research, 1997
Common mistakes in cross-validating classification models.
Anal. Methods. 2017; 9: 4410-4417
Assessing equivalence of two assays using sensitivity and specificity.
J. Biopharm. Stat. 2007; 17: 433-443
Chemometrics in analytical chemistry—Part II: modeling, validation, and applications.
Anal. Bioanal. Chem. 2018; 410: 6691-6704
(2015) Validation of chemometric models—a tutorial.
Anal. Chim. Acta. 2015; 893: 14-24
ICH Q2 (R1) Validation of Analytical Procedures: Text and Methodology.
ICH, 1997
Evaluation of intermediate precision in the validation of analytical procedures for drugs: from NDA dossiers.
Pharm. Tech. Jpn. 2002; 18: 695-704
Nonparametric maximum likelihood estimation of a mixing distribution.
J. Am. Stat. Assoc. 1978; 73: 805-811
The Akaike information criterion: background, derivation, properties, application, interpretation, and refinements.
WIREs Comput. Stat. 2019; 11e1460
Goodness-of-Fit. Testing.
in: Peterson P. Baker E. McGaw B. International Encyclopedia of Education. 3rd ed. Elsevier, 2010: 190-196
(2017) Bioprocess optimization under uncertainty using ensemble modeling.
J. Biotechnol. 2017; 244: 34-44
In silico trials: verification, validation and uncertainty quantification of predictive models used in the regulatory evaluation of biomedical products.
Methods. 2021; 185: 120-127
Verification and validation of computational models used in biopharmaceutical manufacturing: potential application of the ASME verification and validation 40 standard and FDA proposed AI/ML model. Life cycle management framework.
J. Pharm. Sci. 2021; 110: 1540-1544
Validation is not verification: precise terminology and scientific methods in bioprocess modeling.
Trends Biotechnol. 2021; 39: 1117-1119
Aleatory or epistemic? Does it matter?.
Struct. Saf. 2009; 31: 105-112
A review of uncertainty quantification in deep learning: Techniques, applications and challenges.
Inform. Fusion. 2021; 76: 243-297
A method for handling metabonomics data from liquid chromatography/mass spectrometry: combinational use of support vector machine recursive feature elimination, genetic algorithm and random forest for feature selection.
Metabolomics. 2011; 7: 549-558
Application of a kNN-based similarity method to biopharmaceutical manufacturing.
Biotechnol. Prog. 2020; 36e2945
Linear discriminant analysis, partial least squares discriminant analysis, and soft independent modeling of class analogy of experimental and simulated near-infrared spectra of a cultivation medium for mammalian cells.
J. Chemom. 2018; 32e3005
Data mining algorithm for pre-processing biopharmaceutical drug product manufacturing records.
Comput. Chem. Eng. 2019; 124: 253-269
Decision Tree-PLS (DT-PLS) algorithm for the development of process: specific local prediction models.
Biotechnol. Prog. 2019; 35e2818
Advancing biopharmaceutical process development by system-level data analysis and integration of omics data.
in: Hu W. Zeng A.P. Genomics and Systems Biology of Mammalian Cell Culture. vol 127. Springer, Berlin, Heidelberg2011
Genetic programming assisted stochastic optimization strategies for optimization of glucose to gluconic acid fermentation.
Biotechnol. Prog. 2002; 18: 1356-1365
Biovalorisation of crude glycerol and xylose into xylitol by oleaginous yeast Yarrowia lipolytica.
Microb. Cell Factories. 2020; 19: 121
- SEO Powered Content & PR Distribution. Get Amplified Today.
- Platoblockchain. Web3 Metaverse Intelligence. Knowledge Amplified. Access Here.
