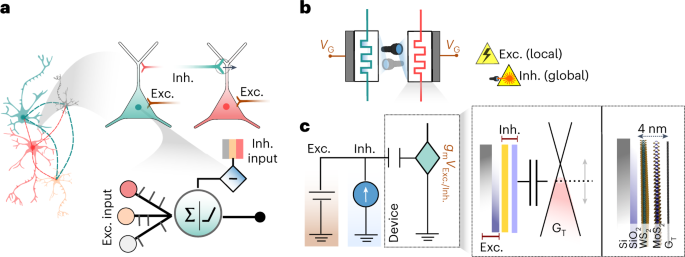
Merolla, P. A. et al. A million spiking-neuron integrated circuit with a scalable communication network and interface. Science 345, 668–673 (2014).
M, D., N, S., T, L. & G, C. Loihi: a neuromorphic manycore processor with on-chip learning. IEEE Micro 38, 82–99 (2018).
Tuma, T., Pantazi, A., Le Gallo, M., Sebastian, A. & Eleftheriou, E. Stochastic phase-change neurons. Nat. Nanotechnol. 11, 693–699 (2016).
Feldmann, J., Youngblood, N., Wright, C. D., Bhaskaran, H. & Pernice, W. H. All-optical spiking neurosynaptic networks with self-learning capabilities. Nature 569, 208–214 (2019).
Kumar, S., Williams, R. S. & Wang, Z. Third-order nanocircuit elements for neuromorphic engineering. Nature 585, 518–523 (2020).
Yi, W. et al. Biological plausibility and stochasticity in scalable VO2 active memristor neurons. Nat. Commun. 9, 4661 (2018).
Hassan, N. et al. Magnetic domain wall neuron with lateral inhibition. J. Appl. Phys. 124, 152127 (2018).
Kandel, E. R. et al. Principles of Neural Science Vol. 4 (McGraw-Hill, 2000).
Yin, H. in Computational Intelligence: a Compendium (eds Fulcher, J. & Jain, L. C.) 715–762 (Springer, 2008).
Maass, W. On the computational power of winner-take-all. Neural Comput. 12, 2519–2535 (2000).
Maass, W. Neural computation with winner-take-all as the only nonlinear operation. Adv. Neural Inf. Process. Syst. 12, 293–299 (2000).
Lazzaro, J., Ryckebusch, S., Mahowald, M. A. & Mead, C. A. Winner-take-all networks of O(n) complexity. Adv. Neural Inf. Process. Syst. 1, 703–711 (1988).
Kaski, S. & Kohonen, T. Winner-take-all networks for physiological models of competitive learning. Neural Netw. 7, 973–984 (1994).
Gerstner, W., Lehmann, M., Liakoni, V., Corneil, D. & Brea, J. Eligibility traces and plasticity on behavioral time scales: experimental support of neohebbian three-factor learning rules. Front. Neural Circuits 12, 53 (2018).
Ferguson, K. A. & Cardin, J. A. Mechanisms underlying gain modulation in the cortex. Nat. Rev. Neurosci. 21, 80–92 (2020).
Kreiser, R., Moraitis, T., Sandamirskaya, Y. & Indiveri, G. On-chip unsupervised learning in winner-take-all networks of spiking neurons. In 2017 IEEE Biomedical Circuits and Systems Conference (BioCAS) 1–4 (IEEE, 2017).
Hsu, D., Figueroa, M. & Diorio, C. Competitive learning with floating-gate circuits. IEEE Trans. Neural Netw. 13, 732–744 (2002).
Diehl, P. U. & Cook, M. Unsupervised learning of digit recognition using spike-timing-dependent plasticity. Front. Comput. Neurosci. 9, 99 (2015).
Ebong, I. E. & Mazumder, P. CMOS and memristor-based neural network design for position detection. Proc. IEEE 100, 2050–2060 (2011).
Srivastava, R. K., Masci, J., Kazerounian, S., Gomez, F. J. & Schmidhuber, J. Compete to compute. In Advances in Neural Information Processing Systems 26 (NIPS 2013), 2310–2318 (Citeseer, 2013).
Oster, M., Douglas, R. & Liu, S.-C. Computation with spikes in a winner-take-all network. Neural Comput. 21, 2437–2465 (2009).
Fenno, L., Yizhar, O. & Deisseroth, K. The development and application of optogenetics. Ann. Rev. Neurosci. 34, 389–412 (2011).
Gradinaru, V. et al. Molecular and cellular approaches for diversifying and extending optogenetics. Cell 141, 154–165 (2010).