- Source: https://www.cell.com/trends/biotechnology/fulltext/S0167-7799(22)00225-6?rss=yes
- 1
- 2017
- 2019
- 2021
- 7
- a
- accelerate
- acceptance
- accuracy
- across
- advanced
- advantages
- AI
- AI/ML
- algorithm
- algorithms
- analysis
- Analytical
- analytics
- and
- anomaly detection
- Antibodies
- Application
- applications
- Apply
- approach
- approaches
- artificial
- artificial intelligence
- Artificial Intelligence and Machine Learning
- artificial neural networks
- associated
- assurance
- authors
- background
- based
- benefit
- Big
- Big Data
- biology
- biomanufacturing
- biomass
- biomedical
- biopharma
- biotechnology
- boost
- business
- Capacity
- capture
- care
- case
- case study
- Cause
- Cells
- chain
- challenges
- changing
- chemical
- China
- Christopher
- class
- classification
- Clinical
- clinical trials
- CNN
- Columns
- computer
- computing
- concentration
- conditions
- continuous
- control
- Cost
- cost-effective
- criteria
- crude
- cultivation
- Culture
- Daniel
- data
- data analysis
- data mining
- data science
- David
- Decision Making
- deep
- deep learning
- deloitte
- depth
- Design
- Detection
- Development
- develops
- Devices
- digital
- Digital twins
- discovery
- distribution
- DOE
- drivers
- drug
- drug development
- drug discovery
- Drugs
- E. coli
- ed
- Education
- efficient
- Engineering
- Ether (ETH)
- evaluation
- evolution
- exploration
- extraction
- factories
- fda
- Feature
- feedback
- feeding
- filter
- FLUX
- Forecast
- forest
- Framework
- from
- fusion
- future
- game
- General
- geography
- Global
- Go
- gradients
- Growth
- Handling
- hands-on
- Hardware
- Health
- healthcare
- High
- How
- HTTPS
- human
- ideal
- identify
- IEEE
- implementation
- in
- independent
- industrial
- industry
- information
- innovative
- insights
- Institution
- integrated
- integration
- Intelligence
- Intelligent
- Intermediate
- interpretation
- Introduction
- investigation
- IT
- iteration
- jack
- Key
- knowledge
- learning
- Level
- Life
- links
- Liquid
- List
- loading
- local
- Look
- machine
- machine learning
- management
- manufacturing
- Marco
- Market
- Matter
- maximum
- measurements
- measures
- medical
- medical devices
- medium
- Mellon
- Methanol
- method
- Methodology
- methods
- Michael
- Mining
- mistakes
- MIT
- Mixing
- Mobile
- model
- modeling
- models
- Modern
- monitoring
- more
- move
- network
- network-based
- networks
- Neural
- neural network
- neural networks
- news
- nlp
- offering
- offline
- opportunities
- optimization
- optimized
- overview
- Oxygen
- Pack
- patent
- patient
- patient monitoring
- Paul
- performance
- performing
- perspectives
- Peter
- Pharmaceutical
- pharmaceuticals
- plato
- Plato Data Intelligence
- PlatoData
- policy
- potential
- Precision
- predicting
- prediction
- present
- principles
- procedures
- process
- processes
- processing
- Product
- Production
- Products
- Programming
- properties
- proposed
- prospects
- Protein
- Proteins
- Publishing
- Q2
- quality
- Ralf
- random
- rapidly
- Rate
- real world
- real-time
- recognition
- recommendations
- records
- Recursive
- regulatory
- reinforcement learning
- remote
- replica
- report
- response
- review
- Richard
- Rise
- Risk
- roadmap
- root
- Scale
- scale-up
- SCI
- Science
- selection
- Sensitivity
- sensors
- Services
- simulation
- simultaneous
- single
- smart
- Soft
- Software
- specific
- specificity
- Spectroscopy
- squares
- standard
- States
- Status
- strategies
- Study
- support
- Supported
- Surface
- surge
- Survey
- system
- Systems
- Tanks
- tech
- Technical
- techniques
- Technologies
- Technology
- terminology
- Testing
- The
- Therapeutic
- Through
- to
- today
- tool
- tools
- toward
- towards
- Training
- transfer
- Transform
- Trends
- trial
- trials
- tutorial
- Twins
- Uncertainty
- under
- understand
- United
- United States
- use
- User
- validation
- value
- value-based
- Verification
- Versus
- via
- W
- will
- without
- workflows
- X
- Yield
- zephyrnet
- zero