Sarwat, S. G., Moraitis, T., Wright, C. D. & Bhaskaran, H. Chalcogenide optomemristors for multi-factor neuromorphic computation. Nat. Commun. 13, 2247 (2022).
Wang, Q. et al. Nonvolatile infrared memory in MoS2/PbS van der Waals heterostructures. Sci. Adv. 4, eaap7916 (2018).
Xiang, D. et al. Two-dimensional multibit optoelectronic memory with broadband spectrum distinction. Natu. Commun. 9, 2966 (2018).
Tran, M. D. et al. Two-terminal multibit optical memory via van der Waals heterostructure. Adv. Mater. 31, 1807075 (2019).
Lee, J. et al. Monolayer optical memory cells based on artificial trap-mediated charge storage and release. Nat. Commun. 8, 14734 (2017).
Sze, S. M., Li, Y. & Ng, K. K. Physics of Semiconductor Devices (John Wiley & Sons, 2021).
Amit, I. et al. Role of charge traps in the performance of atomically thin transistors. Adv. Mater. 29, 1605598 (2017).
Kim, S. Y., Yang, H. I. & Choi, W. Photoluminescence quenching in monolayer transition metal dichalcogenides by Al2O3 encapsulation. Appl. Phys. Lett. 113, 133104 (2018).
Li, Z., Wang, W., Greenham, N. C. & McNeill, C. R. Influence of nanoparticle shape on charge transport and recombination in polymer/nanocrystal solar cells. Phys. Chem. Chem. Phys. 16, 25684–25693 (2014).
Carpenter, G. A. & Grossberg, S. A massively parallel architecture for a self-organizing neural pattern recognition machine. Comput. Vis. Graph. Image Process. 37, 54–115 (1987).
Kohonen, T. Essentials of the self-organizing map. Neural Netw. 37, 52–65 (2013).
Kohonen, T. The self-organizing map. Proc. IEEE 78, 1464–1480 (1990).
Oh, S. et al. Energy-efficient Mott activation neuron for full-hardware implementation of neural networks. Nat. Nanotechnol. 16, 680–687 (2021).
Meng, W. et al. Three-dimensional monolithic micro-led display driven by atomically thin transistor matrix. Nat. Nanotechnol. 16, 1231–1236 (2021).
Hwangbo, S., Hu, L., Hoang, A. T., Choi, J. Y. & Ahn, J.-H. Wafer-scale monolithic integration of full-colour micro-led display using MoS2 transistor. Nat. Nanotechnol. 17, 500–506 (2022).
- SEO Powered Content & PR Distribution. Get Amplified Today.
- PlatoAiStream. Web3 Data Intelligence. Knowledge Amplified. Access Here.
- Minting the Future w Adryenn Ashley. Access Here.
- Buy and Sell Shares in PRE-IPO Companies with PREIPO®. Access Here.
- Source: https://www.nature.com/articles/s41565-023-01391-6
- ][p
- 1
- 10
- 11
- 12
- 13
- 14
- 15%
- 1994
- 20
- 2011
- 2012
- 2014
- 2015
- 2016
- 2017
- 2018
- 2019
- 2020
- 2021
- 2022
- 22
- 23
- 24
- 26
- 27
- 28
- 30
- 7
- 70
- 8
- 9
- a
- Activation
- active
- AL
- and
- Application
- approaches
- architecture
- article
- artificial
- AS
- based
- biomedical
- broadband
- by
- capabilities
- Cells
- charge
- click
- Communication
- compete
- competitive
- complexity
- computation
- computational power
- Compute
- Conference
- Design
- Detection
- Development
- Digit
- Display
- domain
- driven
- e
- E&T
- elements
- eligibility
- Engineering
- essentials
- Ether (ETH)
- extending
- feedback
- For
- Gain
- Gomez
- graph
- http
- HTTPS
- i
- IEEE
- image
- implementation
- in
- influence
- information
- integrated
- integration
- Intelligence
- Interface
- John
- learning
- LINK
- machine
- map
- massively
- Matrix
- mechanisms
- Memory
- metal
- million
- models
- molecular
- Monolithic
- Nature
- network
- networks
- Neural
- neural network
- neural networks
- Neurons
- of
- on
- only
- operation
- optical
- Parallel
- Pattern
- performance
- plato
- Plato Data Intelligence
- PlatoData
- position
- power
- process
- processing
- Processor
- recognition
- release
- Role
- rules
- s
- scalable
- scales
- semiconductor
- Shape
- solar
- Solar cells
- Spectrum
- spikes
- storage
- support
- Systems
- The
- three-dimensional
- time
- to
- transition
- transport
- traps
- underlying
- unsupervised learning
- using
- via
- W
- Wall
- Williams
- with
- Wright
- zephyrnet